Combination of inflammatory and vascular markers in the febrile phase of dengue is associated with more severe outcomes
- NguyenLamVuong[email protected]12
- PhungKhanhLam12
- DamienKengYenMing3
- HuynhThiLeDuyen1
- NguyetMinhNguyen1
- DongThiHoaiTam1
- KienDuongThiHue1
- NguyenVVChau4
- NgounChanpheaktra5
- LucyChaiSeeLum6
- ErnestoPleités7
- CameronPSimmons89
- KerstinDRosenberger10
- ThomasJaenisch1011
- DavidBell12
- NathalieAcestor13
- ChristineHalleux14
- PieroLOlliaro8
- BridgetAWills18
- RonaldBGeskus18
- SophieYacoub[email protected]18
- Research Article
- Medicine
- Microbiology and Infectious Disease
- dengue
- biomarkers
- prognostic
- Virus
- publisher-id67460
- doi10.7554/eLife.67460
- elocation-ide67460
Abstract
Early identification of severe dengue patients is important regarding patient management and resource allocation. We investigated the association of 10 biomarkers (VCAM-1, SDC-1, Ang-2, IL-8, IP-10, IL-1RA, sCD163, sTREM-1, ferritin, CRP) with the development of severe/moderate dengue (S/MD).
We performed a nested case-control study from a multi-country study. A total of 281 S/MD and 556 uncomplicated dengue cases were included.
On days 1–3 from symptom onset, higher levels of any biomarker increased the risk of developing S/MD. When assessing together, SDC-1 and IL-1RA were stable, while IP-10 changed the association from positive to negative; others showed weaker associations. The best combinations associated with S/MD comprised IL-1RA, Ang-2, IL-8, ferritin, IP-10, and SDC-1 for children, and SDC-1, IL-8, ferritin, sTREM-1, IL-1RA, IP-10, and sCD163 for adults.
Our findings assist the development of biomarker panels for clinical use and could improve triage and risk prediction in dengue patients.
This study was supported by the EU's Seventh Framework Programme (FP7-281803 IDAMS), the WHO, and the Bill and Melinda Gates Foundation.
Introduction
Dengue is the most common arboviral disease to affect humans globally. In 2019, the World Health Organization (WHO) identified dengue as one of the top 10 threats to global health 50World Health Organization2019. Transmission occurs in 129 countries, with an estimated 3.9 billion people being at risk 51World Health Organization2020. Over the last two decades, the number of reported cases per year has increased more than eight-fold 51World Health Organization2020, and in 2020 the annual number of dengue virus (DENV) infections was estimated to be 105 million, with 51 million cases being clinically apparent 4Cattarino et al.2020. With climate change, increased travel and urbanization, this rise is forecasted to continue over the coming decades 48Whitehorn and Yacoub201952Yacoub et al.2011. Despite the large disease burden, there is still no specific treatment for dengue, and the only licensed vaccine is recommended only in individuals with earlier dengue infection 31Redoni et al.2020.
In many dengue-endemic settings, seasonal epidemics can rapidly overwhelm fragile health systems. Although most symptomatic dengue infections are self-limiting, a small proportion of patients develop complications, most of which manifest at around 4–6 days from symptom onset. Thus, large numbers of patients require regular assessments to identify complications should they arise. The accurate and early identification of such patients, particularly within the first 3 days of illness in the febrile phase, should allow for appropriate care to be provided and potentially increase health system effectiveness. Although the 2009 WHO dengue guidelines set out specific warning signs for use in patient triage, utility of these guidelines at identifying those at risk for complications remains limited 21Morra et al.2018.
The pathogenesis of dengue involves a complex interplay between viral factors and the host response. It is hypothesized that an excessive immune response acting through inflammatory mediators can lead to the observed manifestations of bleeding, shock, and organ dysfunction. Studies have shown that in secondary infections, adaptive immune activation can result in high circulating levels of plasma cytokines and chemokines 15Katzelnick et al.201719Midgley et al.201137Screaton et al.2015. Binding of viral NS1 protein onto endothelial cells can act in concert with vasoactive substances, cytokines, and chemokines, to result in endothelial activation and glycocalyx disruption, and these processes likely underlie the increased vascular permeability and coagulopathy 18McBride and Khanh Lam202020Modhiran et al.201540St John et al.2013.
The role of blood biomarkers in predicting severe outcomes has been investigated in many studies, but mostly at later time-points or at hospital admission and many of these biomarkers either peak too late in the disease course or have too short a half-life to be clinically useful 1Ab-Rahman et al.201613John et al.201523Oliveira et al.201727Puerta-Guardo et al.201930Rathore et al.202032Robinson and Einav202034S S et al.201738Soo et al.201744Vasey et al.202055Yacoub et al.201754Yacoub et al.201656Yong et al.2017. Acknowledging these characteristics, we selected 10 candidate biomarkers from the vascular, immunological, and inflammatory pathways with good evidence supporting their involvement in the pathogenesis of dengue infection – focusing on those likely to be increased early in the disease course. We included vascular cell adhesion molecule-1 (VCAM-1), syndecan-1 (SDC-1), and angiopoietin-2 (Ang-2) because they represent endothelial activation and glycocalyx integrity 7Han et al.201917Mapalagamage et al.202041Suwarto et al.201753Yacoub et al.2016. For markers of immune activation, we measured interleukin-8 (IL-8) and interferon gamma-induced protein-10 (IP-10) as these are associated with disease severity 23Oliveira et al.201724Pandey et al.201529Rathakrishnan et al.2012, and IL-1 receptor antagonist (IL-1RA), soluble cluster of differentiation 163 (sCD163), and soluble triggering receptor expressed on myeloid cells-1 (sTREM-1) as these are activation markers of monocytes and macrophages, the major targets for dengue replication 1Ab-Rahman et al.201613John et al.201534S S et al.2017. For markers of general inflammation, we included ferritin and C-reactive protein (CRP) 1Ab-Rahman et al.20166Finkelstein et al.202022Mukherjee and Tripathi202039Soundravally et al.201545Vuong et al.2020.
The aims of this study were: (1) to investigate the association of these ten biomarkers with development of more severe dengue outcomes, (2) to find the best combination of biomarkers associated with more severe dengue outcomes. The results of the second aim could help in developing multiplex panels for use in outpatient settings to rapidly identify patients who require hospitalization.
Materials and methods
Study design
We conducted a nested case-control study using the samples and clinical information from a large multi-country observational study named ‘Clinical evaluation of dengue and identification of risk factors for severe disease’ (IDAMS study, NCT01550016) 12Jaenisch et al.2016. The IDAMS study and the blood sample analysis were approved by the Scientific and Ethics Committees of all study sites (Hospital for Tropical Diseases [Ho Chi Minh City, Vietnam] Ref No 03/HDDD-05/01/2018; Angkor Hospital for Children [Siem Reap, Cambodia] Ref No 0146/18-AHC; University of Malaya Medical Centre [Kuala Lumpur, Malaysia] Ref No 201865–6361) and by the Oxford Tropical Research Ethics Committee (OxTREC Ref No 502–18). There were 7428 participants in eight countries across Asia and Latin America enrolled in the IDAMS study. Patients were eligible for inclusion if they were aged 5 years or older, had fever or history of fever for less than 72 hr, and had symptoms consistent with dengue, with no features strongly suggestive of another disease. Participants were followed daily with a standard schedule of clinical examination and blood samples. Individual management (including hospitalization) was in accordance with routine practice at each study site. All diagnostic samples were processed and stored following specific protocols, and later transferred to designated sites for diagnostic testing in order to ensure consistency. Laboratory-confirmed dengue was defined by a positive reverse transcriptase polymerase chain reaction (RT-PCR) or a positive NS1 enzyme-linked immunosorbent assay (ELISA) result. Immune status was classified based on capture IgG results on paired samples. A probable primary infection was defined by two negative IgG results on two consecutive specimens taken at least 2 days apart, with at least one specimen obtained during the convalescent phase (after illness day 5). A probable secondary infection was defined by a positive IgG result identified during either or both the febrile and convalescent phases. In all other cases with the absence of suitable specimens at the appropriate time points immune status was classified as inconclusive. Each participant was given an overall severity grade (severe, moderate, or uncomplicated dengue), using all available information and a grading system in line with current guidelines and recommendations to classify clinical endpoints in dengue clinical trials 43Tomashek et al.2018.
Study population
Of the 2694 laboratory-confirmed dengue cases in the IDAMS study, 38 and 266 cases were classified as severe and moderate dengue respectively. For this study, we selected all severe and moderate cases from five study sites in four countries (Vietnam, Cambodia, Malaysia, and El Salvador), as residual plasma from these countries’ sample sets was available at the Oxford University Clinical Research Unit (OUCRU) in Ho Chi Minh City, Vietnam. For the control group, we selected patients with uncomplicated dengue with similar geographic and demographic characteristics at a 2:1 ratio. In total 281 cases and 556 controls were included in the analysis (Figure 1).
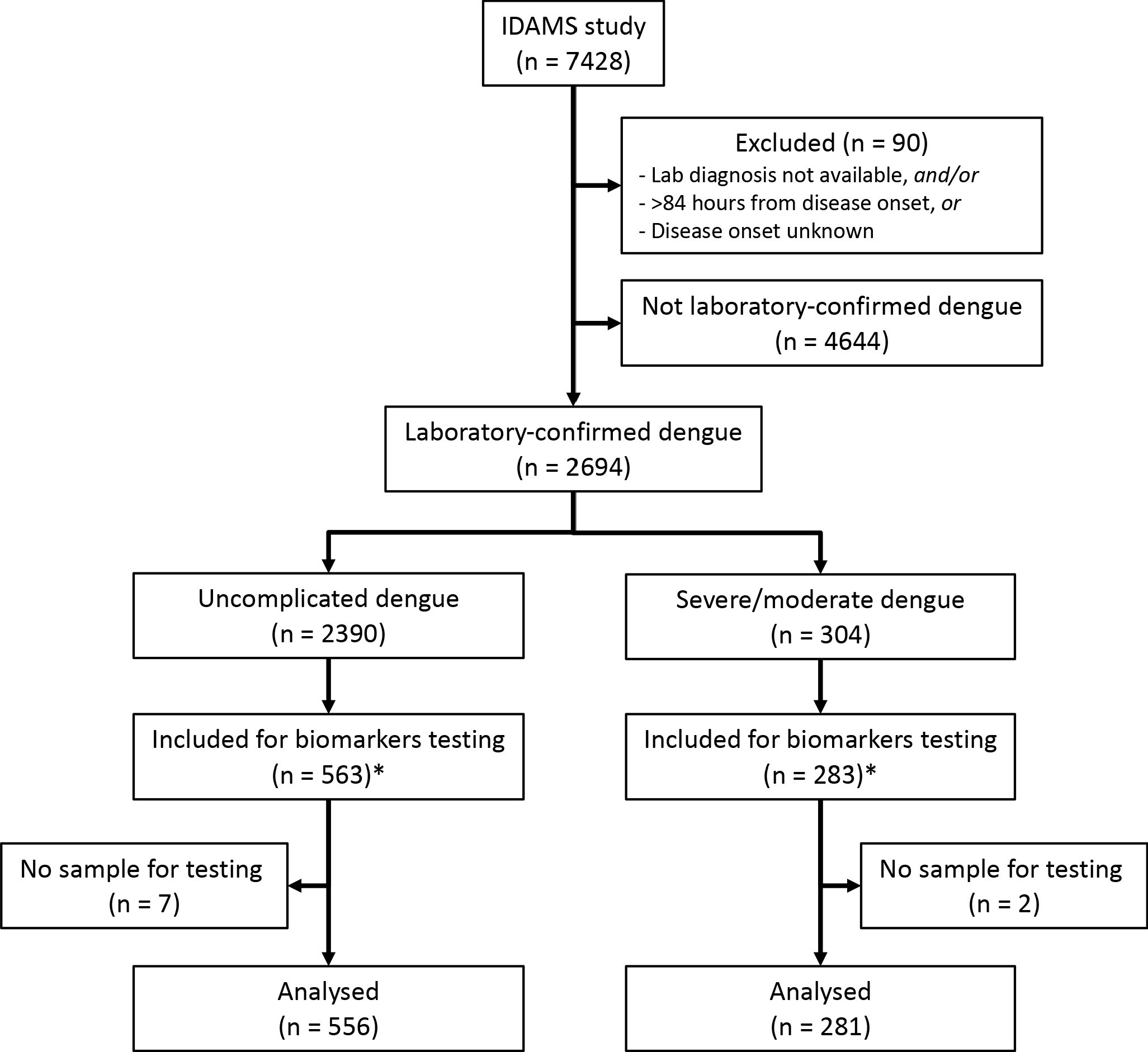
Study flowchart.
*The IDAMS study was performed in eight countries across Asia and Latin America. For this study, we selected cases in four countries (Vietnam, Cambodia, Malaysia, and El Salvador) as the blood samples were stored at the laboratory of the Oxford University Clinical Research Unit in Ho Chi Minh City, Vietnam.
Laboratory evaluation (details in Appendix 1)
The biomarkers were measured at two time points: at enrollment (illness day 1–3) and after recovery (day 10–31 post-symptom onset), if available. Eight biomarkers (CRP and ferritin excepted) were combined in a premixed magnetic bead panel (Cat No. LXSAHM; R and D). CRP was measured using a separate commercial magnetic bead panel (Cat. No. HCVD3MAG-67K; EMD Millipore Corporation). These panels were analyzed using the Luminex200 analyzer with the Luminex calibration (Cat. No. LX200-CAL-K25) and verification kits (Cat. No. LX200-CON-K25). Ferritin was measured using the Human Ferritin ELISA kit (Cat. No. ARG80501, Arigo). All tests were done according to the manufacturer’s specifications.
Study endpoints (details in Appendix 2)
The primary endpoint was combined severe and moderate dengue (S/MD), defined by the development of severe or moderate grades of any of the following – plasma leakage, haemorrhage, or organ impairment (including neurologic, hepatic, or cardiac involvement) (Appendix 2—table 1). We combined severe and moderate dengue to form the primary endpoint (S/MD) as severe dengue events were rare; this combined endpoint is relevant to clinical practice since the moderate group is likely to develop complications and therefore may also require medical intervention and hospitalization. We studied three secondary endpoints: severe dengue alone, severe dengue or dengue with warning signs according to the 2009 WHO classification, and hospitalization. These endpoints were selected as they also reflect the disease burden and severity and are generalizable across different settings. The decision to hospitalize was based only on clinical judgement and local guidelines particular to each study site, without use of any biomarker information.
Statistical analysis (details in Appendix 3)
Plasma levels of all biomarkers were transformed to the base-2 logarithm (log-2) before analysis as a right skewed distribution was apparent. We used a logistic regression model for all endpoints. We investigated the non-linear effects of all biomarkers and age on the endpoints, using restricted cubic splines with three knots at the 10th, 50th, and 90th percentiles.
For the first aim, that is to investigate the association of all biomarkers with the primary and secondary endpoints, we performed two different analyses: (1) fitting models for each biomarker separately (‘single models’) and (2) fitting models including all biomarkers together (‘global models’). In the ‘single models’ for a particular biomarker, only that biomarker along with age and their interaction were included, whereas in the ‘global models’ all the biomarkers along with their interactions with age were included. We performed the ‘global model’ in order to investigate the influence of the biomarkers when considering them together and this was also the initial step to develop models for the second aim. Results are reported as odds ratio (OR) and presented graphically.
For the second aim to find the best combination of biomarkers associated with the primary endpoint, we built upon the results from the first aim to fit separate models for children and adults (<15 versus ≥15 years of age), as differences were apparent by age. We used variable selection based on the ‘best subset’ approach 9Hastie et al.201711Hocking and Leslie1967. Briefly, this approach screened all possible combinations of biomarkers and selected the best based on the Akaike information criterion (AIC). We chose AIC as a ranking measurement because it quantifies goodness of fit, while guarding against over-fitting. The marker combination with the lowest AIC was taken as the best. From an ‘initial model’ including all biomarkers, we determined the best general combination and the best combinations of 2, 3, 4, and 5 biomarkers. We then performed a bootstrap procedure to check the robustness (stability) of the selected models. For this we resampled 1000 times with replacement from the original dataset. For each of these 1000 bootstrap samples, we performed the ‘best subset’ procedure similar to above to determine the best combination. We calculated the selection frequency of each marker combination over the 1000 samples. The frequency of the combination that was selected when using the original dataset in relation to the other combinations characterizes robustness of the selection.
We carried out several sensitivity analyses. First, we fitted the single and global models taking into account potential differences between serotypes by including serotype variable along with its interaction with the biomarkers. Second, we included viremia (viral RNA measured by RT-PCR) levels as an additional biomarker and performed the single model, global model and best subset procedure. Higher viremia levels have been associated with worse disease outcomes; however, viral load was not considered in the main analysis as the focus was on host markers with the potential for combining in a biomarker rapid test.
All analyses were done using the statistical software R version 3.6.3 28R Core Development Team2020 and the packages ‘rms’ 8Harrell Jr2019, ‘MuMIn’ 2Bartoń2020 and ‘ggplot2’ 49Wickham2016. The code is available on GitHub (47Vuong2021; copy archived at swh:1:rev:847d8e0f564eeb3f075b443205fb3384598bc2b4).
Results
Patient characteristics
The majority of the patients were from Vietnam (640 cases, 76%). Median (1st, 3rd quartiles) age of the case and control groups were 12 (9, 22) and 16 (10, 24) years. Among the S/MD group, 127 cases (45%) were children and 154 cases (55%) were adults. Male gender was predominant (60% and 54% in the case and control groups respectively). Serotype distribution was similar between the S/MD and control groups, with DENV-1 predominating (42%), particularly in children (48%). Host immune status however differed: there was a higher proportion of secondary infections in the S/MD group compared with controls (78% versus 64%, respectively) and this was consistent in both children and adults. The S/MD had a slightly lower percentage of obese patients than the control group (10% versus 14%). As expected, hospitalization was more common in the S/MD group (57% versus 31%) (Table 1). Overall, 38 patients developed severe dengue, most were severe plasma leakage (33/38 cases, 87%) and 29/38 (76%) were children. Most of the moderate dengue cases were plasma leakage and/or hepatic involvement (Appendix 4—table 1).
# Load packages
library(tidyverse)
library(gtsummary) # need to install package 'flextable'
library(rms)
library(MuMIn) # for best subset selection
library(facetscales) # need to install package 'facetscales' from devtools::install_github("zeehio/facetscales")
source("Elife ERA functions.R") # to include my functions
options(gtsummary.tbl_summary.percent_fun = function(x) sprintf(x * 100, fmt='%1.0f')) # to report percentages without decimal
options(knitr.kable.NA = '') # to set NA to '' in kable results
theme_set(theme_bw()) # I love black & white theme
options(na.action = "na.fail") # for 'dredge' function [MuMIn]
# Load full data
dat0 <- read_csv("Dengue_Biomarkers_data_27Jul2021.csv") %>%
mutate(group2 = factor(sev.or.inte, levels=c(0,1), labels=c("Uncomplicated dengue","Severe/moderate dengue")),
Country = as.factor(Country),
Serotype = as.factor(Serotype),
Serology = factor(Serology, levels = c("Probable primary", "Probable secondary", "Inconclusive")),
WHO2009 = factor(WHO2009, levels = c("Mild dengue", "Dengue with warning signs", "Severe dengue", "Unknown")))
# Data at enrollment (for Table 1 & Appendix 4-table 1)
dat <- dat0 %>% filter(Time == "Enrolment")
# Data at enrollment for models
## with inverse probability weights (IPW) for inclusion probability by countries (for the analysis of secondary outcomes)
## transform the biomarker's levels to log-2 and viremia to log-10
## set all biomarker's levels under the limit of detection (u...=1) to the limit of detection
dat1 <- dat %>%
mutate(age15 = factor(age15, levels=c("No","Yes"), labels=c("Under 15","15 and above")),
Serotype = ifelse(Serotype=="Unknown", NA, as.character(Serotype)),
Serotype = ifelse(Serotype=="DENV-1", 1, 2),
Serotype2 = Serotype, # for getting results for Appendix5-tables 1, 2
Serotype = factor(Serotype, levels=c(1,2), labels=c("DENV-1","Others")), # set Serotype to DENV-1 and others
ipw = ifelse(sev.or.inte==1, 1,
ifelse(Country=="Vietnam", (1505-204)/436,
ifelse(Country=="Malaysia", (259-29)/58,
ifelse(Country=="El Salvador", (306-18)/23,
ifelse(Country=="Cambodia", (302-30)/39, NA))))),
ipwsd = ipw * 837/2372,
VCAM = ifelse(uVCAM==1, log2(0.028), log2(VCAM1)),
SDC = log2(SDC1),
Ang = ifelse(uAng==1, log2(17.1), log2(Ang2)),
IL8 = ifelse(uIL8==1, log2(1.8), log2(IL8)),
IP10 = ifelse(uIP10==1, log2(1.18), log2(IP10)),
IL1RA = ifelse(uIL1RA==1, log2(18), log2(IL1RA)),
CD163 = log2(CD163),
TREM = ifelse(uTREM==1, log2(10.65), log2(TREM1)),
Fer = log2(Fer),
CRP = log2(CRP),
Vir = log10(Viremia)) %>%
select(Code, Age, age15, Serotype, Serotype2, sev.or.inte, sev.only, sev.or.ws, hospital, VCAM, SDC, Ang, IL8, IP10, IL1RA, CD163, TREM, Fer, CRP, Vir, uVCAM, uAng, uIL8, uIP10, uCD163, uTREM, uVir, ipw, ipwsd)
dat1c <- dat1 %>% filter(age15 == "Under 15") ## Data for children
dat1a <- dat1 %>% filter(age15 == "15 and above") ## Data for adults
# Set references for calculating the ORs and 95% CIs
ref0 <- c(median(dat1$VCAM), median(dat1$SDC), median(dat1$Ang), median(dat1$IL8), median(dat1$IP10), median(dat1$IL1RA), median(dat1$CD163), median(dat1$TREM), median(dat1$Fer), median(dat1$CRP))
# Create data for plotting biomarkers (for Figure 2 & Appendix 4-figure 1)
## Enrollment
tmp1 <- dat0 %>%
filter(Time == "Enrolment") %>%
select(Code, group2, Day, Daygr, VCAM1, SDC1, Ang2, IL8, IP10, IL1RA, CD163, TREM1, Fer, CRP) %>%
gather(., "Biomarker", "Result", 5:14)
## Follow up
tmp2 <- dat0 %>%
filter(Time == "Follow up") %>%
select(Code, group2, Day, Daygr, VCAM1, SDC1, Ang2, IL8, IP10, IL1RA, CD163, TREM1, Fer, CRP) %>%
gather(., "Biomarker", "Result", 5:14)
## Merge long data for biomarkers
dat_plot <- bind_rows(tmp1, tmp2) %>%
mutate(Daygr = factor(Daygr, levels = c("Day 1", "Day 2", "Day 3", "Day 10-20", "Day >20"),
labels = c("1", "2", "3", "10-20", ">20")),
Biomarker = factor(Biomarker, levels=c("VCAM1", "SDC1", "Ang2", "IL8", "IP10", "IL1RA", "CD163", "TREM1", "Fer", "CRP"),
labels=c("VCAM-1 (ng/ml)", "SDC-1 (pg/ml)", "Ang-2 (pg/ml)", "IL-8 (pg/ml)", "IP-10 (pg/ml)",
"IL-1RA (pg/ml)", "sCD163 (ng/ml)", "sTREM-1 (pg/ml)", "Ferritin (ng/ml)", "CRP (mg/l)")))
# All patients
t1 <- dat %>%
select(group2, Country, Age, Sex, Day, Serotype, Serology, Obesity, Diabetes, WHO2009, hospital) %>%
tbl_summary(by = group2,
statistic = list(all_continuous() ~ "{median} ({p25}, {p75})", all_categorical() ~ "{n} ({p})"),
value = list(Sex ~ "Male"),
digits = list(all_continuous() ~ c(0,0)),
label = list(Age ~ "Age (years)", Sex ~ "Gender male", Day ~ "Illness day at enrolment",
Serology ~ "Immune status", WHO2009 ~ "WHO 2009 classification", hospital ~ "Hospitalization")) %>%
add_stat_label(label = c(all_categorical() ~ "n (%)", Age ~ "median (1st, 3rd quartiles)")) %>%
modify_header(label = "", stat_by = "**{level} (N={n})**")
# Children (<15 years of age)
t2 <- dat %>%
filter(age15=="No") %>%
select(group2, Country, Age, Sex, Day, Serotype, Serology, Obesity, Diabetes, WHO2009, hospital) %>%
tbl_summary(by = group2,
statistic = list(all_continuous() ~ "{median} ({p25}, {p75})", all_categorical() ~ "{n} ({p})"),
value = list(Sex ~ "Male"),
digits = list(all_continuous() ~ c(0,0)),
label = list(Age ~ "Age (years)", Sex ~ "Gender male", Day ~ "Illness day at enrolment",
Serology ~ "Immune status", WHO2009 ~ "WHO 2009 classification", hospital ~ "Hospitalization")) %>%
add_stat_label(label = c(all_categorical() ~ "n (%)", Age ~ "median (1st, 3rd quartiles)")) %>%
modify_header(label = "", stat_by = "**{level} (N={n})**")
# Adults (>=15 years of age)
t3 <- dat %>%
filter(age15=="Yes") %>%
select(group2, Country, Age, Sex, Day, Serotype, Serology, Obesity, Diabetes, WHO2009, hospital) %>%
tbl_summary(by = group2,
statistic = list(all_continuous() ~ "{median} ({p25}, {p75})", all_categorical() ~ "{n} ({p})"),
value = list(Sex ~ "Male"),
digits = list(all_continuous() ~ c(0,0)),
label = list(Age ~ "Age (years)", Sex ~ "Gender male", Day ~ "Illness day at enrolment",
Serology ~ "Immune status", WHO2009 ~ "WHO 2009 classification", hospital ~ "Hospitalization")) %>%
add_stat_label(label = c(all_categorical() ~ "n (%)", Age ~ "median (1st, 3rd quartiles)")) %>%
modify_header(label = "", stat_by = "**{level} (N={n})**")
# Merge tables
tbl1 <- tbl_merge(tbls = list(t1, t2, t3),
tab_spanner = c("**All patients**", "**Children**", "**Adults**"))
# Convert the table to a dataframe for output as an HTML table
tbl1_formatted <- data.frame(
var = tbl1$table_body$label,
al1 = tbl1$table_body$stat_1_1,
al2 = tbl1$table_body$stat_2_1,
ch1 = tbl1$table_body$stat_1_2,
ch2 = tbl1$table_body$stat_2_2,
ad1 = tbl1$table_body$stat_1_3,
ad2 = tbl1$table_body$stat_2_3
)
names(tbl1_formatted) <- c(
"Variable",
"All, uncomplicated dengue (N=556)",
"All, severe/moderate dengue (N=281)",
"Children, uncomplicated dengue (N=337)",
"Children, severe/moderate dengue (N=127)",
"Adults, uncomplicated dengue (N=219)",
"Adults, severe/moderate dengue (N=154)"
)
tbl1_formatted
Summary of clinical data by primary outcome.
Obesity is defined as body mass index of higher than 30 kg/m2 (for patients of older than 18 years) or two standard deviations of the median of body mass index for age (for patients of 18 years or below). WHO: World Health Organization.
Biomarker levels
On average, the patients who progressed to S/MD had higher levels of the biomarkers in both children and adult patients, both at enrollment and at follow-up (Figure 2, Appendix 4—table 2). For most individuals, the levels of five biomarkers (VCAM-1, IL-8, IP-10, IL-1RA, and CRP) decreased between enrollment and follow-up, whereas SDC-1 increased slightly and the other markers showed no clear trends (Appendix 4—figure 1). In some of the cases the biomarkers did not return to normal at convalescence. Moderate-to-strong positive correlations were evident for some markers, in particular IP-10 and IL-1RA, and IP-10 and VCAM-1, both with Spearman’s rank correlation coefficients above 0.6 (Appendix 4—figure 2).
#' @width 28
#' @height 20
tick <- c(0,1,4,10,40,100,200,400,1000,4000,10000,20000,40000,70000) # for y-axis tick labels
dat_plot %>%
ggplot(., aes(Daygr, Result^(1/4), fill=group2, color=group2)) +
geom_boxplot(alpha=.5, outlier.size=.9, lwd=.4, fatten=1) +
geom_boxplot(alpha=0, outlier.color=NA, color="black", lwd=.4, fatten=1) +
facet_wrap(~ Biomarker, scales="free", ncol=5) +
scale_y_continuous(breaks=tick^(1/4), labels=tick) +
xlab("Illness day (day 1 [n=140]; day 2 [n=390]; day 3 [n=307]; day 10-20 [n=625]; day >20 [n=43])") +
theme(axis.title.y=element_blank(), legend.position="top", legend.title=element_blank(),
axis.text.y=element_text(size=rel(.8)))
Biomarker levels by groups.
VCAM-1: vascular cell adhesion molecule-1; SDC-1: syndecan-1; Ang-2: angiopoietin-2; IL-8: interleukin-8; IP-10: interferon gamma-induced protein-10; IL-1RA: interleukin-1 receptor antagonist; sCD163: soluble cluster of differentiation 163; sTREM-1: soluble triggering receptor expressed on myeloid cells-1; CRP: C-reactive protein. Y-axes are transformed using the fourth root transformation.
Associations between biomarker levels and the endpoints
In the single models, higher levels of each biomarker on illness days 1, 2, or 3 increased the risk of developing S/MD, with the exception of ferritin in adults where there was a downward trend at higher values (Figure 3, Table 2). We observed differences between children and adults for several biomarkers, the most pronounced being SDC-1, IL-8, ferritin, and IL-1RA. Associations between SDC-1 and IL-8 and the S/MD endpoint were stronger in adults than children, while the effects of IL-1RA and ferritin were stronger in children than adults.
#' @width 28
#' @height 20
# Set datadist for 'lrm' function [rms]
dd <- datadist(dat1); options(datadist="dd")
# Get results from models for children
dd$limits["Adjust to","Age"] <- 10
dat_m1 <- get_pred1(out="sev.or.inte", bio="VCAM", age=10, dat=dat1) %>% rename(value=VCAM) %>%
select(value, yhat, lower, upper, biomarker, model) %>% slice(which(dat1$age15=="Under 15"))
dat_m2 <- get_pred1(out="sev.or.inte", bio="SDC", age=10, dat=dat1) %>% rename(value=SDC) %>%
select(value, yhat, lower, upper, biomarker, model) %>% slice(which(dat1$age15=="Under 15"))
dat_m3 <- get_pred1(out="sev.or.inte", bio="Ang", age=10, dat=dat1) %>% rename(value=Ang) %>%
select(value, yhat, lower, upper, biomarker, model) %>% slice(which(dat1$age15=="Under 15"))
dat_m4 <- get_pred1(out="sev.or.inte", bio="IL8", age=10, dat=dat1) %>% rename(value=IL8) %>%
select(value, yhat, lower, upper, biomarker, model) %>% slice(which(dat1$age15=="Under 15"))
dat_m5 <- get_pred1(out="sev.or.inte", bio="IP10", age=10, dat=dat1) %>% rename(value=IP10) %>%
select(value, yhat, lower, upper, biomarker, model) %>% slice(which(dat1$age15=="Under 15"))
dat_m6 <- get_pred1(out="sev.or.inte", bio="IL1RA", age=10, dat=dat1) %>% rename(value=IL1RA) %>%
select(value, yhat, lower, upper, biomarker, model) %>% slice(which(dat1$age15=="Under 15"))
dat_m7 <- get_pred1(out="sev.or.inte", bio="CD163", age=10, dat=dat1) %>% rename(value=CD163) %>%
select(value, yhat, lower, upper, biomarker, model) %>% slice(which(dat1$age15=="Under 15"))
dat_m8 <- get_pred1(out="sev.or.inte", bio="TREM", age=10, dat=dat1) %>% rename(value=TREM) %>%
select(value, yhat, lower, upper, biomarker, model) %>% slice(which(dat1$age15=="Under 15"))
dat_m9 <- get_pred1(out="sev.or.inte", bio="Fer", age=10, dat=dat1) %>% rename(value=Fer) %>%
select(value, yhat, lower, upper, biomarker, model) %>% slice(which(dat1$age15=="Under 15"))
dat_m10 <- get_pred1(out="sev.or.inte", bio="CRP", age=10, dat=dat1) %>% rename(value=CRP) %>%
select(value, yhat, lower, upper, biomarker, model) %>% slice(which(dat1$age15=="Under 15"))
dat_mg1 <- get_pred2(out="sev.or.inte", bio="VCAM", age=10, dat=dat1) %>% rename(value=VCAM) %>%
select(value, yhat, lower, upper, biomarker, model) %>% slice(which(dat1$age15=="Under 15"))
dat_mg2 <- get_pred2(out="sev.or.inte", bio="SDC", age=10, dat=dat1) %>% rename(value=SDC) %>%
select(value, yhat, lower, upper, biomarker, model) %>% slice(which(dat1$age15=="Under 15"))
dat_mg3 <- get_pred2(out="sev.or.inte", bio="Ang", age=10, dat=dat1) %>% rename(value=Ang) %>%
select(value, yhat, lower, upper, biomarker, model) %>% slice(which(dat1$age15=="Under 15"))
dat_mg4 <- get_pred2(out="sev.or.inte", bio="IL8", age=10, dat=dat1) %>% rename(value=IL8) %>%
select(value, yhat, lower, upper, biomarker, model) %>% slice(which(dat1$age15=="Under 15"))
dat_mg5 <- get_pred2(out="sev.or.inte", bio="IP10", age=10, dat=dat1) %>% rename(value=IP10) %>%
select(value, yhat, lower, upper, biomarker, model) %>% slice(which(dat1$age15=="Under 15"))
dat_mg6 <- get_pred2(out="sev.or.inte", bio="IL1RA", age=10, dat=dat1) %>% rename(value=IL1RA) %>%
select(value, yhat, lower, upper, biomarker, model) %>% slice(which(dat1$age15=="Under 15"))
dat_mg7 <- get_pred2(out="sev.or.inte", bio="CD163", age=10, dat=dat1) %>% rename(value=CD163) %>%
select(value, yhat, lower, upper, biomarker, model) %>% slice(which(dat1$age15=="Under 15"))
dat_mg8 <- get_pred2(out="sev.or.inte", bio="TREM", age=10, dat=dat1) %>% rename(value=TREM) %>%
select(value, yhat, lower, upper, biomarker, model) %>% slice(which(dat1$age15=="Under 15"))
dat_mg9 <- get_pred2(out="sev.or.inte", bio="Fer", age=10, dat=dat1) %>% rename(value=Fer) %>%
select(value, yhat, lower, upper, biomarker, model) %>% slice(which(dat1$age15=="Under 15"))
dat_mg10 <- get_pred2(out="sev.or.inte", bio="CRP", age=10, dat=dat1) %>% rename(value=CRP) %>%
select(value, yhat, lower, upper, biomarker, model) %>% slice(which(dat1$age15=="Under 15"))
dat_p1 <- rbind(dat_m1,dat_mg1, dat_m2,dat_mg2, dat_m3,dat_mg3, dat_m4,dat_mg4, dat_m5,dat_mg5, dat_m6,dat_mg6, dat_m7,dat_mg7, dat_m8,dat_mg8, dat_m9,dat_mg9, dat_m10,dat_mg10) %>%
mutate(age = "10 years")
# Get results from models for adults
dd$limits["Adjust to","Age"] <- 25
dat_m1 <- get_pred1(out="sev.or.inte", bio="VCAM", age=25, dat=dat1) %>% rename(value=VCAM) %>%
select(value, yhat, lower, upper, biomarker, model) %>% slice(which(dat1$age15=="15 and above"))
dat_m2 <- get_pred1(out="sev.or.inte", bio="SDC", age=25, dat=dat1) %>% rename(value=SDC) %>%
select(value, yhat, lower, upper, biomarker, model) %>% slice(which(dat1$age15=="15 and above"))
dat_m3 <- get_pred1(out="sev.or.inte", bio="Ang", age=25, dat=dat1) %>% rename(value=Ang) %>%
select(value, yhat, lower, upper, biomarker, model) %>% slice(which(dat1$age15=="15 and above"))
dat_m4 <- get_pred1(out="sev.or.inte", bio="IL8", age=25, dat=dat1) %>% rename(value=IL8) %>%
select(value, yhat, lower, upper, biomarker, model) %>% slice(which(dat1$age15=="15 and above"))
dat_m5 <- get_pred1(out="sev.or.inte", bio="IP10", age=25, dat=dat1) %>% rename(value=IP10) %>%
select(value, yhat, lower, upper, biomarker, model) %>% slice(which(dat1$age15=="15 and above"))
dat_m6 <- get_pred1(out="sev.or.inte", bio="IL1RA", age=25, dat=dat1) %>% rename(value=IL1RA) %>%
select(value, yhat, lower, upper, biomarker, model) %>% slice(which(dat1$age15=="15 and above"))
dat_m7 <- get_pred1(out="sev.or.inte", bio="CD163", age=25, dat=dat1) %>% rename(value=CD163) %>%
select(value, yhat, lower, upper, biomarker, model) %>% slice(which(dat1$age15=="15 and above"))
dat_m8 <- get_pred1(out="sev.or.inte", bio="TREM", age=25, dat=dat1) %>% rename(value=TREM) %>%
select(value, yhat, lower, upper, biomarker, model) %>% slice(which(dat1$age15=="15 and above"))
dat_m9 <- get_pred1(out="sev.or.inte", bio="Fer", age=25, dat=dat1) %>% rename(value=Fer) %>%
select(value, yhat, lower, upper, biomarker, model) %>% slice(which(dat1$age15=="15 and above"))
dat_m10 <- get_pred1(out="sev.or.inte", bio="CRP", age=25, dat=dat1) %>% rename(value=CRP) %>%
select(value, yhat, lower, upper, biomarker, model) %>% slice(which(dat1$age15=="15 and above"))
dat_mg1 <- get_pred2(out="sev.or.inte", bio="VCAM", age=25, dat=dat1) %>% rename(value=VCAM) %>%
select(value, yhat, lower, upper, biomarker, model) %>% slice(which(dat1$age15=="15 and above"))
dat_mg2 <- get_pred2(out="sev.or.inte", bio="SDC", age=25, dat=dat1) %>% rename(value=SDC) %>%
select(value, yhat, lower, upper, biomarker, model) %>% slice(which(dat1$age15=="15 and above"))
dat_mg3 <- get_pred2(out="sev.or.inte", bio="Ang", age=25, dat=dat1) %>% rename(value=Ang) %>%
select(value, yhat, lower, upper, biomarker, model) %>% slice(which(dat1$age15=="15 and above"))
dat_mg4 <- get_pred2(out="sev.or.inte", bio="IL8", age=25, dat=dat1) %>% rename(value=IL8) %>%
select(value, yhat, lower, upper, biomarker, model) %>% slice(which(dat1$age15=="15 and above"))
dat_mg5 <- get_pred2(out="sev.or.inte", bio="IP10", age=25, dat=dat1) %>% rename(value=IP10) %>%
select(value, yhat, lower, upper, biomarker, model) %>% slice(which(dat1$age15=="15 and above"))
dat_mg6 <- get_pred2(out="sev.or.inte", bio="IL1RA", age=25, dat=dat1) %>% rename(value=IL1RA) %>%
select(value, yhat, lower, upper, biomarker, model) %>% slice(which(dat1$age15=="15 and above"))
dat_mg7 <- get_pred2(out="sev.or.inte", bio="CD163", age=25, dat=dat1) %>% rename(value=CD163) %>%
select(value, yhat, lower, upper, biomarker, model) %>% slice(which(dat1$age15=="15 and above"))
dat_mg8 <- get_pred2(out="sev.or.inte", bio="TREM", age=25, dat=dat1) %>% rename(value=TREM) %>%
select(value, yhat, lower, upper, biomarker, model) %>% slice(which(dat1$age15=="15 and above"))
dat_mg9 <- get_pred2(out="sev.or.inte", bio="Fer", age=25, dat=dat1) %>% rename(value=Fer) %>%
select(value, yhat, lower, upper, biomarker, model) %>% slice(which(dat1$age15=="15 and above"))
dat_mg10 <- get_pred2(out="sev.or.inte", bio="CRP", age=25, dat=dat1) %>% rename(value=CRP) %>%
select(value, yhat, lower, upper, biomarker, model) %>% slice(which(dat1$age15=="15 and above"))
dat_p2 <- rbind(dat_m1,dat_mg1, dat_m2,dat_mg2, dat_m3,dat_mg3, dat_m4,dat_mg4, dat_m5,dat_mg5, dat_m6,dat_mg6, dat_m7,dat_mg7, dat_m8,dat_mg8, dat_m9,dat_mg9, dat_m10,dat_mg10) %>%
mutate(age = "25 years")
# Merge results for children and adults
vis1 <- rbind(dat_p1, dat_p2) %>% mutate(outcome = "outcome 1")
# Merge data for plots
tmp0 <- dat1 %>% arrange(age15) %>% select(sev.or.inte)
tmp <- data.frame(sev.or.inte = rep(tmp0$sev.or.inte, 20))
tmp_p1 <- vis1 %>%
arrange(biomarker, model) %>%
mutate(value1 = 2^value,
age = factor(age, levels=c("10 years", "25 years")),
gr = ifelse(model=="Single model" & age=="10 years", 1,
ifelse(model=="Single model" & age=="25 years", 2,
ifelse(model=="Global model" & age=="10 years", 3, 4))),
gr = factor(gr, levels=c(1:4), labels=c("Single children", "Single adults", "Global children", "Global adults")),
model = factor(model, levels=c("Single model", "Global model"))) %>%
bind_cols(., tmp)
vline <- tmp_p1 %>%
filter(model=="Single model") %>%
filter(yhat==0) %>%
select(biomarker, value, value1) %>%
rename(vline=value, vline1=value1)
dat_p <- left_join(tmp_p1, vline, by="biomarker")
# Alternative data to limit to 5th - 95th of the values
dat_alt <- dat_p %>%
group_by(biomarker, model) %>%
mutate(up = quantile(value, .95),
lo = quantile(value, .05)) %>%
ungroup() %>%
mutate(is.outlier = value<lo | value>up | value<0,
value = ifelse(is.outlier, NA, value),
value1 = ifelse(is.outlier, NA, value1),
yhat = ifelse(is.outlier, NA, yhat),
lower = ifelse(is.outlier, NA, lower),
upper = ifelse(is.outlier, NA, upper))
dat_1_5 <- dat_alt %>%
filter(biomarker %in% c("VCAM", "SDC", "Ang", "IL8", "IP10")) %>%
mutate(biomarker = factor(biomarker, levels=c("VCAM", "SDC", "Ang", "IL8", "IP10")))
dat_6_10 <- dat_alt %>%
filter(biomarker %in% c("IL1RA", "CD163", "TREM", "Fer", "CRP")) %>%
mutate(biomarker = factor(biomarker, levels=c("IL1RA", "CD163", "TREM", "Fer", "CRP")))
# Modify facets' scales
require(facetscales)
xVCAM <- c(1,4,15,60,250,1000,4000)
xSDC <- c(1400,2000,2800,4000,5600)
xAng <- c(50,100,250,500,1000,2000)
xIL8 <- c(5,7,10,14,20,28,40)
xIP10 <- c(25,100,400,1600,6400)
xIL1RA <- c(1000,2000,4000,8000,16000)
xCD163 <- c(75,150,300,600)
xTREM <- c(35,50,70,100,140,200)
xFer <- c(50,100,200,400,800)
xCRP <- c(2.5,5,10,20,40,80)
scales_x <- list(
`VCAM` = scale_x_continuous(breaks = log2(xVCAM), labels = xVCAM),
`SDC` = scale_x_continuous(breaks = log2(xSDC), labels = xSDC),
`Ang` = scale_x_continuous(breaks = log2(xAng), labels = xAng),
`IL8` = scale_x_continuous(breaks = log2(xIL8), labels = xIL8),
`IP10` = scale_x_continuous(breaks = log2(xIP10), labels = xIP10),
`IL1RA` = scale_x_continuous(breaks = log2(xIL1RA), labels = xIL1RA),
`CD163` = scale_x_continuous(breaks = log2(xCD163), labels = xCD163),
`TREM` = scale_x_continuous(breaks = log2(xTREM), labels = xTREM),
`Fer` = scale_x_continuous(breaks = log2(xFer), labels = xFer),
`CRP` = scale_x_continuous(breaks = log2(xCRP), labels = xCRP)
)
ybreak1 <- c(.125, .25, .5, 1, 2, 4, 8)
ybreak2 <- c(.125, .25, .5, 1, 2, 4)
scales_y1 <- list(
`Single model` = scale_y_continuous(limits = c(log(.124), log(8.01)), breaks = log(ybreak1), labels = ybreak1),
`Global model` = scale_y_continuous(limits = c(log(.124), log(8.01)), breaks = log(ybreak1), labels = ybreak1)
)
scales_y2 <- list(
`Single model` = scale_y_continuous(limits = c(log(.124), log(4.01)), breaks = log(ybreak2), labels = ybreak2),
`Global model` = scale_y_continuous(limits = c(log(.124), log(4.01)), breaks = log(ybreak2), labels = ybreak2)
)
# Set facets' names
models <- c(`Single model` = "Single model",
`Global model` = "Global model")
biomarkers <- c(`VCAM` = "VCAM-1 (ng/ml)",
`SDC`= "SDC-1 (pg/ml)",
`Ang` = "Ang-2 (pg/ml)",
`IL8` = "IL-8 (pg/ml)",
`IP10` = "IP-10 (pg/ml)",
`IL1RA` = "IL-1RA (pg/ml)",
`CD163` = "sCD163 (ng/ml)",
`TREM` = "sTREM-1 (pg/ml)",
`Fer` = "Ferritin (ng/ml)",
`CRP` = "CRP (mg/l)")
# Plot for the first 5 biomarkers
p.1.5 <- ggplot(dat_1_5, aes(x=value)) +
geom_ribbon(aes(ymin=lower, ymax=upper, fill=age), alpha=.15) +
geom_vline(aes(xintercept=vline), color="black", linetype="dashed", alpha=.4) +
geom_hline(yintercept=0, color="black", linetype="dashed", alpha=.4) +
geom_line(aes(y=yhat, color=age), size=.5, alpha=.7) +
geom_rug(data=filter(dat_1_5, model=="Single model" & sev.or.inte==0 & age=="10 years"), sides="b", alpha=.2, color="red") +
geom_rug(data=filter(dat_1_5, model=="Single model" & sev.or.inte==1 & age=="10 years"), sides="t", alpha=.2, color="red") +
geom_rug(data=filter(dat_1_5, model=="Global model" & sev.or.inte==0 & age=="25 years"), sides="b", alpha=.2, color="blue") +
geom_rug(data=filter(dat_1_5, model=="Global model" & sev.or.inte==1 & age=="25 years"), sides="t", alpha=.2, color="blue") +
scale_color_manual(values=c("red","blue"), labels=c("Children","Adults")) +
scale_fill_manual(values=c("red","blue"), labels=c("Children","Adults")) +
ylab("Odds ratio") +
theme(legend.position="top", legend.title=element_blank(), axis.title.x=element_blank(), axis.text.x=element_text(angle=45)) +
facet_grid_sc(cols=vars(biomarker), rows=vars(model),
scales=list(x=scales_x, y=scales_y1),
labeller = as_labeller(c(models, biomarkers)))
# Plot for the last 5 biomarkers
p.6.10 <- ggplot(dat_6_10, aes(x=value)) +
geom_ribbon(aes(ymin=lower, ymax=upper, fill=age), alpha=.15) +
geom_vline(aes(xintercept=vline), color="black", linetype="dashed", alpha=.4) +
geom_hline(yintercept=0, color="black", linetype="dashed", alpha=.4) +
geom_line(aes(y=yhat, color=age), size=.5, alpha=.7) +
geom_rug(data=filter(dat_6_10, model=="Single model" & sev.or.inte==0 & age=="10 years"), sides="b", alpha=.2, color="red") +
geom_rug(data=filter(dat_6_10, model=="Single model" & sev.or.inte==1 & age=="10 years"), sides="t", alpha=.2, color="red") +
geom_rug(data=filter(dat_6_10, model=="Global model" & sev.or.inte==0 & age=="25 years"), sides="b", alpha=.2, color="blue") +
geom_rug(data=filter(dat_6_10, model=="Global model" & sev.or.inte==1 & age=="25 years"), sides="t", alpha=.2, color="blue") +
scale_color_manual(values=c("red","blue"), labels=c("Children","Adults")) +
scale_fill_manual(values=c("red","blue"), labels=c("Children","Adults")) +
ylab("Odds ratio") +
theme(legend.position="none", axis.title.x=element_blank(), axis.text.x=element_text(angle=45)) +
facet_grid_sc(cols=vars(biomarker), rows=vars(model),
scales=list(x=scales_x, y=scales_y2),
labeller = as_labeller(c(models, biomarkers)))
# Merge plots
gridExtra::grid.arrange(p.1.5, p.6.10, nrow=2, heights=c(1,.86))
Results from models for the primary endpoint (severe or moderate dengue).
The odds ratio of severe/moderate dengue (the red and blue lines) and 95% confidence interval (the red and blue regions) are estimated from multivariable logistic regression models allowing for a non-linear relation of log-2 of the biomarker level with severe/moderate dengue using restricted cubic splines. Each single model contains the corresponding biomarker, age and their interaction, while the global model contains all biomarkers and their interaction with age. The reference values for the odds ratios (where the odds ratio is equal to 1) are represented by the vertical gray dashed lines. They are chosen as the median of the biomarker levels of the whole study population (VCAM-1: 1636 ng/ml; SDC-1: 2519 pg/ml; Ang-2: 1204 pg/ml; IL-8: 14 pg/ml; IP-10: 3093 pg/ml; IL-1RA: 6434 pg/ml; sCD163: 295 ng/ml; sTREM-1: 85 ng/ml; ferritin: 243 ng/ml; and CRP: 28 mg/l). The x-axis represents biomarker levels; it is transformed using log-2 and its range truncated by the 5th and 95th percentiles of the biomarker levels of the whole study population. The rug plot on the x-axis represents the distribution of individual cases; the bottom rug plot represents the uncomplicated dengue cases and the top rug plot represents the severe/moderate dengue cases (children [<15 years of age] are in red and adults [≥15 years of age] are in blue). The red line and region represent children; results are shown for children at age of 10 years. The blue line and region represents adults; results are shown for adults at age of 25 years. VCAM-1: vascular cell adhesion molecule-1; SDC-1: syndecan-1; Ang-2: angiopoietin-2; IL-8: interleukin-8; IP-10: interferon gamma-induced protein-10; IL-1RA: interleukin-1 receptor antagonist; sCD163: soluble cluster of differentiation 163; sTREM-1: soluble triggering receptor expressed on myeloid cells-1; CRP: C-reactive protein.
# Set datadist for 'lrm' function [rms]
dd <- datadist(dat1); options(datadist="dd")
# Use my function 'get_est1' to get ORs and CIs from models
tmp1 <- data.frame(
sort1 = rep(c(1:10), 2),
sort2 = c(rep(2,10), rep(3,10)),
bio = rep(c("VCAM", "SDC", "Ang", "IL8", "IP10", "IL1RA", "CD163", "TREM", "Fer", "CRP"), 2),
ref1 = c(ref0-1, ref0),
ref2 = c(ref0, ref0+1)
) %>%
arrange(sort1, sort2) %>%
group_by(sort1, sort2) %>%
do(cbind(.,
# Children - single models
or1c = get_est1(out="sev.or.inte", bio=.$bio, ref1=.$ref1, ref2=.$ref2, est="OR", age=10, dat=dat1),
lo1c = get_est1(out="sev.or.inte", bio=.$bio, ref1=.$ref1, ref2=.$ref2, est="loCI", age=10, dat=dat1),
up1c = get_est1(out="sev.or.inte", bio=.$bio, ref1=.$ref1, ref2=.$ref2, est="upCI", age=10, dat=dat1),
# Children - global model
or2c = get_est2(out="sev.or.inte", bio=.$bio, ref1=.$ref1, ref2=.$ref2, est="OR", age=10, dat=dat1),
lo2c = get_est2(out="sev.or.inte", bio=.$bio, ref1=.$ref1, ref2=.$ref2, est="loCI", age=10, dat=dat1),
up2c = get_est2(out="sev.or.inte", bio=.$bio, ref1=.$ref1, ref2=.$ref2, est="upCI", age=10, dat=dat1),
# Adults - single models
or1a = get_est1(out="sev.or.inte", bio=.$bio, ref1=.$ref1, ref2=.$ref2, est="OR", age=25, dat=dat1),
lo1a = get_est1(out="sev.or.inte", bio=.$bio, ref1=.$ref1, ref2=.$ref2, est="loCI", age=25, dat=dat1),
up1a = get_est1(out="sev.or.inte", bio=.$bio, ref1=.$ref1, ref2=.$ref2, est="upCI", age=25, dat=dat1),
# Adults - global model
or2a = get_est2(out="sev.or.inte", bio=.$bio, ref1=.$ref1, ref2=.$ref2, est="OR", age=25, dat=dat1),
lo2a = get_est2(out="sev.or.inte", bio=.$bio, ref1=.$ref1, ref2=.$ref2, est="loCI", age=25, dat=dat1),
up2a = get_est2(out="sev.or.inte", bio=.$bio, ref1=.$ref1, ref2=.$ref2, est="upCI", age=25, dat=dat1))) %>%
ungroup()
for (i in 6:17) {tmp1[[i]] <- sprintf("%.2f", round(tmp1[[i]],2))}
# Use my function 'get_est1' to get p-values from models
tmp2 <- data.frame(
sort1 = c(1:10),
sort2 = rep(1,10),
bio = c("VCAM", "SDC", "Ang", "IL8", "IP10", "IL1RA", "CD163", "TREM", "Fer", "CRP")
) %>%
group_by(sort1) %>%
do(cbind(.,
p.s1 = get_est1(out="sev.or.inte", bio=.$bio, est="p", dat=dat1), # P overall from single models
p.s1_int = get_est1(out="sev.or.inte", bio=.$bio, est="p int", dat=dat1), # P interaction from single models
p.g1 = get_est2(out="sev.or.inte", bio=.$bio, est="p", dat=dat1), # P overall from global models
p.g1_int = get_est2(out="sev.or.inte", bio=.$bio, est="p int", dat=dat1))) %>% # P interaction from global models
ungroup()
for (i in 4:7) {tmp2[[i]] <- ifelse(tmp2[[i]]<0.001, "<0.001", sprintf("%.3f", round(tmp2[[i]],3)))}
# Combine results into a table
res1 <- bind_rows(tmp1, tmp2) %>%
arrange(sort1, sort2) %>%
mutate(bio = ifelse(!is.na(ref1), paste(" -", round(2^ref2,0), "vs", round(2^ref1,0), sep=" "), as.character(bio)),
or.sc1 = ifelse(is.na(lo1c), NA, paste(or1c, " (", lo1c, "-", up1c, ")", sep="")), # s: single model; c: children
or.gc1 = ifelse(is.na(lo2c), NA, paste(or2c, " (", lo2c, "-", up2c, ")", sep="")), # g: global model
or.sa1 = ifelse(is.na(lo1a), NA, paste(or1a, " (", lo1a, "-", up1a, ")", sep="")), # a: adults
or.ga1 = ifelse(is.na(lo2a), NA, paste(or2a, " (", lo2a, "-", up2a, ")", sep=""))) %>%
select(bio, or.sc1, or.sa1, p.s1, p.s1_int, or.gc1, or.ga1, p.g1, p.g1_int)
names(res1) <- c("Biomarker", "OR (95% CI) [children - single model]", "OR (95% CI) [adults - single model]",
"P-overall [single model]", "P-interaction [single model]",
"OR (95% CI) [children - global model]", "OR (95% CI) [adults - global model]",
"P-overall [global model]", "P-interaction [global model]")
# Report the results
res1
Results from models for the primary endpoint (severe or moderate dengue).
Poverall is derived from Wald test for the overall association of the biomarker with the endpoint; Pinteraction is from the test for the interaction between the biomarker and age. The odds ratios are estimated at age of 10 and 25 years, represented as children and adults respectively.
In the global model there were some differences compared to the single models (Figure 3, Table 2). The biomarkers SDC-1 and IL-1RA were the most stable relative to the single models for both children and adults. However, for IP-10 the trend of the association with S/MD changed from positive to negative in both children and adults. In children, VCAM-1 changed the trend from positive to weakly negative and IL-8 changed the trend from weakly positive to negative. Other biomarkers showed weaker associations with the endpoint in the global model based on the ORs. In addition, the differences of the associations between children and adults were more marked, particularly for Ang-2, IL-8, and ferritin.
The sensitivity analysis showed that the association between the biomarkers and S/MD did not differ between DENV-1 and other serotypes (Appendix 5—figure 1; Appendix 5—figure 2; Appendix 5—table 1; Appendix 5—table 2). Similar patterns were observed in the various analyses related to the secondary endpoints, as described in detail in the Appendix 6 (Appendix 6—figure 1; Appendix 6—figure 2, Appendix 6—table 1; Appendix 6—table 2, Appendix 6—table 3).
Best combinations of biomarkers associated with the primary endpoint
For children, the best subset that showed the clearest association with S/MD was the combination of the six markers IL-1RA, Ang-2, IL-8, ferritin, IP-10, and SDC-1 with an AIC of 465.9. This model was selected most often in the bootstrap procedure, but was not highly robust (it was selected in 134 of the 1000 samples) (Table 3, Appendix 7—table 1). Over the 1000 samples, the six variables had an inclusion frequency ranging from 73.5% for SDC-1 to 100% for IL-1RA. The most important biomarkers in order were IL-1RA, Ang-2, IL-8, and ferritin (Appendix 7—table 2). The best combination of two biomarkers was IL-1RA and ferritin, the best of three added Ang-2, the best of four added IP-10, and the best of five added IL-8. The best combinations of two and five variables were most robust with a selection percentage of 43.7% and 44%. The best of five had almost the same AIC as the best subset of six markers (467.6 versus 465.9) (Table 3). The coefficients of the selected biomarkers were similar to the initial model estimates (Appendix 7—table 2).
# EPV --------------------------------------------------------------
pred <- c("VCAM", "SDC", "Ang", "IL8", "IP10", "IL1RA", "CD163", "TREM", "Fer", "CRP")
# Estimate full model ----------------------------------------------
full_mod <- glm(sev.or.inte ~ VCAM + SDC + Ang + IL8 + IP10 + IL1RA + CD163 + TREM + Fer + CRP,
family=binomial, data=dat1c, x=T, y=T)
# Selected model ---------------------------------------------------
sel_var <- matrix(0, ncol=length(pred)+1, nrow=5, dimnames=list(NULL, c(pred, "aic")))
for (i in 1:5) {
if (i==1) {
bs <- dredge(full_mod, rank="AIC")
} else {
bs <- dredge(full_mod, rank="AIC", m.lim=c(i,i))
}
bs_var <- attr(get.models(bs, 1)[[1]]$terms, "term.labels")
for (j in 1:(ncol(sel_var)-1)) {sel_var[i,j] <- ifelse(names(sel_var[i,j]) %in% bs_var, 1, 0)}
formula <- paste("sev.or.inte~", paste(names(sel_var[i,][sel_var[i,]==1]), collapse = "+"))
sel_mod <- glm(formula, data = dat1c, family = binomial, x = T, y = T)
sel_var[i, ncol(sel_var)] <- AIC(sel_mod)
}
# Report results --------------------------------------------------
out1 <- as.data.frame(sel_var) %>% mutate(AIC = round(aic,1)) %>% select(-aic)
for (i in 1:(ncol(out1)-1)) {out1[,i] <- ifelse(out1[,i]==0, NA, "+")}
out2 <- as.data.frame(t(out1))
colnames(out2) <- c("Best of all combinations", "Best combination of 2 variables", "Best combination of 3 variables",
"Best combination of 4 variables", "Best combination of 5 variables")
rownames(out2) <- c("- VCAM-1", "- SDC-1", "- Ang-2", "- IL-8", "- IP-10", "- IL-1RA", "- sCD163", "- sTREM-1",
"- Ferritin", "- CRP", "AIC of the selected model")
out2
# For bootstrap results please look at Appendix 7-tables 1, 2 (for the best of all combinations)
# and the bootstrap codes for the best combinations of 2, 3, 4, and 5 variables
Best combinations of biomarkers associated with severe or moderate dengue for children.
VCAM-1: vascular cell adhesion molecule-1; SDC-1: syndecan-1; Ang-2: angiopoietin-2; IL-8: interleukin-8; IP-10: interferon gamma-induced protein-10; IL- 1RA: interleukin-1 receptor antagonist; sCD163: soluble cluster of differentiation 163; sTREM-1: soluble triggering receptor expressed on myeloid cells-1; CRP: C-reactive protein; AIC: Akaike information criterion.
For adults, the best subset included the seven markers SDC-1, IL-8, ferritin, sTREM-1, IL-1RA, IP-10, and sCD163. This model was selected 79 times among 1000 bootstrap samples, but still was selected more often than the other models (Table 4, Appendix 7—table 3). Over the 1000 samples, the seven variables had a bootstrap inclusion frequency ranging from 59.1% for sCD163 to 99.2% for SDC-1. The three most important biomarkers in order were SDC-1, IL-8, and ferritin (Appendix 7—table 4). The best combination of two biomarkers included SDC-1 and IL-8, the best of three added ferritin, the best of four added IL-1RA, and the best of five added sTREM-1. The best combination of two was the most robust with a selection percentage of 56.7%, followed by the best of three variables (43.2%) (Table 4). The coefficients of the selected markers were also similar to the initial model estimates (Appendix 7—table 4).
# EPV --------------------------------------------------------------
pred <- c("VCAM", "SDC", "Ang", "IL8", "ns1(IP10)", "IL1RA", "CD163", "TREM", "Fer", "CRP")
# Estimate full model ----------------------------------------------
full_mod <- glm(sev.or.inte ~ VCAM + SDC + Ang + IL8 + ns1(IP10) + IL1RA + CD163 + TREM + Fer + CRP,
family=binomial, data=dat1a, x=T, y=T)
# Selected model ---------------------------------------------------
sel_var <- matrix(0, ncol=length(pred)+1, nrow=5, dimnames=list(NULL, c(pred, "aic")))
for (i in 1:5) {
if (i==1) {
bs <- dredge(full_mod, rank="AIC")
} else {
bs <- dredge(full_mod, rank="AIC", m.lim=c(i,i))
}
bs_var <- attr(get.models(bs, 1)[[1]]$terms, "term.labels")
for (j in 1:(ncol(sel_var)-1)) {sel_var[i,j] <- ifelse(names(sel_var[i,j]) %in% bs_var, 1, 0)}
formula <- paste("sev.or.inte~", paste(names(sel_var[i,][sel_var[i,]==1]), collapse = "+"))
sel_mod <- glm(formula, data = dat1a, family = binomial, x = T, y = T)
sel_var[i, ncol(sel_var)] <- AIC(sel_mod)
}
# Report results --------------------------------------------------
out1 <- as.data.frame(sel_var) %>% mutate(AIC = round(aic,1)) %>% select(-aic)
for (i in 1:(ncol(out1)-1)) {out1[,i] <- ifelse(out1[,i]==0, NA, "+")}
out2 <- as.data.frame(t(out1))
colnames(out2) <- c("Best of all combinations", "Best combination of 2 variables", "Best combination of 3 variables",
"Best combination of 4 variables", "Best combination of 5 variables")
rownames(out2) <- c("- VCAM-1", "- SDC-1", "- Ang-2", "- IL-8", "- IP-10", "- IL-1RA", "- sCD163", "- sTREM-1",
"- Ferritin", "- CRP", "AIC of the selected model")
out2
# For bootstrap results please look at Appendix 7-tables 3, 4 (for the best of all combinations)
# and the bootstrap codes for the best combinations of 2, 3, 4, and 5 variables
Best combinations of biomarkers associated with severe or moderate dengue for adults.
VCAM-1: vascular cell adhesion molecule-1; SDC-1: syndecan-1; Ang-2: angiopoietin-2; IL-8: interleukin-8; IP-10: interferon gamma-induced protein-10; IL- 1RA: interleukin-1 receptor antagonist; sCD163: soluble cluster of differentiation 163; sTREM-1: soluble triggering receptor expressed on myeloid cells-1; CRP: C-reactive protein; AIC: Akaike information criterion. *Variable is kept as non-linear effect using natural cubic splines with three knots.
In the sensitivity analysis, viremia was not selected in any of the best combinations for children, and the marker combinations remained the same as the main analysis. For adults, the best subset included five markers SDC-1, IL-8, ferritin, viremia and sCD163. The best of two and three were the same as the main analysis; viremia was selected in the best of four and five (Appendix 8—figure 1; Appendix 8—table 1; Appendix 8—table 2; Appendix 8—table 3).
Discussion
This nested case-control study has shown that a range of endothelial, immune activation and inflammatory biomarkers measured during the early febrile phase of dengue are associated with progression to worse clinical outcomes in both children and adults. In children we found IL-1RA to have the most robust association with S/MD, whereas in adults we found SDC-1 and IL-8 to have the most robust association. For children, the best combination (ordered by robustness) included six biomarkers IL-1RA, Ang-2, IL-8, ferritin, IP-10, and SDC-1; for adults the best combination identified comprised seven biomarkers SDC-1, IL-8, ferritin, sTREM-1, IL-1RA, IP-10, and sCD163.
Our results add to the current literature on biomarkers in severe/moderate dengue compared with uncomplicated dengue, by including early time-points prior to the development of the severe manifestations, as well as providing data on the use of biomarker combinations, which takes into consideration the complex inflammatory-vascular pathogenesis of severe dengue. We observed that there were marked changes in the associations between individual biomarkers and outcomes when considering them together, while other biomarkers showed consistent associations. Particularly, the association of IP-10 with S/MD changed significantly from the single to global model, which may be because another biomarker in our model is a mediator or confounder of IP-10 in the pathway to the outcome. This could be IL-1RA as its association with S/MD was similar between the single and global model, and the correlation between IP-10 and IL-1RA was strong (Spearman’s rank correlation coefficient was 0.75). Nonetheless, changing the direction of the association from the single to global model does not diminish the possibility of that biomarker being selected in the best combinations.
Our study also demonstrates some key differences between pediatric and adult dengue. Clinical phenotypes of dengue in children and adults differ, with children experiencing more shock and adults more organ impairment and bleeding, with distinct clinical management guidelines published by the WHO. Our results imply dengue pathogenesis may differ by age, with distinct combinations of immune-activation and vascular markers demonstrated between children and adults. Specifically, the association of IL-8 and ferritin differed between children and adults, which is likely to be due to the composite endpoint of severe and moderate dengue. As shown in the analysis of severe dengue alone (Appendix 4—figure 1, Appendix 4—table 1), the effects of IL-8 and ferritin were similar in children and adults, which suggests these biomarkers are still associated with severe disease in all age groups and that the difference is driven by the moderate dengue group. In addition, uncomplicated dengue in adults have higher ferritin levels compared to in children, with increasing age and chronic conditions in adults likely contributing to this observation. Hence patients’ age should be considered when developing biomarker panels for dengue risk prediction.
The use of biomarker panels for the prediction of severe outcomes in dengue has been investigated in previous studies, using several statistical approaches 3Brasier et al.20125Conroy et al.201514Ju and Brasier201316Lee et al.201625Pang et al.2016. However, because of small sample size and differences in the biomarkers assessed, the associations found vary between studies and as yet there are no validated prognostic panels for dengue. Dengue cases are forecasted to increase over the next few decades and, given the limited healthcare resources available in many endemic settings, particularly during epidemics, there is an urgent need to develop innovative methods to rapidly identify patients likely to develop complications and require hospital care 33Rodriguez-Manzano et al.2018. Previously, we showed that CRP as a single biomarker was useful for early dengue diagnosis and risk identification, which is currently easy to use in all settings 45Vuong et al.2020. Recently, we also showed that higher plasma viremia was associated with increased dengue severity regardless of age, serotype and immune status of patients 46Vuong et al.2021. However, future point-of-care testing could be improved by using a combination of biomarkers outlined in this study. Our results are applicable to the development of point-of-care panels capable of multiplex analysis and suited for use in outpatient settings for dengue prognosis, with scope for incorporation with innovative point-of-care technologies. To be more applicable by balancing model fit, robustness, and parsimony, we suggest the combination of five biomarkers IL-1RA, Ang-2, IL-8, ferritin, and IP-10 for children, and the combination of three biomarkers SDC-1, IL-8, and ferritin for adults to be used in practice. These combinations had a similar AIC with the best combinations (the difference of AIC was less than 5), but they required fewer number of biomarkers in a test panel. With the advent of novel technologies including microarray platforms and multiplex lateral flow assays, the cost is likely to come down in the future, allowing for wide-spread use in low-to-middle-income countries.
Methods of variable selection have been discussed previously but there remains no clear consensus regarding the best approach 10Heinze et al.201835Sauerbrei et al.2020. We adopted a data-driven ‘best subset’ approach which we think offers advantages over other methods, given the complexity of the biomarkers involved and their interactions. We also explored other approaches for variable selection 10Heinze et al.201826Piironen and Vehtari201735Sauerbrei et al.2020 and the results were very similar to the best subset procedure (Appendix 9—table 1; Appendix 9—table 2).
Strengths of our study include the large sample size and use of a nested case-control dataset from a prospective multi-country cohort study with consistent data collection and standardized outcome definitions and laboratory methodologies. The biomarker panel we selected was guided by pathogenesis studies, focusing on pathways activated early in the disease course, thus ensuring clinical relevance.
There are some limitations in our study. One being we analysed the biomarkers at only one time-point in the early phase; limited financial resources did not allow us to evaluate the full range of biomarkers across the whole IDAMS population and at more time-points. Secondly, this study was not designed to build prediction models so we did not use a measure of predictive value as a criterion, which was motivated by the nested case-control design. Our findings need to be validated in new studies.
In conclusion, higher levels of the ten biomarkers (VCAM-1, SDC-1, Ang-2, IL-8, IP-10, IL-1RA, sCD163, sTREM-1, ferritin, and CRP), when considered individually, are associated with increased risk of adverse clinical outcomes in both children and adults with dengue. The best biomarker combination for children includes IL-1RA, Ang-2, IL-8, ferritin, IP-10, and SDC-1; for adults, SDC-1, IL-8, ferritin, sTREM-1, IL-1RA, IP-10, and sCD163 were selected. These findings serve to assist the development of biomarker panels to improve future triage and early assessment of dengue patients. This would aid not only individual patient management and facilitate healthcare allocation which would be of major public health benefit especially in outbreak settings, but could also serve as potential biological endpoints for dengue clinical trials.
References
-
- HAAb-Rahman
- HRahim
- SAbuBakar
- PFWong
-
- KBartoń
-
- ARBrasier
- HJu
- JGarcia
- HMSpratt
- SSVictor
- BMForshey
- ESHalsey
- GComach
- GSierra
- PJBlair
- CRocha
- ACMorrison
- TWScott
- IBazan
- TJKochel
- Venezuelan Dengue Fever Working Group
-
- LCattarino
- IRodriguez-Barraquer
- NImai
- DATCummings
- NMFerguson
-
- ALConroy
- MGélvez
- MHawkes
- NRajwans
- VTran
- WCLiles
- LAVillar-Centeno
- KCKain
-
- JLFinkelstein
- SColt
- AJLayden
- JTKrisher
- AMStewart-Ibarra
- MPolhemus
- EBeltrán-Ayala
- JMTedesco
- WBCárdenas
- TEndy
- SMehta
-
- LHan
- XAo
- SLin
- SGuan
- LZheng
- XHan
- HYe
-
- FEHarrell Jr
-
- THastie
- RTibshirani
- RJTibshirani
-
- GHeinze
- CWallisch
- DDunkler
-
- RRHocking
- RNLeslie
-
- TJaenisch
- DTTam
- NTKieu
- TVan Ngoc
- NTNam
- NVan Kinh
- SYacoub
- NChanpheaktra
- VKumar
- LLSee
- JSathar
- EPSandoval
- GMAlfaro
- ISLaksono
- YMahendradhata
- MSarker
- FAhmed
- ACaprara
- BSBenevides
- ETMarques
- TMagalhaes
- PBrasil
- MNetto
- ATami
- SEBethencourt
- MGuzman
- CSimmons
- NTQuyen
- LMerson
- NTDung
- DBeck
- MWirths
- MWolbers
- PKLam
- KRosenberger
- BWills
-
- DVJohn
- YSLin
- GCPerng
-
- HJu
- ARBrasier
-
- LCKatzelnick
- LGresh
- MEHalloran
- JCMercado
- GKuan
- AGordon
- ABalmaseda
- EHarris
-
- YHLee
- WYLeong
- AWilder-Smith
-
- MMapalagamage
- SMHandunnetti
- ARWickremasinghe
- GPremawansa
- SThillainathan
- TFernando
- KKanapathippillai
- ADDe Silva
- SPremawansa
-
- AMcBride
- PKhanh Lam
-
- CMMidgley
- MBajwa-Joseph
- SVasanawathana
- WLimpitikul
- BWills
- AFlanagan
- EWaiyaiya
- HBTran
- AECowper
- PChotiyarnwong
- PChotiyarnwon
- JMGrimes
- SYoksan
- PMalasit
- CPSimmons
- JMongkolsapaya
- GRScreaton
-
- NModhiran
- DWatterson
- DAMuller
- AKPanetta
- DPSester
- LLiu
- DAHume
- KJStacey
- PRYoung
-
- MEMorra
- AMAAltibi
- SIqtadar
- LHNMinh
- SSElawady
- AHallab
- AElshafay
- OAOmer
- AIraqi
- PAdhikari
- JHLabib
- KMElhusseiny
- AElgebaly
- SYacoub
- LTMHuong
- KHirayama
- NTHuy
-
- SMukherjee
- ATripathi
-
- ROliveira
- MTCordeiro
- PMoura
- PNBBaptista Filho
- UMBraga-Neto
- ETAMarques
- LGil
-
- NPandey
- AJain
- RKGarg
- RKumar
- OPAgrawal
- PVLakshmana Rao
-
- JPang
- ALindblom
- TTolfvenstam
- TLThein
- ANNaim
- LLing
- AChow
- MIChen
- EEOoi
- YSLeo
- MLHibberd
-
- JPiironen
- AVehtari
-
- HPuerta-Guardo
- DRGlasner
- DAEspinosa
- SBBiering
- MPatana
- KRatnasiri
- CWang
- PRBeatty
- EHarris
-
- R Core Development Team
-
- ARathakrishnan
- SMWang
- YHu
- AMKhan
- SPonnampalavanar
- LCLum
- RManikam
- SDSekaran
-
- APRathore
- FSFarouk
- ALSt John
-
- MRedoni
- SYacoub
- LRivino
- DRGiacobbe
- RLuzzati
- SDi Bella
-
- MRobinson
- SEinav
-
- JRodriguez-Manzano
- PYChia
- TWYeo
- AHolmes
- PGeorgiou
- SYacoub
-
- SGS S
- ABPillai
- VSRamachandrappa
- TKadhiravan
- RDhodapkar
- JKah
- SRajendiran
-
- WSauerbrei
- APerperoglou
- MSchmid
- MAbrahamowicz
- HBecher
- HBinder
- DDunkler
- FEHarrell
- PRoyston
- GHeinze
- for TG2 of the STRATOS initiative
-
- EDSchifano
-
- GScreaton
- JMongkolsapaya
- SYacoub
- CRoberts
-
- KMSoo
- BKhalid
- SMChing
- CLTham
- RBasir
- HYChee
-
- RSoundravally
- BAgieshkumar
- MDaisy
- JSherin
- CCCleetus
-
- ALSt John
- APRathore
- BRaghavan
- MLNg
- SNAbraham
-
- SSuwarto
- RTSasmono
- RSinto
- EIbrahim
- MSuryamin
-
- EJTchetgen Tchetgen
-
- KMTomashek
- BWills
- LCSee Lum
- LThomas
- ADurbin
- YSLeo
- NBosch
- ERojas
- KHendrickx
- MErpicum
- LAgulto
- TJaenisch
- HTissera
- PSuntarattiwong
- BACollers
- DWallace
- ACSchmidt
- APrecioso
- FNarvaez
- SJThomas
- REdelman
- JBSiqueira
- MCCassetti
- WDempsey
- DJGubler
-
- BVasey
- AHShankar
- BBHerrera
- ABecerra
- KXhaja
- MEchenagucia
- SRMachado
- DCaicedo
- JMiller
- PAmedeo
- ENNaumova
- IBosch
- Norma Blumenfeld deBosch
-
- NLVuong
- HTLe Duyen
- PKLam
- DTHTam
- NVVinh Chau
- NVan Kinh
- NChanpheaktra
- LCSLum
- EPleités
- NKJones
- CPSimmons
- KRosenberger
- TJaenisch
- CHalleux
- PLOlliaro
- BWills
- SYacoub
-
- NLVuong
- NTHQuyen
- NTHTien
- NMTuan
- DTHKien
- PKLam
- DTHTam
- TVan Ngoc
- SYacoub
- TJaenisch
- RBGeskus
- CPSimmons
- BAWills
-
- NLVuong
-
- JWhitehorn
- SYacoub
-
- HWickham
-
- World Health Organization
-
- World Health Organization
-
- SYacoub
- SKotit
- MHYacoub
-
- SYacoub
- PKLam
- LHMVu
- TLLe
- NTHa
- TTToan
- NTVan
- NTHQuyen
- HTLe Duyen
- NVan Kinh
- AFox
- JMongkolspaya
- MWolbers
- CPSimmons
- GRScreaton
- HWertheim
- BWills
-
- SYacoub
- JMongkolsapaya
- GScreaton
-
- SYacoub
- PKLam
- TTHuynh
- HHNguyen Ho
- HTDong Thi
- NTVan
- LTLien
- QNTHa
- DHTLe
- JMongkolspaya
- ACulshaw
- TWYeo
- HWertheim
- CSimmons
- GScreaton
- BWills
-
- YKYong
- HYTan
- SHJen
- EMShankar
- SKNatkunam
- JSathar
- RManikam
- SDSekaran