Evolution of C4 photosynthesis predicted by constraint-based modelling
- Mary-AnnBlätke[email protected]1
- AndreaBräutigam12
- Research Article
- Computational and Systems Biology
- Plant Biology
- metabolic networks
- constraint-based model
- C4 photosynthesis
- model evolution
- flux balance analysis
- None
- publisher-id49305
- doi10.7554/eLife.49305
- elocation-ide49305
Abstract
Constraint-based modelling (CBM) is a powerful tool for the analysis of evolutionary trajectories. Evolution, especially evolution in the distant past, is not easily accessible to laboratory experimentation. Modelling can provide a window into evolutionary processes by allowing the examination of selective pressures which lead to particular optimal solutions in the model. To study the evolution of C4 photosynthesis from a ground state of C3 photosynthesis, we initially construct a C3 model. After duplication into two cells to reflect typical C4 leaf architecture, we allow the model to predict the optimal metabolic solution under various conditions. The model thus identifies resource limitation in conjunction with high photorespiratory flux as a selective pressure relevant to the evolution of C4. It also predicts that light availability and distribution play a role in guiding the evolutionary choice of possible decarboxylation enzymes. The data shows evolutionary CBM in eukaryotes predicts molecular evolution with precision.
Introduction
Identifying specific evolutionary trajectories and modelling the outcome of adaptive strategies at the molecular levels is a major challenge in evolutionary systems biology 55Papp et al.2011. The evolution of novel metabolic pathways from existing parts may be predicted using constraint-based modelling (CBM) 52Orth et al.2010. In CBM, selective pressures are coded via the objective functions for which the model is optimised. The factors which constrain evolution are integrated into the models via changes in model inputs or outputs and via flux constraints. We hypothesised that the evolution of the agriculturally important trait of C4 photosynthesis is accessible to CBM.
C4 photosynthesis evolved independently in at least 67 independent origins in the plant kingdom 65Scheben et al.2017 and it allows colonisation of marginal habitats 63Sage et al.2012 and high biomass production in annuals such as crops 62Sage200423Edwards et al.2010. The C4 cycle acts as a biochemical pump which enriches the CO2 concentration at the site of Rubisco to overcome a major limitation of carbon fixation 62Sage2004. Enrichment is beneficial because Rubisco, the carbon fixation enzyme, can react productively with CO2 and form two molecules of 3-PGA, but it also reacts with O2 and produces 2-phosphoglycolate which requires detoxification by photorespiration 51Ogren and Bowes1971. The ratio between both reactions is determined by the enzyme specificity towards CO2, by the temperature, and the concentrations of both reactants, which in turn is modulated by stresses such as drought and pathogen load. Evolution of Rubisco itself is constrained since any increase in specificity is paid for by a reduction in speed 73Spreitzer and Salvucci2002. Lower speeds most likely cause maladaptivity since Rubisco is a comparatively slow enzyme and can comprise up to 50% of the total leaf protein 24Ellis1979. In the C4 cycle, phosphoenolpyruvate carboxylase affixes CO2 to a C3 acid, phosphoenolpyruvate (PEP), forming a C4 acid, oxaloacetate (OAA). After stabilisation of the resulting C4 acid by reduction to malate or transamination to aspartate, it is transferred to the site of Rubisco and decarboxylated by one of three possible decarboxylation enzymes, NADP-dependent malic enzyme (NADP-ME), NAD-dependent malic enzyme (NAD-ME), or PEP carboxykinase (PEP-CK) 30Hatch198767Schlüter et al.2016. Species such as corn (Zea mays) 57Pick et al.2011 and great millet (Sorghum bicolor) 20Döring et al.2016 use NADP-ME, species like common millet (Panicum miliaceum) 30Hatch1987 and African spinach (Gynandropsis gynandra) 25Feodorova et al.201082Voznesenskaya et al.2007 use NAD-ME and species such as guinea grass (Panicum maximum) 12Bräutigam et al.2014 use mainly PEP-CK with the evolutionary constraints leading to one or the other enzyme unknown. Mixed forms are only known to occur between a malic enzyme and PEP-CK but not between both malic enzymes 83Wang et al.2014. After decarboxylation, the C3 acid diffuses back to the site of phosphoenolpyruvate carboxylase (PEPC) and is recycled for another C4 cycle by pyruvate phosphate dikinase (PPDK) 30Hatch198767Schlüter et al.2016. All the enzymes involved in the C4 cycle are also present in C3 plants 4Aubry et al.2011. In its most typical form, this C4 cycle is distributed between different cell types in a leaf in an arrangement called Kranz anatomy 29Haberlandt and Engelmann1904. Initial carbon fixation by PEPC occurs in the mesophyll cell, the outer layer of photosynthetic tissue. The secondary fixation by Rubisco after decarboxylation occurs in an inner layer of photosynthetic tissue, the bundle sheath which in turn surrounds the veins. Both cells are connected by plasmodesmata which are pores with limited transfer specificity between cells. A model which may test possible carbon fixation pathways at the molecular level thus requires two cell architectures connected by transport processes 15Bräutigam and Weber2010.
CBM of genome-scale or close to it are well suited to study evolution (summarised in 55Papp et al.2011). Evolution of different metabolic modes from a ground state, the metabolism of Escherichia coli, such as glycerol usage 39Lewis et al.2010 or endosymbiotic metabolism 54Pál et al.2006 have been successfully predicted. Metabolic maps of eukaryotic metabolism are of higher complexity compared to bacteria since they require information about intracellular compartmentation and intracellular transport 21Duarte2004 and may require multicellular approaches. In plants, aspects of complex metabolic pathways, such as the energetics of CAM photosynthesis 17Cheung et al.2014, and fluxes in C3 and C4 metabolism 11Boyle and Morgan200928Gomes de Oliveira Dal’Molin et al.201119de Oliveira Dal'Molin et al.20102Arnold and Nikoloski201464Saha et al.2011 have been elucidated with genome scale models. The C4 cycle is not predicted by these current C4 models unless the C4 cycle is forced by constraints 28Gomes de Oliveira Dal’Molin et al.201147Mallmann et al.2014. In the C4GEM model, the fluxes representing the C4 cycle are a priori constrained to the cell types 28Gomes de Oliveira Dal’Molin et al.2011, and in the Mallmann model, the C4 fluxes are induced by activating flux through PEPC 47Mallmann et al.2014. Models in which specific a priori constraints activated C4 were successfully used to study metabolism under conditions of photosynthesis, photorespiration, and respiration 64Saha et al.2011 and to study N-assimilation under varying conditions 71Simons et al.2013. However, they are incapable of testing under which conditions the pathway may evolve.
Schematic models suggest that the C4 cycle evolves from its ancestral metabolic state C3 photosynthesis along a sequence of stages (summarised in 62Sage2004; 14Bräutigam and Gowik2016). In the presence of tight vein spacing and of photosynthetically active bundle sheath cells (i.e. Kranz anatomy), a key intermediate in which the process of photorespiration is divided between cell types is thought to evolve 48Monson199963Sage et al.201231Heckmann et al.20136Bauwe2010. The metabolic fluxes in this intermediate suggest an immediate path towards C4 photosynthesis 47Mallmann et al.201414Bräutigam and Gowik2016. 31Heckmann et al.2013 built a kinetic model in which the complex C4 cycle was represented by a single enzyme, PEPC. Assuming carbon assimilation as a proxy for fitness, the model showed that the evolution from a C3 progenitor species with Kranz-type anatomy towards C4 photosynthesis occurs in modular, individually adaptive steps on a Mount Fuji fitness landscape. It is frequently assumed that evolution of C4 photosynthesis requires water limitation 14Bräutigam and Gowik201631Heckmann et al.201347Mallmann et al.2014. However, ecophysiological research showed that C4 can likely evolve in wet habitats 53Osborne and Freckleton200944Lundgren and Christin2017. CBM presents a possible avenue to study the evolution of C4 photosynthesis including its metabolic complexity in silico.
In this study, we establish a generic two-celled, constraint-based model starting from the Arabidopsis core model 2Arnold and Nikoloski2014. We test under which conditions and constraints C4 photosynthesis is predicted as the optimal solution. Finally, we test which constraints result in the prediction of the particular C4 modes with their different decarboxylation enzymes. In the process, we demonstrate that evolution is predictable at the molecular level in an eukaryotic system and define the selective pressures and limitations guiding the 'choice' of metabolic flux.
Materials and methods
Flux Balance Analysis
Flux balance analysis (FBA) is a CBM approach 52Orth et al.2010 to investigate the steady-state behaviour of a metabolic network defined by its stoichiometric matrix . By employing linear programming, FBA allows computing an optimised flux distribution that minimises and/or maximises the synthesis and/or consumption rate of one specific metabolite or a combination of various metabolites. Next to the steady-state assumption and stoichiometric matrix , FBA relies on the definition of the reaction directionality and reversibility, denoted by the lower bound and upper bound , as well as the definition of an objective function . The objective function defines a flux distribution , with respect to an objective .
The degeneracy problem, the possible existence of alternate optimal solutions, is one of the major issues of constraint-based optimisation, such as FBA 46Mahadevan and Schilling2003. To avoid this problem, we use the parsimonious version of FBA (pFBA) 39Lewis et al.2010. This approach incorporates the flux parsimony as a constraint to find the solution with the minimum absolute flux value among the alternative optima, which is in agreement with the assumption that the cell is evolutionary optimised to allocate a minimum amount of resources to achieve its objective.
All FBA experiments in this study employ pFBA and are performed using the cobrapy module in a python 2.7 environment run on a personal computer (macOS Sierra, 4 GHz Intel Core i7, 32 GB 1867 MHz DDR3). All FBA experiments are available as jupyter notebooks in the supplementary material and can also be accessed and executed from the GitHub repository https://github.com/ma-blaetke/CBM_C3_C4_Metabolism (10Blätke2019; copy archived at https://github.com/elifesciences-publications/CBM_C3_C4_Metabolism).
##### Import Modules #####
## numpy
import numpy as np
## pandas
import pandas as pd
pd.set_option('display.max_rows', None)
pd.set_option('display.max_columns', None)
pd.set_option('display.width', None)
pd.set_option('display.max_colwidth', None)
## plotly
import plotly
import plotly.graph_objects as go
from plotly.subplots import make_subplots
## cobra
import cobra
## escher
from escher import Builder
## ipython HTML display
from IPython.display import HTML
## goatools
from goatools import obo_parser
## tqdm
from tqdm.notebook import trange, tqdm
## string
import string
display(HTML(f"<h1>Code Cell 1: Import Python Modules</h1>"))
Code Cell 1: Import Python Modules
display(HTML(f"<h1>Code Cell 2: Set Parameters</h1>"))
##### Set Parameters #####
inf = float(1e6)
Code Cell 2: Set Parameters
display(HTML(f"<h1>Code Cell 3: Define Functions </h1>"))
##### Define Functions - SBML Model and FBA #####
## Load SBML model
def load_sbml_model():
'''
Return cobra model
Parameters:
Returns:
cobra_model (cobra.model): cobra model of specified sbm file
'''
sbml_file = 'elife-49305.ipython.src/2018-23-05-mb-genC3.sbml'
cobra_model = cobra.io.sbml.read_sbml_model(sbml_file)
return cobra_model
## Add reactions to model
def add_rxn(name, D_mets, model, rev=True):
'''
Add new reaction to cobra model
Parameters:
name (str): short name/id of reaction
D_mets (dict): dictionary of metabolites and their stoichimetric coeffcients attending at reaction
model (cobra.model): cobra model to add the reaction
rev (bool): reversiblity of reaction (default: True)
Returns:
'''
r_name = name
r_obj = cobra.Reaction(rname)
r_obj.name = r_name
r_obj.id = r_name
model.add_reaction(r_obj)
r_obj.add_metabolites(D_mets)
r_obj.objective_coefficient = 0
r_obj.bounds = (-inf,inf) if rev else (0,inf)
## Set flux of a reaction to a fixed value
def set_fixed_flux(r_id, val, model):
'''
Set flux of reaction to a fixed value
Parameters:
r_id (str): reaction id
val (float): flux value
model (cobra.model): cobra model to add the reaction
Returns:
'''
r_obj = model.reactions.get_by_id(r_id)
r_obj.bounds = (val,val)
## Set lower and upper flux bound of a reaction
def set_bounds(r_id, val_tuple, model):
'''
Set flux bounds of reaction
Parameters:
r_id (str): reaction id
val_tuple (tuple): (lower_bound, upper_bound)
model (cobra.model): cobra model to add the reaction
Returns:
'''
r_obj = model.reactions.get_by_id(r_id)
r_obj.bounds = val_tuple
## Set flux ratio for two reactions
def set_fixed_flux_ratio(r_dict, name, model):
'''
Set flux ratio of two reactions
Parameters:
r_dict (dict): rdictionary with two keys (reaction ids) and their proportion in the ratio {r_id1: prop1, r_ids2: prop2}
name (str): name of constraint
model (cobra.model): cobra model to add the reaction
Returns:
'''
if len(r_dict) == 2:
r_id1 = list(r_dict.keys())[0]
r_obj1 = model.reactions.get_by_id(r_id1)
r_v1 = list(r_dict.values())[0]
r_id2 = list(r_dict.keys())[1]
r_obj2 = model.reactions.get_by_id(r_id2)
r_v2 = list(r_dict.values())[1]
const = model.problem.Constraint(
float(r_v1) * r_obj2.flux_expression - float(r_v2) * r_obj1.flux_expression,
lb = 0.0,
ub = 0.0,
name = name)
model.add_cons_vars(const)
return const
Code Cell 3: Define Functions
Generic model for C3 metabolism
Metabolic model
The generic model representing the metabolism of a mesophyll cell of a mature photosynthetically active C3 leaf, further on called one-cell model, is based on the Arabidopsis core model 2Arnold and Nikoloski2014. The model is compartmentalised into cytosol (c), chloroplast (h), mitochondria (m), and peroxisome (p). Each reaction in the Arabidopsis core model 2Arnold and Nikoloski2014 was compared with the corresponding entry in AraCyc 49Mueller et al.2003. Based on the given information, we corrected co-factors, gene associations, enzyme commission numbers and reversibility (information from BRENDA 68Schomburg et al.2002 were included). The gene associations and their GO terms 3Ashburner et al.2000 of the cellular components were used to correct the location of reactions. Major additions to the model are the cyclic electron flow 70Shikanai2016, alternative oxidases in mitochondria and chloroplast 81Vishwakarma et al.2015, as well as several transport processes between the compartments and the cytosol 42Linka and Weber2010. NAD-dependent dehydrogenase to oxidise malate is present in all compartments 27Gietl19929Berkemeyer et al.1998, which excludes the interconversion of NAD and NADP by cycles through the nitrate reductase present in the Arabidopsis core model. Correctly defining the protonation state of the metabolites in the various cellular compartments is a general drawback of metabolic models due to the lack of knowledge in that area. This issue mainly affects biochemical reactions and transport reactions involving protons. We added a sink/source reaction for protons in the form:
to all compartments to prevent futile fluxes of protons and other metabolites coupled through the proton transport. The curated one-cell model is provided in Figure 1—source data 1.
display(HTML(f"<h1>Code Cell 4: Load Metabolic Model of C3 Photosynthesis in Arabidposis thaliana</h1>"))
##### Load model #####
c3_model = load_sbml_model()
# Model summary
c3_num_mets = len(c3_model.metabolites)
c3_num_rxn = len(c3_model.reactions)
c3_num_transport_rxn = len(c3_model.reactions.query(lambda rxn: (rxn.id.startswith('Tr_'))))
c3_num_export_rxn = len(c3_model.reactions.query(lambda rxn: (rxn.id.startswith('Ex_'))))
c3_num_import_rxn = len(c3_model.reactions.query(lambda rxn: (rxn.id.startswith('Im_'))))
df_c3_model_summary = pd.DataFrame([c3_num_mets, c3_num_rxn, c3_num_transport_rxn, c3_num_export_rxn, c3_num_import_rxn],
index=['total metabolites','total reactions','transport reactions', 'export reactions' ,'import reactions'], columns=['Count'])
df_c3_model_summary
Code Cell 4: Load Metabolic Model of C3 Photosynthesis in Arabidposis thaliana
Count | |
---|---|
total metabolites | 413 |
total reactions | 572 |
transport reactions | 139 |
export reactions | 90 |
import reactions | 8 |
Import
display(HTML(f"<h1>Code Cell 5: Add Constraints on Metabolite Input</h1>"))
##### Add constraints on metabolite uptake #####
## CONSTRAINT: CO2 uptake rate in C3 plants is about 20 μmol/(m2*s)
f_c3_CO2 = 20 #[μmol/(m2*s)]
set_bounds('Im_CO2', (0, f_c3_CO2), c3_model)
## CONSTRAINT: max. photon consumption 1000 μE
f_c3_hnu = 1000 #[μE]
set_bounds('Im_hnu', (0, f_c3_hnu), c3_model)
## CONSTRAINT: Fluxes of other import reactions
set_bounds('Im_H2O', (-inf, inf), c3_model)
set_bounds('Im_H2S', (0.,0.), c3_model)
set_bounds('Im_NH4', (0., 0.), c3_model)
set_bounds('Im_NO3', (0., inf), c3_model)
set_bounds('Im_Pi', (0., inf), c3_model)
set_bounds('Im_SO4', (0., inf), c3_model)
set_bounds('Ex_O2', (-inf, inf), c3_model)
set_bounds('Ex_Suc', (0., inf), c3_model)
set_bounds('Ex_starch', (0., inf), c3_model)
set_bounds('Ex_AA', (0., inf), c3_model)
Code Cell 5: Add Constraints on Metabolite Input
As in 2Arnold and
Nikoloski2014, we assume photoautotrophic growth
conditions. Only the import of light, water, CO2, inorganic phosphate (),
nitrate/ammonium, and sulphates/hydrogen sulphide is allowed, compare Table 3. More specifically, we
do only allow for nitrate uptake, since it is the main source (80%) of nitrogen in leaves
45Macduff and
Bakken2003. The CO2 uptake is limited to
f_c3_CO2
null
Export
display(HTML(f"<h1>Code Cell 6: Add Constraints on Metabolite Output</h1>"))
##### Add constraints on metabolite secretion #####
## CONSTRAINT: Fluxes of other import reactions
set_bounds('Ex_O2', (-inf, inf), c3_model)
set_bounds('Ex_Suc', (0., inf), c3_model)
set_bounds('Ex_starch', (0., inf), c3_model)
set_bounds('Ex_AA', (0., inf), c3_model)
## CONSTRAINT: Output of sucrose : total amino acid
r_suc_aa = (2.2, 1.0)
const_c3_suc_aa = set_fixed_flux_ratio({'Ex_Suc':r_suc_aa[0],'Ex_AA':r_suc_aa[1]}, 'const_c3_suc_aa', c3_model)
## CONSTRAINT: Output of sucrose : starch
r_suc_starch = (1.0, 1.0)
const_c3_suc_starch = set_fixed_flux_ratio({'Ex_Suc':r_suc_starch[0],'Ex_starch':r_suc_starch[1]}, 'const_c3_suc_starch', c3_model)
Code Cell 6: Add Constraints on Metabolite Output
In contrast to 2Arnold and
Nikoloski2014, we focus on mature, fully
differentiated and photosynthetic active leaves supporting the growth of the plant through
the export of nutrients in the phloem sap, mainly sucrose and amino acids. An output
reaction for sucrose Ex_Suc is already included in the
model. An additional export reaction Ex_AA represents the relative
proportion of 18 amino acids in the phloem sap of Arabidopsis as stoichiometric
coefficients in accordance to experimentally measured data from 85Wilkinson and
Douglas2003. The ratio of exported sucrose : total
amino acid is estimated to be r_suc_aa[0]
r_suc_aa[1]
display(HTML(f"<h1>Code Cell 7: Create Table on Flux Boundary Constraints of Input and Output Reactions</h1>"))
#### Table 3 ####
#{
# "caption": "#### Flux boundary constraints of Im-/export reactions",
# "id": "table3",
# "label": "Table 3.",
# "trusted": true
#}
index = c3_model.reactions.query(lambda rxn: (rxn.id.startswith('Ex_') or rxn.id.startswith('Im_'))).list_attr('id')
lower_bounds = c3_model.reactions.query(lambda rxn: (rxn.id.startswith('Ex_') or rxn.id.startswith('Im_'))).list_attr('lower_bound')
upper_bounds = c3_model.reactions.query(lambda rxn: (rxn.id.startswith('Ex_') or rxn.id.startswith('Im_'))).list_attr('upper_bound')
cols = ['Lower bound [μmol/(m^2^s)]', 'Upper bound [μmol/(m^2^s)]' ]
df_ex_im_rxn_bounds = pd.DataFrame(np.array([lower_bounds, upper_bounds]).T,index=index, columns=cols)
df_ex_im_rxn_bounds.index.name = 'Reaction ID'
df_ex_im_rxn_bounds.style.applymap(lambda val: 'color: red' if val != 0 else 'color: black')
Code Cell 7: Create Table on Flux Boundary Constraints of Input and Output Reactions
Lower bound [μmol/(m^2^s)] | Upper bound [μmol/(m^2^s)] | |
---|---|---|
Reaction ID | ||
Im_hnu | 0 | 1000 |
Im_CO2 | 0 | 20 |
Im_H2O | -1000000 | 1000000 |
Im_Pi | 0 | 1000000 |
Im_NO3 | 0 | 1000000 |
Im_NH4 | 0 | 0 |
Im_SO4 | 0 | 1000000 |
Im_H2S | 0 | 0 |
Ex_O2 | -1000000 | 1000000 |
Ex_Ala_c | 0 | 0 |
Ex_Ala_h | 0 | 0 |
Ex_Ala_m | 0 | 0 |
Ex_Ala_p | 0 | 0 |
Ex_Arg_c | 0 | 0 |
Ex_Arg_h | 0 | 0 |
Ex_Arg_m | 0 | 0 |
Ex_Arg_p | 0 | 0 |
Ex_Asn_c | 0 | 0 |
Ex_Asn_h | 0 | 0 |
Ex_Asn_m | 0 | 0 |
Ex_Asn_p | 0 | 0 |
Ex_Asp_c | 0 | 0 |
Ex_Asp_h | 0 | 0 |
Ex_Asp_m | 0 | 0 |
Ex_Asp_p | 0 | 0 |
Ex_Cys_c | 0 | 0 |
Ex_Cys_h | 0 | 0 |
Ex_Cys_m | 0 | 0 |
Ex_Cys_p | 0 | 0 |
Ex_Gln_c | 0 | 0 |
Ex_Gln_h | 0 | 0 |
Ex_Gln_m | 0 | 0 |
Ex_Gln_p | 0 | 0 |
Ex_Glu_c | 0 | 0 |
Ex_Glu_h | 0 | 0 |
Ex_Glu_m | 0 | 0 |
Ex_Glu_p | 0 | 0 |
Ex_Gly_c | 0 | 0 |
Ex_Gly_h | 0 | 0 |
Ex_Gly_m | 0 | 0 |
Ex_Gly_p | 0 | 0 |
Ex_His_c | 0 | 0 |
Ex_His_h | 0 | 0 |
Ex_His_m | 0 | 0 |
Ex_His_p | 0 | 0 |
Ex_Ile_c | 0 | 0 |
Ex_Ile_h | 0 | 0 |
Ex_Ile_m | 0 | 0 |
Ex_Ile_p | 0 | 0 |
Ex_Leu_c | 0 | 0 |
Ex_Leu_h | 0 | 0 |
Ex_Leu_m | 0 | 0 |
Ex_Leu_p | 0 | 0 |
Ex_Lys_c | 0 | 0 |
Ex_Lys_h | 0 | 0 |
Ex_Lys_m | 0 | 0 |
Ex_Lys_p | 0 | 0 |
Ex_Met_c | 0 | 0 |
Ex_Met_h | 0 | 0 |
Ex_Met_m | 0 | 0 |
Ex_Met_p | 0 | 0 |
Ex_Phe_c | 0 | 0 |
Ex_Phe_h | 0 | 0 |
Ex_Phe_m | 0 | 0 |
Ex_Phe_p | 0 | 0 |
Ex_Pro_c | 0 | 0 |
Ex_Pro_h | 0 | 0 |
Ex_Pro_m | 0 | 0 |
Ex_Pro_p | 0 | 0 |
Ex_Ser_c | 0 | 0 |
Ex_Ser_h | 0 | 0 |
Ex_Ser_m | 0 | 0 |
Ex_Ser_p | 0 | 0 |
Ex_Thr_c | 0 | 0 |
Ex_Thr_h | 0 | 0 |
Ex_Thr_m | 0 | 0 |
Ex_Thr_p | 0 | 0 |
Ex_Trp_c | 0 | 0 |
Ex_Trp_h | 0 | 0 |
Ex_Trp_m | 0 | 0 |
Ex_Trp_p | 0 | 0 |
Ex_Tyr_c | 0 | 0 |
Ex_Tyr_h | 0 | 0 |
Ex_Tyr_m | 0 | 0 |
Ex_Tyr_p | 0 | 0 |
Ex_Val_c | 0 | 0 |
Ex_Val_h | 0 | 0 |
Ex_Val_m | 0 | 0 |
Ex_Val_p | 0 | 0 |
Ex_starch | 0 | 1000000 |
Ex_Glc | 0 | 0 |
Ex_Frc | 0 | 0 |
Ex_Suc | 0 | 1000000 |
Ex_cellulose | 0 | 0 |
Ex_Mas | 0 | 0 |
Ex_MACP | 0 | 0 |
Ex_Tre | 0 | 0 |
Ex_AA | 0 | 1000000 |
Flux boundary constraints of Im-/export reactions
Additional Constraints
display(HTML(f"<h1>Code Cell 8: Add Constraints on ATP maintenance costs</h1>"))
## CONSTRAINT: Maintenance cost
atp_cost_L3_m = 0.009111187245501572 #Mitochondria-L3-ATP Cost [µmol*s-1*m-2]
atp_cost_L3_h = 0.15270708327974447 #Chloroplast-L3-ATP Cost [µmol*s-1*m-2]
atp_cost_L3_p = 0.0076669066992201855 #Peroxisome-L3-ATP Cost [µmol*s-1*m-2]
atp_cost_L3_c = 0.042683072918274702 #Cytosl/Other-L3-ATP Cost [µmol*s-1*m-2]
set_fixed_flux('NGAM_c',atp_cost_L3_c + atp_cost_L3_p, c3_model)
set_fixed_flux('NGAM_m',atp_cost_L3_m, c3_model)
set_fixed_flux('NGAM_h',atp_cost_L3_h, c3_model)
Code Cell 8: Add Constraints on ATP maintenance costs
#### Table 4 ####
#{
# "caption": "#### Maintenance costs by compartment",
# "id": "table4",
# "label": "Table 4.",
# "trusted": true
#}
df_maintenance = pd.DataFrame([atp_cost_L3_c,atp_cost_L3_h, atp_cost_L3_m, atp_cost_L3_p], index=['cytosol','chloroplast','mitochondria','peroxisome'], columns=['Flux [μmol/(m^2^s)]'])
df_maintenance.index.name = 'Compartment'
df_maintenance
Flux [μmol/(m^2^s)] | |
---|---|
Compartment | |
cytosol | 0.042683 |
chloroplast | 0.152707 |
mitochondria | 0.009111 |
peroxisome | 0.007667 |
Maintenance costs by compartment
We explicitly include the maintenance costs in our model to cover the amounts of ATP that is used to degradation and re-synthesis proteins for each compartment. 40Li et al.2017 specifies the ATP costs for protein degradation and synthesis of each compartment of a mature Arabidopsis leaf. Based on the given data, we were able to calculate the flux rates to constrain the maintenance reactions in each compartment (Table 4).
null
The one-cell model contains maintenance reactions only for the cytsol (NGAM_c), chloroplast (NGAM_h) and mitochondria (NGAM_m) in the form:
An equivalent maintenance reaction cannot be formulated for the peroxisome since in the one-cell model ATP/ADP are not included as peroxisomal metabolites. The flux through the maintenance reactions is fixed to the determined maintenance costs given in Table 4. The peroxisomal maintenance costs are added to the cytosolic maintenance costs.
display(HTML(f"<h1>Code Cell 10: Add Constraint on Rubisco Oxygenation : Decarboxylation Ratio</h1>"))
## CONSTRAINT: oxygenation : decarboxylation = 1 : 10
r_c3_rbc_rbo = (10.0, 1.0)
const_c3_rbc_rbo = set_fixed_flux_ratio({'RBC_h':r_c3_rbc_rbo[0],'RBO_h':r_c3_rbc_rbo[1]}, 'const_c3_rbc_rbo', c3_model)
Code Cell 10: Add Constraint on Rubisco Oxygenation : Decarboxylation Ratio
The CO2 and O2 partial pressures determine the ratio of the oxygenation : carboxylation rate of Rubisco (given by reactions RBO_h and RBC_h) and can be described by the mathematical expression:
where specifies the ability of Rubisco to bind CO2 over O2. In the case of a mature leave and ambient CO2 and O2 partial pressures in temperate regions with adequate water supply, the ratio is fixed and is predicted to be {{int(r_c3_rbc_rbo0)}}:{{int(r_c3_rbc_rbo1)}}, which is encoded by an additional flux ratio constraint.
display(HTML(f"<h1>Code Cell 11: Add Constraint on NADPH dehydrogenase and plastoquinol oxidase</h1>"))
## CONSTRAINT: fluxes through the chloroplastic NADPH dehydrogenase and plastoquinol oxidase were set to zero
#because the contributions of NADPH dehydrogenase (Yamamoto et al., 2011) and plastoquinol oxidase
#(Josse et al., 2000) to photosynthesis are thought to be minor.
set_bounds('AOX4_h',(0,0), c3_model)
set_bounds('iCitDHNADP_h',(0,0), c3_model)
Code Cell 11: Add Constraint on NADPH dehydrogenase and plastoquinol oxidase
We assume no flux for the chloroplastic NADPH dehydrogenase (iCitDHNADP_h) and plastoquinol oxidase (AOX4_h) because 33Josse et al.2000 and 88Yamamoto et al.2011 have shown that their effect on the photosynthesis is minor.
display(HTML(f"<h1>Code Cell 12: Add additional Transport Constraints</h1>"))
## CONSTRAINT: NTT is only active at night
set_fixed_flux('Tr_NTT',0, c3_model)
## CONSTRAINT: No uncoupled pyruvate transport
set_bounds('Tr_Pyr1',(0,0), c3_model)
set_bounds('Tr_Pyr2',(0,0), c3_model)
## CONSTRAINT:
set_bounds('G6PDH_h', (0.,0.), c3_model)
set_bounds('PPIF6PK_c', (0,0.), c3_model)
Code Cell 12: Add additional Transport Constraints
Objective
display(HTML(f"<h1>Code Cell 13: Add Objective - Optimize Sucrose Output</h1>"))
## Optimize/Maximize sucrose output
r_c3_opt_id = "Ex_Suc"
r_c3_opt_obj = c3_model.reactions.get_by_id(r_c3_opt_id)
r_c3_opt_obj.objective_coefficient = 1.
Code Cell 13: Add Objective - Optimize Sucrose Output
In accordance with the assumption of mature, fully differentiated and photosynthetic active leaf, the model’s objective is to maximise the phloem sap output defined by reactions {{r_c3_opt_id}}. Additionally, we assume that the involved plant cells put only a minimal metabolic effort, in the form of energy and resources, into the production of phloem sap as possible. This assumption is in correspondence with minimising the nitrogen investment by reducing the number of enzymes that are active in a metabolic network. Therefore, we perform a parsimonious FBA to minimise the total flux.
For enhanced compliance with the recent standards of the systems biology community, the one-cell model is encoded in SBML level 3. Meta-information on subsystems, publications, cross-references are provided as evidence code in the form of MIRIAM URI’s. FBA related information, gene association rules, charge and formula of a species element are encoded using the Flux Balance Constraints package developed for SBML level 3. All fluxes in the model are consistently defined as μmol/(m2s).
Generic model for C4 metabolism
Metabolic model
display(HTML(f"<h1>Code Cell 14: Initiate Metabolic Model of C4 Photosynthesis</h1>"))
## Intitialize C4 model
c4_model = cobra.Model('c4_model')
## Define cell types
cell_types = ['M', 'B']
## Duplicate metabolites
for m in c3_model.metabolites:
for cell in cell_types:
m_dt = cobra.Metabolite('['+cell+']_'+m.id, name = m.formula, compartment = m.compartment)
c4_model.add_metabolites([m_dt])
## Duplicate reactions
for r_c3_obj in c3_model.reactions:
for cell in cell_types:
r_c4_obj = cobra.Reaction('['+cell+']_'+r_c3_obj.id)
r_c4_obj.name = r_c3_obj.name
r_c4_obj.subsystem = r_c3_obj.subsystem
r_c4_obj.bounds = r_c3_obj.bounds
c4_model.add_reaction(r_c4_obj)
r_c4_obj.add_metabolites({'['+cell+']_'+m_c3_obj.id: r_c3_obj.get_coefficient(m_c3_obj) for m_c3_obj in r_c3_obj.metabolites})
## Model Summary
c4_num_mets = len(c4_model.metabolites)
c4_num_rxn = len(c4_model.reactions)
df_c4_model_summary = pd.DataFrame([c4_num_mets, c4_num_rxn], index=['Number of metabolites','Number of reactions'], columns=['Count'])
df_c4_model_summary
Code Cell 14: Initiate Metabolic Model of C4 Photosynthesis
Count | |
---|---|
Number of metabolites | 826 |
Number of reactions | 1144 |
The generic model of C4 metabolism, short two-cell model, comprises two copies of the one-cell model to represent one mesophyll and one bundle sheath cell. Reactions and metabolites belonging to the metabolic network of the mesophyll are indicated with the prefix [M], whereas the prefix for the bundle sheath is [B]. The separate mesophyll and bundle sheath networks are connected via reversible transport reactions of the cytosolic metabolites indicated with the prefix [MB], Figure 2. The C4 evolution not only confined Rubisco to the bundle sheath cells, the CO2 concentrating mechanism steadily supplies Rubisco with CO2 in such a way that the oxygenation rate is negligible. Therefore, the bundle sheath network is equipped with two Rubisco populations. The native Rubisco population binds external CO2 and adheres to forced oxygenation : carboxylation ratios, where the optimised evolutionary population binds only internal CO2 and the carboxylation occurs independently of the oxygenation. External CO2 is defined as _[B]_CO2_ex_{_c,h} supplied by the mesophyll network. Internal CO2 given by _[B]_CO2_{_c,h,m} originates from reactions in the bundle sheath network producing CO2. External CO2 in the bundle sheath network is only allowed to move to the chloroplast [B]_Tr_CO2h_Ex and to react with Rubisco [B]_RBC_h_Ex. The differentiation of two Rubisco populations binding either external or internal CO2 approximates the concentration-dependent shift of the oxygenation : carboxylation ratio.
Imports
display(HTML(f"<h1>Code Cell 15: Adapt CO2 Input Constraint</h1>"))
## CONSTRAINT: CO2 uptake rate uin C4 plants is higher, about 40 μmol/(m2*s)
f_C4_CO2_M = 40 #[μmol/(m2*s)]
set_bounds('[M]_Im_CO2', (0, f_C4_CO2_M), c4_model)
## CONSTRAINT: No CO2 uptake in bundle sheat cells due to suberin layer in cell membranes
f_C4_CO2_B = 0 #[μmol/(m2*s)]
set_fixed_flux('[B]_Im_CO2', f_C4_CO2_B, c4_model)
## Other constraints on inputs are directly transfered from the c3 model
Code Cell 15: Adapt CO2 Input Constraint
As for the one-cell model, we assume photoautotrophic growth conditions, see Table 3. During C4 evolution the CO2 assimilation became more efficient allowing higher CO2 assimilation rates. Zea mays achieves up to {{f_C4_CO2_M}} μmol/(m2s) ([M]_Im_CO2) 60Rozema1993. We assume that the CO2 uptake from the environment by the bundle sheath has to be bridged by the mesophyll. Therefore, the input flux of [B]_Im_CO2 is set to {{f_C4_CO2_B}}.
Exports
display(HTML(f"<h1>Code Cell 16: Adapt Output Constraints</h1>"))
## CONSTRAINT: Output of sucrose : total amino acid and sucrose : starch
r_suc_aa = (2.2, 1.0)
const_c4_suc_aa_b = set_fixed_flux_ratio({'[B]_Ex_Suc':r_suc_aa[0],'[B]_Ex_AA':r_suc_aa[1]}, 'const_c4_suc_aa_b', c4_model)
const_c4_suc_aa_m = set_fixed_flux_ratio({'[M]_Ex_Suc':r_suc_aa[0],'[M]_Ex_AA':r_suc_aa[1]}, 'const_c4_suc_aa_m', c4_model)
r_suc_starch = (1.0, 1.0)
const_c4_suc_starch_b = set_fixed_flux_ratio({'[B]_Ex_Suc':r_suc_starch[0],'[B]_Ex_starch':r_suc_starch[1]}, 'const_c4_suc_starch_b', c4_model)
const_c4_suc_starch_m = set_fixed_flux_ratio({'[M]_Ex_Suc':r_suc_starch[0],'[M]_Ex_starch':r_suc_starch[1]}, 'const_c4_suc_starch_m', c4_model)
## Other constraints on outputs are directly transfered from the c4 model
Code Cell 16: Adapt Output Constraints
The outputs of the one-cell model are transferred to the mesophyll and bundle sheath network, as well as the corresponding flux ratios, see Table 3.
Additional Constraints
display(HTML(f"<h1>Code Cell 17: Add Metabolite Exchange Reactions</h1>"))
## Metabolites excluded from M/BS exchange
no_transport = ['NO3','NO2', 'O2','Na', 'H2S', 'SO4',
'H2O','FBP','F26BP','DPGA','H','ACD','AC','M_DASH_THF', '5M_DASH_THF', 'H_DASH_Cys', 'aH_DASH_Cys', 'ORO', 'DHO',
'GABA','A_DASH_Ser','PRPP','AD','THF','DHF','ADN','Mas','CoA','GluP',
'A_DASH_CoA','cellulose1','cellulose2','cellulose3','starch1',
'starch2','starch3','TRXox','TRXrd','Glu_DASH_SeA','T6P','aMet',
'PPi', 'P5C', 'NH4', 'Pi', 'CO2', 'OAA','HCO3',
'UTP', 'UDP', 'UDPG', 'ATP', 'ADP', 'AMP', 'IMP', 'XMP',
'GTP', 'GDP', 'GMP', 'OMP', 'UMP', 'CTP', 'GDP', 'CDP', 'dADP',
'dCDP', 'dGDP', 'dUDP', 'dUTP', 'dUMP', 'dTMP', 'dTDP', 'GTP',
'dATP', 'dCTP', 'dGTP', 'dTTP', 'NAD', 'NADH', 'NADP', 'NADPH']
## dd M/BS exchange reactions
L_r_transport = []
for m_c3_obj in c3_model.metabolites:
if m_c3_obj.id[-1:] == 'c' and m_c3_obj.id[:-2] not in no_transport:
r_c4_obj = cobra.Reaction('[MB]_'+m_c3_obj.id)
r_c4_obj.name = '[MB]_'+m_c3_obj.id
r_c4_obj.subsystem = 'Exchange'
r_c4_obj.bounds = (-inf, inf)
c4_model.add_reaction(r_c4_obj)
r_c4_obj.add_metabolites({'[M]_'+m_c3_obj.id: -1,'[B]_'+m_c3_obj.id: 1 })
L_r_transport.append('[MB]_'+m_c3_obj.id)
## Model Summary
c4_num_mets = len(c4_model.metabolites)
c4_num_rxn = len(c4_model.reactions)
df_c4_model_summary = pd.DataFrame([c4_num_mets, c4_num_rxn], index=['Number of metabolites','Number of reactions'], columns=['Count'])
df_c4_model_summary
Code Cell 17: Add Metabolite Exchange Reactions
Count | |
---|---|
Number of metabolites | 826 |
Number of reactions | 1188 |
The mesophyll and bundle sheath networks are connected by a range of cytosolic transport metabolites including amino acids, sugars (glucose, fructose, sucrose, trehalose, ribose), single phosphorylated sugar (glucose-6-phosphate, glucose-1-phosphate, fructose-6-phosphate, sucrose-6-phosphate), mono-/di-/tri-carboxylic acids (phosphoenolpyruvate, pyruvate, citrate, cis-aconitate, isocitrate, -ketoglutarate, succinate, fumarate, malate), glyceric acids (2-Phosphoglycerate, 3-Phosphoglycerate), glycolate, glycerate, glyceraldehyde-3-phosphate, di-hydroxyacetone-phosphate and CO2. Nucleotides, NAD/NADH, NADP/NADPH, pyrophosphate, inorganic phosphate are not considered as transport metabolites. Oxaloacetate has been excluded as transport metabolite since concentrations of oxaloacetate are very low in vivo and it is reasonably unstable in aqueous solutions. Other small molecules that can be imported by the bundle sheath from the environment, as well as protons and HCO3-, are not exchanged between the two cell types.
display(HTML(f"<h1>Code Cell 18: Add Constraints for CO2 Uptake in BS Cells and Rubisco Carboxylation : Oxygenation Ratio</h1>"))
## CONSTRAINT: Add external CO2 species to bundle sheath
#(the original CO2 species is treated as internal CO2)
m_list_CO_Ex= ['[B]_CO2_ex_c','[B]_CO2_ex_h']
for m_id in m_list_CO_Ex:
m_obj = cobra.Metabolite(m_id)
c4_model.add_metabolites(m_obj)
## CONSTRAINT: Copy reactions 'Tr_CO2h', 'RBC_h' and replace internal CO2 with external CO2 in the copied reactions
r_list_CO_Ex = ['Tr_CO2h', 'RBC_h']
for r_id in r_list_CO_Ex:
r_obj = c4_model.reactions.get_by_id('[B]_'+r_id)
r_obj_Ex = cobra.Reaction(r_obj.id+'_Ex')
r_obj_Ex.name = r_obj.id+'_Ex'
r_obj_Ex.subsystem = r_obj.subsystem
r_obj_Ex.bounds = r_obj.bounds
c4_model.add_reaction(r_obj_Ex)
r_obj_Ex.add_metabolites({m_obj.id if not m_obj.id[:-2] == '[B]_CO2' else '[B]_CO2_ex'+m_obj.id[-2:]: r_obj.get_coefficient(m_obj)
for m_obj in r_obj.metabolites})
## CONSTRAINT: CO2 exchange between mesophyll and bundle sheat
r_c4_obj = cobra.Reaction('[MB]_CO2_c')
r_c4_obj.name = '[MB]_CO2_c'
r_c4_obj.subsystem = 'Exchange'
r_c4_obj.bounds = (-inf, inf)
c4_model.add_reaction(r_c4_obj)
r_c4_obj.add_metabolites({'[M]_CO2_c': -1,'[B]_CO2_ex_c': 1 })
L_r_transport.append('[MB]_CO2_c')
## CONSTRAINT: oxygenation : carboxylation = 1 : 3
r_c4_rbc_rbo = (3.0, 1.0)
const_c4_rbc_rbo_b = set_fixed_flux_ratio({'[B]_RBC_h_Ex':r_c4_rbc_rbo[0],'[B]_RBO_h':r_c4_rbc_rbo[1]}, 'const_c4_rbc_rbo_b', c4_model)
const_c4_rbc_rbo_m = set_fixed_flux_ratio({'[M]_RBC_h':r_c4_rbc_rbo[0],'[M]_RBO_h':r_c4_rbc_rbo[1]}, 'const_c4_rbc_rbo_m', c4_model)
#Model Summary
c4_num_mets = len(c4_model.metabolites)
c4_num_rxn = len(c4_model.reactions)
df_c4_model_summary = pd.DataFrame([c4_num_mets, c4_num_rxn], index=['Number of metabolites','Number of reactions'], columns=['Count'])
df_c4_model_summary
Code Cell 18: Add Constraints for CO2 Uptake in BS Cells and Rubisco Carboxylation : Oxygenation Ratio
Count | |
---|---|
Number of metabolites | 828 |
Number of reactions | 1191 |
The ATP costs for cell maintenance in the genC3 model are assigned to both cell types in the two-cell model. Due to declining CO2 concentrations over evolutionary time and/or adverse conditions which close the stromata, the oxygenation : carboxylation ratio of the native Rubisco population in the bundle sheath and the mesophyll is increased and can be predicted as {{r_c4_rbc_rbo0}} : {{r_c4_rbc_rbo1}}, the corresponding flux ratios are adapted accordingly.
display(HTML(f"<h1>Code Cell 19: Add Constraint on Photon Uptake</h1>"))
## Reaction variables for light uptake
B_Im_hnu = c4_model.reactions.get_by_id("[B]_Im_hnu")
M_Im_hnu = c4_model.reactions.get_by_id("[M]_Im_hnu")
## CONSTRAINT: Total Photon uptake limited to 1000 µE
f_c4_hnu_ub = 1000 #[μE]
f_c4_hnu_lb = 0 #[μE]
const_hnu_sum = c4_model.problem.Constraint(
B_Im_hnu.flux_expression + M_Im_hnu.flux_expression,
lb = f_c4_hnu_lb,
ub = f_c4_hnu_ub,
name = 'const_hnu_sum',
)
c4_model.add_cons_vars(const_hnu_sum)
## CONSTRAINT: Total Photon uptake by bundle sheath must be less equal than in mesophyll
const_hnu_ratio = c4_model.problem.Constraint(
M_Im_hnu.flux_expression - B_Im_hnu.flux_expression,
lb = f_c4_hnu_lb,
ub = f_c4_hnu_ub,
name = 'const_hnu_ratio')
c4_model.add_cons_vars(const_hnu_ratio)
Code Cell 19: Add Constraint on Photon Uptake
Furthermore, we assume that the total photon uptake in the mesophyll and bundle sheath is in the range of {{f_c4_hnu_lb}} μmol/(m2s) to {{f_c4_hnu_lb}} μmol/(m2s). Since they are more central in the leaf, the photon uptake by the bundle sheath must be equal or less compared to the mesophyll.
Objective
display(HTML(f"<h1>Code Cell 20: Add Objective to Optimize Sucrose Output</h1>"))
## Optimize/Maximize sucrose output
r_opt_id = "[B]_Ex_Suc"
r_opt_obj = c4_model.reactions.get_by_id(r_opt_id)
r_opt_obj.objective_coefficient = 1.
Code Cell 20: Add Objective to Optimize Sucrose Output
The maximisation of the phloem sap output through the bundle sheath and the minimisation of the metabolic effort are kept as objectives in the two-cell model.
Results
The curated Arabidopsis core model predicts physiological results
Flux balance analysis requires five types of information, the metabolic map of the organism, the input, the output, a set of constraints (i.e. limitations on input, directionality of reactions, forced flux through reactions), and optimisation criteria for the algorithm which approximate the selective pressures the metabolism evolved under. In this context, inputs define the resources that need to be taken up by the metabolic network to fulfil a particular metabolic function, which is related to the outputs, for example the synthesis of metabolites part of the biomass or other specific products. In CBM, the objective is most likely related to the in- and/or outputs.
For reconstruction of the C3 metabolic map we curated the Arabidopsis core model 2Arnold and Nikoloski2014 manually (Table 1) to represent the metabolism of a mesophyll cell in a mature photosynthetically active leaf of a C3 plant , further on called one-cell model (provided in Figure 1—source data 1). The Arabidopsis core model is a bottom-up-assembled, large-scale model relying solely on Arabidopsis-specific annotations and the inclusion of only manually curated reactions of the primary metabolism. The Arabidopsis core model is accurate with respect to mass and energy conservation, allowing optimal nutrient utilisation and biochemically sound predictions 2Arnold and Nikoloski2014.
Arabidopsis core model | Observation | one-cell model | Reference |
---|---|---|---|
NADP-dependent malate dehydrogenases in all compartments | cycles through nitrate reductase to interconvert NAD and NADP | NAD-dependent malate dehydrogenases in all compartments, NADP-dependent malate dehydrogenase only in chloroplast | (76Swarbreck et al.2008) |
Cyclic electron flow | absence of cyclic electron flow | added | (70Shikanai2016) |
Alternative oxidase | missing alternative routes for electrons to pass the electron transport chain to reduce oxygen | added alternative oxidase reactions to the chloroplast and mitochondria | (81Vishwakarma et al.2015) |
Alanine transferase | No alanine transferase in cytosol Alanine transferase | added | (41Liepman and Olsen2003) |
Transport chloroplast | no maltose transporter by MEX1 | added | (42Linka and Weber2010) |
no glucose transporter by MEX1 and pGlcT MEX1 | added | ||
no unidirectional transport of ATP, ADP, AMP by BT-like | added | ||
no Mal/OAA, Mal/Pyr, and Mal/Glu exchange by DiTs | added | ||
no folate transporter by FBT and FOLT1 | added | ||
Transport Mitochondria | no Mal/OAA, Cit/iCit, Mal/KG exchange by DTC | added | (42Linka and Weber2010) |
no H+ importer by UCPs import | added | ||
no OAA/Pi exchange by DIC1-3 | added | ||
no ATP/Pi exchange by APCs | added | ||
no NAD/ADP and NAD/AMP exchange by NDT2 | added | ||
no ThPP/ATP exchange by TPCs | added | ||
no Asp/Glu by AGCs | added | ||
no uncoupled Ala exchange | added | ||
Transport peroxisome | missing NAD/NADH, NAD/ADP, NAD/AMP exchange by PXN | added | (42Linka and Weber2010) |
no ATP/ADP and ATP/AMP exchange by PNCs | added | ||
H+ sinks/sources | H+ sinks/source reaction for the cytosol and futile transport cycles introduced by H+ -coupled transport reactions | H+ sinks/source reaction added for each compartment | |
ATPase stoichiometry | False H+/ATP ratios for the plastidal and mitochondrial ATP synthase | H+/ATP ratio set to 3 : 1 (chloroplast) and 4:1 (mitochondria) | (56Petersen et al.2012; 79Turina et al.2016) |
Alanine/aspartate transferase | no direct conversion of alanine and aspartate | added to cytosol, chloroplast and mitochondria | (69Schultz and Coruzzi1995; 22Duff et al.2012) |
For the inputs, we considered a photoautotrophic growth scenario with a fixed CO2 uptake of about {{f_c3_CO2}} μmol/(m2s) 37Lacher2003. Light, sulphates, and phosphate are freely available. Due to the observation that nitrate is the main source (80%) of nitrogen in leaves in many species 45Macduff and Bakken2003, we set nitrate as the sole nitrogen source. If both ammonia and nitrate are allowed, the model will inevitably predict the physiologically incorrect sole use of ammonia since fewer reactions and less energy are required to convert it into glutamate, the universal amino group currency in plants. Water and oxygen can be freely exchanged with the environment in both directions.
To compute the output, we assume a mature fully differentiated and photosynthetically active leaf, which is optimised for the synthesis and export of sucrose and amino acids to the phloem under minimal metabolic effort. Following the examples of models in bacteria, many plant models use a biomass function which assumes that the leaf is required to build itself 19de Oliveira Dal'Molin et al.20102Arnold and Nikoloski201464Saha et al.2011 using photoautotrophic that is 2Arnold and Nikoloski2014 or heterotrophic that is 17Cheung et al.2014 energy and molecule supply. In plants, however, leaves transition from a sink phase in which they build themselves from metabolites delivered by the phloem to a source phase in which they produce metabolites for other organs including sink leaves 78Turgeon1989. The composition of Arabidopsis phloem exudate 85Wilkinson and Douglas2003 was used to constrain the relative proportions of the 18 amino acids and the ratio of sucrose : total amino acids (rsuc_aa0 : r_suc_aa1). To account for daily carbon storage as starch for export during the night, we assume that half of the assimilated carbon is stored in the _one-cell model. We explicitly account for maintenance costs by the use of a generic ATPase and use the measured ATP costs for protein degradation and synthesis of a mature Arabidopsis leaf 40Li et al.2017 as a constraint. We initially assume a low photorespiratory flux according to the ambient CO2 and O2 partial pressures considering no heat, drought, salt or osmotic stress which may alter the ratio towards higher flux towards the oxygenation reaction.
To develop a largely unconstrained model and detect possible errors in the metabolic map, we initially kept the model unconstrained with regard to fixed fluxes, flux ratios, and reaction directions. Different model iterations were run in (re-)design, simulate, validate cycles against known physiology with errors sequentially eliminated and a minimal set of constraints required for a C3 model recapitulating extant plant metabolism determined. After each change, the CBM predicted all fluxes which were output as a table and manually examined (for example see Figure 1—source data 2).
The initial FBA resulted in carbon fixation by enzymes such as the malic enzymes which, in reality, are constrained by the kinetics of the enzymes towards decarboxylation. All decarboxylation reactions were made unidirectional towards decarboxylation to prevent erroneous carbon fixation in the flux distribution. The next iteration of FBA predicted loops through nitrate reductases which ultimately converted NADH to NADPH. We traced this loop to an error in the initial model, in which malate dehydrogenases in the cytosol and mitochondrion were NADP-dependent instead of NAD-dependent. After correction of the co-factor in the one-cell model, the loops through nitrate reductases were no longer observed. Another iteration predicted excessive flux through the mitochondrial membrane where multiple metabolites were exchanged and identified missing transport processes as the likely reason. Based on 42Linka and Weber2010, we added known fluxes across the mitochondrial and plastidic envelope membranes which remedied the excessive fluxes in the solution. The chloroplastic ADP/ATP carrier protein is constrained to zero flux since its mutant is only affected during the night but not if light is available 59Reiser et al.2004.
The obtained flux distribution still contained excessive fluxes through multiple transport proteins across internal membranes which ultimately transferred protons between the organelles and the cytosol. Since for most if not all transport proteins the precise protonation state of metabolites during transport is unknown and hence cannot be correctly integrated into the model, we allowed protons to appear and disappear as needed in all compartments. This provision precludes conclusions about the energetics of membrane transport. ATP generation occurred in a distorted way distributed across different organelles which were traced to the H+ consumption of the ATPases in mitochondria and chloroplasts. The stoichiometry was altered to to 3:1 (chloroplast) and 4:1 (mitochondria) 56Petersen et al.201279Turina et al.2016. We assume no flux for the chloroplastic NADPH dehydrogenase and plastoquinol oxidase because 33Josse et al.200088Yamamoto et al.2011 have shown that their effect on photosynthesis is minor.
In preparation for modelling the C4 cycle, we ensured that all reactions known to occur in C4 (i.e. malate/pyruvate exchange, likely via DiT2 in maize 84Weissmann et al.2016, possibly promiscuous amino transferases 22Duff et al.2012) are present in the one-cell model, since 4Aubry et al.2011 showed that all genes encoding enzymes and transporters underlying the C4 metabolism are already present in the genome of C3 plants. We integrated cyclic electron flow 70Shikanai2016 and alternative oxidases in the mitochondria 81Vishwakarma et al.2015, since both have been hypothesised to be important during the evolution and/or execution of the C4 cycle. Models and analysis workflows provided as jupyter notebooks 77Thomas et al.2016 are available as supplementary material or can be accessed on GitHub https://github.com/ma-blaetke/CBM_C3_C4_Metabolism (10Blätke2019; copy archived at https://github.com/elifesciences-publications/CBM_C3_C4_Metabolism).
The one-cell model comprises in total {{c3_num_mets}} metabolites and {{c3_num_rxn}} reactions, whereof {{c3_num_transport_rxn}} are internal transporters, {{c3_num_export_rxn}} are export and {{c3_num_import_rxn}} import reactions (see also below), which are involved in 59 subsystems. Figure 1 provides an overview of the primary subsystems according to 2Arnold and Nikoloski2014.

Schematic representation of the primary subsystems in the one-cell model and the used input/output constraints; adapted from 2Arnold and Nikoloski2014.
######################################################################################################################################
######################################################################################################################################
############## EXPERIMENT 1: Effect of CO2 Uptake ##############
######################################################################################################################################
######################################################################################################################################
display(HTML(f"<h1>Code Cell 21: Experiment 1 -- Effect of CO2 Uptake </h1>"))
#Create copy of c3 model
c3_model_exp1 = c3_model.copy()
#Optimize/Maximize sucrose output
result_exp1_1_fba = c3_model_exp1.optimize('maximize') #perform FBA
#Optimize/Minimize total flux
if result_exp1_1_fba.status == 'optimal':
result_exp1_1_pfba = cobra.flux_analysis.parsimonious.pfba(c3_model_exp1)
#Fetch flux for CO2 uptake
v_co2_exp1 = result_exp1_1_pfba.fluxes['Im_CO2']
#Array defining proprtion of CO2 uptake
co2_ratios_exp1 = np.linspace(0,1,21)
df_result_exp1 = pd.DataFrame()
#Iterate over proportions of CO2 uptake
for co2_ratio in tqdm(co2_ratios_exp1):
#Fix upper flux bound for photon uptake
set_bounds('Im_CO2', (0, v_co2_exp1 * co2_ratio), c3_model_exp1)
#Optimize/Maximize sucrose output
result_exp1_2_fba = c3_model_exp1.optimize('maximize') #perform FBA
#Optimize/Minimize total flux
if result_exp1_2_fba.status == 'optimal': # check if feasible
result_exp1_2_pfba = cobra.flux_analysis.parsimonious.pfba(c3_model_exp1) #perform pFBA
if result_exp1_2_pfba.status == 'optimal':
df_result_exp1[v_co2_exp1 * co2_ratio] = result_exp1_2_pfba.fluxes
Code Cell 21: Experiment 1 -- Effect of CO2 Uptake
0%| | 0/21 [00:00<?, ?it/s]
######################################################################
############## Figure 1—figure supplement 1 ##############
######################################################################
#{
# "caption": "#### Effect of CO~2. Dependence of the phloem output on CO~2~ input flux in the range 0 μmol/(m^2^s)–20 μmol/(m^2^s). Sucrose and starch are produced in the same amounts, each of them consists of 12 C-atoms.",
# "id": "fig1s1",
# "label": "Figure 1—figure supplement 1",
# "trusted": true
#}
#Define reactions of interest by id
r_ids_exp1 = ['Ex_Suc','Ex_AA']
#Create figure
fig_exp1 = go.Figure()
#Add traces for reactions of interest
for r_id in r_ids_exp1:
#Create trace
trace = go.Scatter(
y = df_result_exp1.loc[r_id,:],
x = df_result_exp1.columns,
name = r_id,
mode = 'lines+markers',
)
#Add trace
fig_exp1.add_trace(trace)
#Update xaxes
fig_exp1.update_xaxes(
title = dict(
text = 'CO\u2082 Uptake [µmol/(m\u00B2s)]',
font = dict(size=18)
),
tickfont = dict(size=16)
)
#Update yaxes
fig_exp1.update_yaxes(
title = dict(
text = 'Flux [µmol/(m\u00B2s)]',
font = dict(size=18)
),
tickfont = dict(size=16)
)
#Update layout
fig_exp1.update_layout(
width=1000,
height=500,
title = dict(
text='<b>Phloem Export</b>',
x=0.5,
font=dict(size=20)
),
legend=dict(
font=dict(size=18),
)
)
#Show figure
fig_exp1.show()
Effect of CO2. Dependence of the phloem output on CO2~ input flux in the range 0 μmol/(m2s)–20 μmol/(m2s). Sucrose and starch are produced in the same amounts, each of them consists of 12 C-atoms.
######################################################################################################################################
######################################################################################################################################
############## EXPERIMENT 2: Effect of PPFD ##############
######################################################################################################################################
######################################################################################################################################
display(HTML(f"<h1>Code Cell 23: Experiment 2 -- Effect of PPFD </h1>"))
#Create copy of c3 model
c3_model_exp2 = c3_model.copy()
#Optimize/Maximize sucrose output
result_exp2_1_fba = c3_model_exp2.optimize('maximize') #perform FBA
#Optimize/Minimize total flux
if result_exp2_1_fba.status == 'optimal':
result_exp2_1_pfba = cobra.flux_analysis.parsimonious.pfba(c3_model_exp2)
#Fetch flux for photon uptake
v_hnu_exp2 = result_exp2_1_pfba.fluxes['Im_hnu']
#Array defining proprtion of photon uptake
hnu_ratios_exp2 = np.linspace(0,2,21)
df_result_exp2 = pd.DataFrame()
#Iterate over proportions of photon uptake
for hnu_ratio in tqdm(hnu_ratios_exp2):
#Fix upper flux bound for photon uptake
set_bounds('Im_hnu', (v_hnu_exp2 * hnu_ratio, v_hnu_exp2 * hnu_ratio), c3_model_exp2)
#Optimize/Maximize sucrose output
result_exp2_2_fba = c3_model_exp2.optimize('maximize') #perform FBA
#Optimize/Minimize total flux
if result_exp2_2_fba.status == 'optimal': # check if feasible
result_exp2_2_pfba = cobra.flux_analysis.parsimonious.pfba(c3_model_exp2) #perform pFBA
if result_exp2_2_pfba.status == 'optimal':
df_result_exp2[v_hnu_exp2 * hnu_ratio] = result_exp2_2_pfba.fluxes
Code Cell 23: Experiment 2 -- Effect of PPFD
0%| | 0/21 [00:00<?, ?it/s]
/Users/blaetke/opt/anaconda3/envs/elife-49305-era/lib/python3.9/site-packages/cobra/util/solver.py:508: UserWarning:
Solver status is 'infeasible'.
######################################################################
############## Figure 1—figure supplement 2 ##############
######################################################################
#{
# "caption": "#### PPFD variation. Dependence of phloem output on the PPFD in the range 0 μmol/(m^2^s)–400 μmol/(m^2^s). Sucrose and starch are produced in the same amounts, each of them consists of 12 C-atoms.",
# "id": "fig1s2,
# "label": "Figure 1—figure supplement 2",
# "trusted": true
#}
#Define reactions of interest by id
r_ids_exp2 = ['Ex_Suc','Ex_AA']
#Create figure
fig_exp2 = go.Figure()
#Add traces for reactions of interest
for r_id in r_ids_exp2:
#Create trace
trace = go.Scatter(
y = df_result_exp2.loc[r_id,:],
x = df_result_exp2.columns,
name = r_id,
mode = 'lines+markers',
)
#Add trace
fig_exp2.add_trace(trace)
#Update xaxes
fig_exp2.update_xaxes(
title = dict(
text = '<b>PPFD [µE]</b>',
font = dict(size=18)
),
tickfont = dict(size=16)
)
#Update yaxes
fig_exp2.update_yaxes(
title = dict(
text = 'Flux [µmol/(m\u00B2s)]',
font = dict(size=18)
),
tickfont = dict(size=16)
)
#Update layout
fig_exp2.update_layout(
width=1000,
height=500,
title = dict(
text='Phloem Export',
x=0.5,
font=dict(size=20)
),
legend=dict(
font=dict(size=18),
)
)
#Show figure
fig_exp2.show()
PPFD variation. Dependence of phloem output on the PPFD in the range 0 μmol/(m2s)–400 μmol/(m2s). Sucrose and starch are produced in the same amounts, each of them consists of 12 C-atoms.
null
######################################################################################################################################
######################################################################################################################################
############## EXPERIMENT 3: Simulate C3 Fluxes ##############
######################################################################################################################################
######################################################################################################################################
display(HTML(f"<h1>Code Cell 25: Experiment 3 -- Simulate C3 Fluxes </h1>"))
#Create copy of c3 model
c3_model_exp3 = c3_model.copy()
#Optimize/Maximize sucrose output
result_exp3_fba = c3_model_exp3.optimize('maximize')
#Optimize/Minimize total flux
if result_exp3_fba.status == 'optimal':
result_exp3_pfba = cobra.flux_analysis.parsimonious.pfba(c3_model_exp3)
#Load GO Term Database
goDB = obo_parser.GODag('elife-49305.ipython.src/go_basic.obo')
def get_go_term(go_ids):
if isinstance(go_ids, list):
go_terms = [goDB[go_id].name for go_id in go_ids]
else:
go_terms= [goDB[go_ids].name]
return go_terms
#Filter all biochemical reactions
c3_biochem_rxn = c3_model_exp3.reactions.query(lambda x: ~x.id.startswith('Tr') and ~x.id.startswith('Ex') and ~x.id.startswith('Im'))
#Grab annotation provided for the biochemical reactions, keep only GO IDs
df_anno = pd.DataFrame(
c3_biochem_rxn.list_attr('annotation'),
index=c3_biochem_rxn.list_attr('id')
).drop(['doi','ec-code','kegg.reaction','pubmed','isbn'], axis=1)
#Get GO Terms of GO IDs
df_anno['go term'] = df_anno['go'].apply(lambda go_ids: get_go_term(go_ids))
#Create Dataframe mapping GO IDs to biochemical reactions
df_go_term = pd.DataFrame(False,index=df_anno.index, columns=set(df_anno['go term'].sum()))
for r_id in df_anno.index:
df_go_term.loc[r_id,df_anno.loc[r_id,'go term'][0]] = True
#Define metabolites of interest (here energy equivalents)
met_classes = ['ATP','NADH', 'NADPH']
#Set up list to store Go terms
go_terms = []
#Search for all GO terms related to the reactions the metabolites are involved in
for met_class in met_classes:
#Find the specific metabolite ids in all compartments
met_ids = c3_model_exp3.metabolites.query(lambda x: x.id.startswith(met_class)).list_attr('id')
#Find reactions reactions and assigned GO Terms for all the metabolites
for met_id in met_ids:
#Get reaction ids
rxn_ids = [r_obj.id for r_obj in c3_model_exp3.metabolites.get_by_id(met_id).reactions]
#Get & collect GO Terms
go_terms += df_anno.loc[df_anno.index.intersection(rxn_ids),'go term'].sum()
#Create final list of all unique GO Terms
go_terms = list(set(go_terms))
go_terms = sorted(go_terms) + ['Others']
Code Cell 25: Experiment 3 -- Simulate C3 Fluxes
elife-49305.ipython.src/go_basic.obo: fmt(1.2) rel(2017-10-20) 47,002 GO Terms
##############################################################################
############## Figure 1—figure supplement 3 ##############
##############################################################################
#{
# "caption": "### Energy Flux Distribution in the _one-cell_ Model. (**A**) ATP production and consumption, (**B**) NADPH production and consumption, (**C**) NADH production and consumption.",
# "id": "fig1s3",
# "label": "Figure 1—figure supplement 3",
# "trusted": true
#}
def get_flux_by_go_term(df_rxn_go_term, rxn_fluxes):
'''
Return flux sum for go terms
Parameters:
rxn_fluxes (dict): dictionary of reactions and their flux values
df_rxn_go_term (dataframe): dataframe relating reactions and go terms
Returns:
df_rxn_go_term_flux (dataframe): go term related to prodcution of a specified energy equivalent and flux sum
'''
#Convert dictionary of reaction fluxes into series
s_rxn_fluxes = pd.Series(rxn_fluxes)
#Get flux sum
flux_sum = s_rxn_fluxes.sum()
#Determine flux sum for go terms
s_rxn_fluxes = s_rxn_fluxes[s_rxn_fluxes / flux_sum > 0.01]
df_rxn_go_term = df_rxn_go_term.loc[s_rxn_fluxes.index, df_rxn_go_term.loc[s_rxn_fluxes.index,:].sum() > 0]
df_rxn_go_term_flux = df_rxn_go_term.mul(s_rxn_fluxes[s_rxn_fluxes.index.intersection(df_rxn_go_term.index)],axis=0).sum()
df_rxn_go_term_flux['Others'] = flux_sum - df_rxn_go_term_flux.sum()
return df_rxn_go_term_flux
def prod_cons_charts(met_id, rxn_fluxes_prod, rxn_fluxes_cons, go_terms, df_rxn_go_term):
'''
Creates figure with two pie charts for production and consumption of a specified energy equivalent,
and returns two lists of go terms related to the production and consumption
Parameters:
met_id (int): metabolite id of the energy equivalent
rxn_fluxes_prod (dict): dictionary of reactions producing the energy equivalents and their flux values
rxn_fluxes_cons (dict): dictionary of reactions consuming the energy equivalents and their flux values
go_terms (list): list of all go terms
df_rxn_go_term (dataframe): dataframe relating reactions and go terms
Returns:
trace_prod (plotly trace): pie chart of go term related to prodcution
go_term_fluxes_prod (dataframe): go term related to prodcution of a specified energy equivalent and flux sum
trace_cons (plotly trace): pie chart of go term related to consumption
go_term_fluxes_cons (dataframe): go term related to consumption of a specified energy equivalent and flux sum
'''
#Get GO terms for production and consuption of energy equivalent
go_term_fluxes_prod = get_flux_by_go_term(df_rxn_go_term, rxn_fluxes_prod)
go_term_fluxes_cons = get_flux_by_go_term(df_rxn_go_term, rxn_fluxes_cons)
#Create index of GO terms for production and consuption of energy equivalent
go_term_index = go_term_fluxes_prod.index.union(go_term_fluxes_cons.index)
#Create trace for GO terms of energy equivalent production
trace_prod = go.Pie(
labels = go_term_fluxes_prod.index,
values = go_term_fluxes_prod,
marker=dict(line=dict(color='#FFF', width=1)),
)
#Create trace for GO terms of energy equivalent consumption
trace_cons = go.Pie(
labels = go_term_fluxes_cons.index,
values = go_term_fluxes_cons,
marker=dict(line=dict(color='#FFF', width=1)),
)
return trace_prod, go_term_fluxes_prod, trace_cons, go_term_fluxes_cons
#Set up Dataframe to store total production and consumption flux per metabolite class
df_prod_cons = pd.DataFrame(index=met_classes, columns=['Production', 'Consumption'])
df_prod_cons_percentage = pd.DataFrame(index=go_terms)
#Create figure with subplots
fig_exp31 = make_subplots(
rows=3,
cols=2,
subplot_titles = ['<b>Production</b>','<b>Consumption</b>'] * 3,
row_titles = [f'<b>({list(string.ascii_uppercase)[i]}) {met_class}</b>'
for i, met_class in enumerate(met_classes)],
specs=[[{"type": "pie"}, {"type": "pie"}],[{"type": "pie"}, {"type": "pie"}],[{"type": "pie"}, {"type": "pie"}]],
vertical_spacing = 0.0
)
#Create pie charts reflecting the proportions of a metabolite class produced or consumped by Go Terms
for i, met_class in enumerate(met_classes):
#Find the specific metabolite ids in all compartments
met_ids = c3_model_exp3.metabolites.query(lambda x: x.id.startswith(met_class)).list_attr('id')
#Set up dictionaries to hold fluxes producing or consuming a certain metabolite
fluxes_prod_met_class = {}
fluxes_cons_met_class = {}
for met_id in met_ids:
#Get reactions and fluxes producing or consuming a certain metabolite (excluding transport, import and export reactions)
fluxes = {r_obj.id: result_exp3_pfba.fluxes[r_obj.id] * r_obj.get_coefficient(met_id)
for r_obj in c3_model_exp3.metabolites.get_by_id(met_id).reactions
if not r_obj.id[:3] in ['Tr_', 'Im_', 'Ex_']}
#Extract reactions and fluxes producing a certain metabolite
fluxes_prod = {r_id: abs(flux) for r_id, flux in fluxes.items() if flux > 0}
fluxes_prod_met_class = {**fluxes_prod_met_class, **fluxes_prod}
#Extract reactions and fluxes consuming a certain metabolite
fluxes_cons = {r_id: abs(flux) for r_id, flux in fluxes.items() if flux < 0}
fluxes_cons_met_class = {**fluxes_cons_met_class, **fluxes_cons}
#Store total fluxes
df_prod_cons.loc[met_class] = [sum(fluxes_prod_met_class.values()), sum(fluxes_cons_met_class.values())]
#Plot porportion of fluxes by Go Terms
trace_prod, go_term_fluxes_prod, trace_cons, go_term_fluxes_cons = prod_cons_charts(met_class, fluxes_prod_met_class, fluxes_cons_met_class, go_terms, df_go_term)
#Add trace to figure
#fig_exp31.append_trace(trace_go,i+1,1)
#Add trace to figure
fig_exp31.append_trace(trace_prod,i+1,1)
#Add trace to figure
fig_exp31.append_trace(trace_cons,i+1,2)
#save flux sum for GO Terms
df_prod_cons_percentage[f'{met_class}_prod'] = np.nan
df_prod_cons_percentage[f'{met_class}_prod'].loc[go_term_fluxes_prod.index] = go_term_fluxes_prod
df_prod_cons_percentage[f'{met_class}_cons'] = np.nan
df_prod_cons_percentage[f'{met_class}_cons'].loc[go_term_fluxes_cons.index] = go_term_fluxes_cons
df_prod_cons_percentage.dropna(how='all', inplace=True)
df_prod_cons_percentage = df_prod_cons_percentage / df_prod_cons_percentage.sum() * 100
df_prod_percentage = df_prod_cons.loc[:,'Production'] / df_prod_cons['Production'].sum() * 100
#Update traces
fig_exp31.update_traces(
textposition='inside',
textinfo='percent'
)
#Get all GO terms in figure
go_term_labels = list(set([label for trace in fig_exp31['data'] for label in trace['labels']]))
#Create list colors according to number of GO terms in figure
colors = ['hsl('+str(h)+',50%'+',50%)' for h in np.linspace(0, 360, len(go_term_labels))]
#Assign color to GO term
go_term_color = pd.Series(index=go_term_labels, data=colors)
#Re-color trace in figure
for trace in fig_exp31['data']:
trace['marker']['colors'] = go_term_color[trace['labels']].values
#Update annotations
fig_exp31.update_annotations(
font=dict(size=18)
)
#Re-position subfigure enumeration
for anno in fig_exp31['layout']['annotations']:
if anno['xanchor'] == 'left':
anno['x'] = 0.1
anno['y'] = anno['y'] + 0.15
anno['textangle'] = 0
anno['xanchor'] = 'right'
if 'Production' in anno['text'] or 'Consumption' in anno['text']:
anno['y'] = anno['y'] - 0.025
#Update layout
fig_exp31.update_layout(
uniformtext_minsize=12,
uniformtext_mode='hide',
width=1000,
height=2000,
legend=dict(
font=dict(size=18),
orientation = 'h',
xanchor = 'center',
x = 0.5,
y = 0.05
)
)
#Show figure
fig_exp31.show()
Energy Flux Distribution in the one-cell Model. (A) ATP production and consumption, (B) NADPH production and consumption, (C) NADH production and consumption.
#########################################################################
############## Figure 1—figure supplement 4 ##############
#########################################################################
#{
# "caption": "### Energy Flux Distribution in the _one-cell_ Model. (**A**) proportion of ATP, NADPH, NADH used as energy equivalent, (**B**) proportion of respiratory ATP used for maintenance.",
# "id": "fig1s4",
# "label": "Figure 1—figure supplement 4",
# "trusted": true
#}
#Create figure with subplots
fig_exp32 = make_subplots(
rows=1,
cols=2,
subplot_titles = ['<b>(A) Proportion of Energy Equivalents</b>', '<b>(B) Proportion of Respiratory ATP</b>'],
specs=[[{"type": "pie"}, {"type": "pie"}]])
#Create trace for energy equivalents
trace_energy = go.Pie(
labels = df_prod_cons.index,
values = df_prod_cons['Production'],
marker=dict(line=dict(color='#FFF', width=1)),
showlegend = False,
)
#Add trace to figure
fig_exp32.append_trace(trace_energy,1,1)
#Create trace for energy maintenace in respect to respiratory ATP
rxn_maintenance = ['NGAM_h','NGAM_c','NGAM_m']
rxn_maintenance_flux = [result_exp3_pfba.fluxes[r_id] for r_id in rxn_maintenance]
atp_flux = result_exp3_pfba.fluxes['cplx5_m'] * c3_model_exp3.reactions.get_by_id('cplx5_m').get_coefficient('ATP_m')
atp_maintenance_percentage = sum(rxn_maintenance_flux) / (atp_flux) * 100
trace_maintenance = go.Pie(
labels = rxn_maintenance + ['Others'],
values = rxn_maintenance_flux + [atp_flux - sum(rxn_maintenance_flux)],
textfont=dict(size=18,family='Arial',),
marker=dict(line=dict(color='#FFF', width=1)),
showlegend = False,
)
#Add trace to figure
fig_exp32.append_trace(trace_maintenance,1,2)
#Update traces
fig_exp32.update_traces(
textposition='outside',
textinfo='percent+label'
)
#Update annotations
fig_exp32.update_annotations(
font=dict(size=18)
)
#Update layout
fig_exp32.update_layout(
uniformtext_minsize=16,
uniformtext_mode='hide',
width=1000,
height=500)
#Show figure
fig_exp32.show()
Energy Flux Distribution in the one-cell Model. (A) proportion of ATP, NADPH, NADH used as energy equivalent, (B) proportion of respiratory ATP used for maintenance.
null
The one-cell model requires a photosynthetic photon flux density (PPFD) of {{round(resultexp3_pfba'Im_hnu',2)}} μmol/(m2s) (Table 2). The _one-cell model takes up the maximal amount of CO2 to produce the maximum amount of phloem sap, as well as {{round(result_exp3_pfba'Im_NO3',2)}} μmol/(m2s) of NO3- and {{round(result_exp3_pfba'Im_H2O',2)}} μmol/(m2s) of H2O. According to the assumed ratio of sucrose and amino acids in the phloem sap, the flux of sucrose predicted by the model is {{round(result_exp3_pfba'Ex_Suc',2)}} μmol/(m2s) and of amino acids {{round(result_exp3_pfba'Ex_AA',2)}} μmol/(m2s). The rate of oxygen supply by the network is {{round(result_exp3_pfba'Ex_O2',2)}} μmol/(m2s). Part of the complete flux table is displayed in Table 2; the full table is available, see Figure 1—source data 2. The flux table of all reactions did not display circular fluxes, and the reactions were within expected physiological ranges (Figure 1—source data 2).
Molecular Species | Flux [µmol/(m2/s)] | Physiological Range [µmol/(m2/s)] | Reference |
---|---|---|---|
(i) Inputs | |||
Photons | {{round(result_exp3_pfba'Im_hnu',2)}} | 100 - 400 | 5Bailey et al.2001 |
CO2 | {{round(result_exp3_pfba'Im_CO2',2)}} | 20 | 37Lacher2003 |
NO3- | {{round(result_exp3_pfba'Im_NO3',2)}} | 0.11 - 0.18 | 35Kiba et al.2012 |
H2O | {{round(result_exp3_pfba'Im_H2O',2)}} | - | |
(ii) Outputs | |||
O2 | {{round(result_exp3_pfba'Ex_O2',2)}} | 16.5 | 75Sun et al.1999 |
Amino Acids | {{round(result_exp3_pfba'Ex_AA',2)}} | - | |
Sucrose/Starch | {{round(result_exp3_pfba'Ex_Suc',2)}} | - |
The CO2 uptake rate and the phloem sap output have a positive linear relationship, see Figure 1—figure supplement 1. The same is true for the correlation of the PPFD and phloem sap output in the range of 100 μmol/(m2s)–200 μmol/(m2s), see Figure 1—figure supplement 2). Above 200 μmol/(m2s), the CO2 uptake rate acts as a limiting factor restricting the increase of phloem sap production. If either the PPFD or the CO2 uptake rate is zero, the phloem sap cannot be produced, compare Figure 1—figure supplement 1 and Figure 1—figure supplement 2. Most of the metabolic processes use ATP/ADP as main energy equivalent ({{round(df_prod_percentage'ATP',2)}}%), followed by NADP/NADPH ({{round(df_prod_percentage'NADPH',2)}}%) and NAD/NADH ({{round(df_prod_percentage'NADH',2)}}%), see Figure 1—figure supplement 4(A). Nearly all ATP is produced by the light reactions ({{round(df_prod_cons_percentage.loc'photosynthesis, light reaction','ATP_prod',2)}}%) and consumed by the reductive pentose phosphate cycle ({{round(df_prod_cons_percentage.loc'reductive pentose-phosphate cycle','ATP_cons',2)}}%), see Figure 1—figure supplement 3(A). The oxidative phosphorylation produces only ({{round(df_prod_cons_percentage.loc'oxidative phosphorylation','ATP_prod',2)}}%) of ATP. In proportion, the maintenance cost for protein synthesis and degradation makeup {{round(atp_maintenance_percentage,2)}}% of the respiratory ATP produced by the oxidative phosphorylation (Figure 1—figure supplement 4(B)). Similarly, nearly all NADPH is produced by the light reaction ({{round(df_prod_cons_percentage.loc'photosynthesis, light reaction','NADPH_prod',2)}}%), which is consumed by the reductive pentose-phosphate cycle ({{round(df_prod_cons_percentage.loc'reductive pentose-phosphate cycle','NADPH_cons',2)}}%) as well (Figure 1—figure supplement 3(C)). The canonical glycolysis and photorespiration produce nearly equal amounts of NADH, {{round(df_prod_cons_percentage.loc'canonical glycolysis','NADH_prod',2)}}% and {{round(df_prod_cons_percentage.loc'photorespiration','NADH_prod',2)}}%), significantly less NADH is produced through gluconeogenesis ({{round(df_prod_cons_percentage.loc'gluconeogenesis','NADH_prod',2)}}%) and pyruvate dehydrogenase activity ({{round(df_prod_cons_percentage.loc'pyruvate dehydrogenase activity','NADH_prod',2)}})%. Photorespiration ({{round(df_prod_cons_percentage.loc'photorespiration','NADH_cons',2)}}%), Nitrate assimilation ({{round(df_prod_cons_percentage.loc'nitrate assimilation','NADH_cons',2)}}%), glutamate biosynthesis ({{round(df_prod_cons_percentage.loc'glutamate biosynthetic process','NADH_cons',2)}}%), glyoxylate cycle ({{round(df_prod_cons_percentage.loc'glyoxylate cycle','NADH_cons',2)}}%) and alternative respiration ({{round(df_prod_cons_percentage.loc'alternative respiration','NADH_cons',2)}}%) consume the produced NADH (Figure 1—figure supplement 3(B)).
A C4 cycle is predicted under resource limitation
To rebuild the characteristic physiology of C4 leaves, we duplicated the one-cell model and connected the two network copies by bi-directional transport of cytosolic metabolites including amino acids, sugars, single phosphorylated sugars, mono-/di-/tri-carboxylic acids, glyceric acids, glycolate, glycerate, glyceraldehyde-3-phosphate, di-hydroxyacetone-phosphate and CO2, see Materials and methods for details. Since CBM is limited to static model analysis, we introduced two Rubisco populations in the bundle sheath network to approximate CO2 concentration-dependent changes in the oxygenation : carboxylation ratio of Rubisco () itself. We kept the native constrained Rubisco population that is forced to undertake oxygenation reactions and added a CCM-dependent Rubisco population which can only carboxylate ribulose 1,5-bisphosphate. The CCM-dependent Rubisco population is only able to use CO2 produced by the bundle sheath network but not environmental CO2 released by the mesophyll. C4 plants have a higher CO2 consumption and thus, an increased CO2 uptake of {{fC4_CO2_M}} μmol/(m2s) was allowed 38Leakey et al.2006. All other constraints and the objective of the _one-cell model are maintained in the two-cell model, see Figure 2.
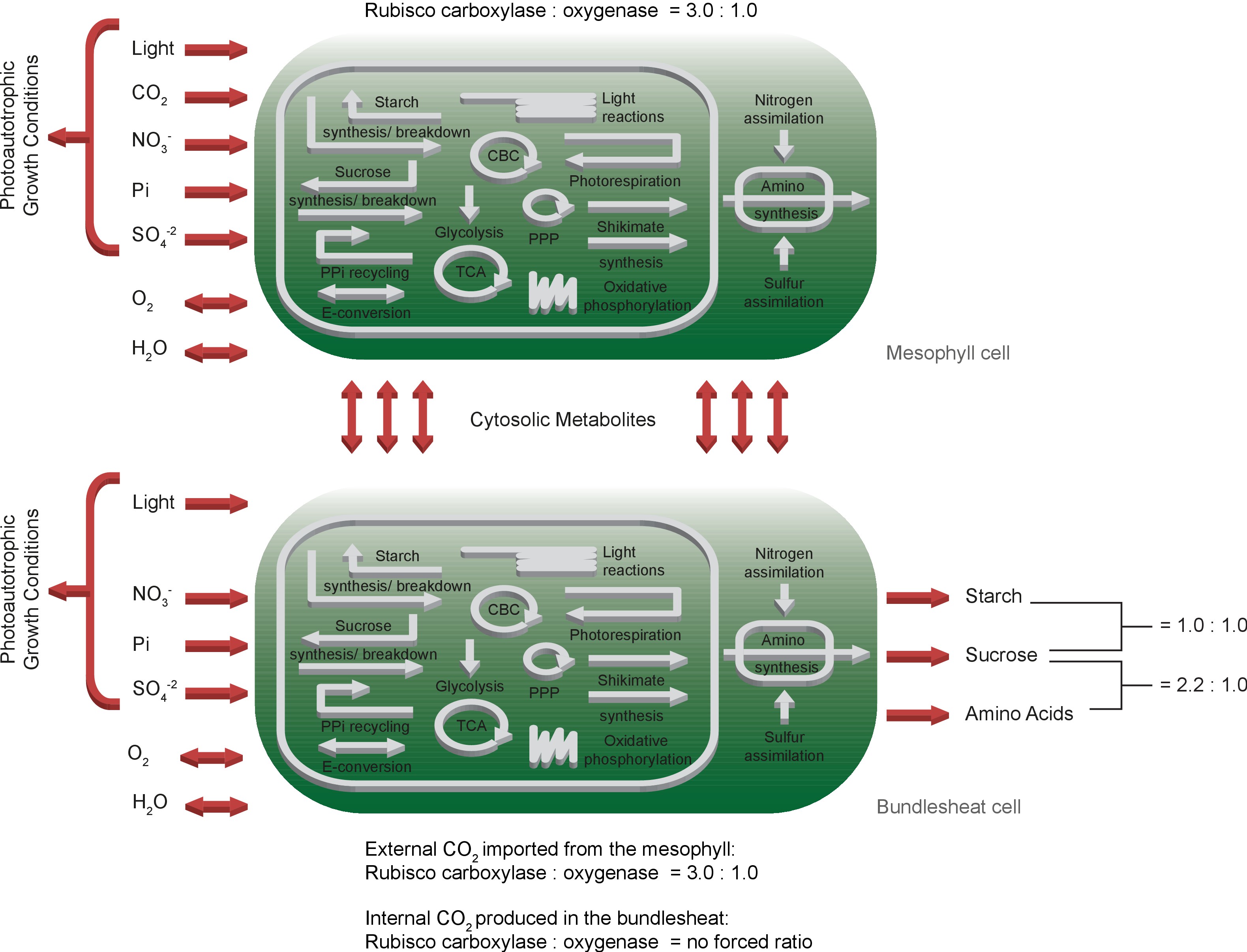
Schematic representation of the primary subsystems in the two-cell model and the used input/output constraints; adapted from 2Arnold and Nikoloski2014.
######################################################################################################################################
######################################################################################################################################
############## EXPERIMENT 4: Effect of Photorespiratory Flux (Decarboxylation-Oxygenation Ratio) ##############
######################################################################################################################################
######################################################################################################################################
display(HTML(f"<h1>Code Cell 28: Experiment 4-- Effect of Photorespiratory Flux (Decarboxylation-Oxygenation Ratio) </h1>"))
#Remove original c4_rbc_rbo constraints from c4 model
c4_model.remove_cons_vars(const_c4_rbc_rbo_b)
c4_model.remove_cons_vars(const_c4_rbc_rbo_m)
#Create copy of c4 model
c4_model_exp4 = c4_model.copy()
#Reaction Variables
B_Ex_Suc = c4_model_exp4.reactions.get_by_id("[B]_Ex_Suc")
#Proportions of Decarboxylation in the RBC : RBO ratio
rbc_proportions = np.arange(1,10.25,0.25)
#Initiate dataframe to save results
df_result_exp4 = pd.DataFrame(index=c4_model.reactions.list_attr('id'), columns=rbc_proportions)
#Iterate over
for rbc_value in tqdm(rbc_proportions):
#Set c4_rbc_rbo constraints
r_c4_rbc_rbo = (float(rbc_value), 1.0)
const_c4_rbc_rbo_b_exp4 = set_fixed_flux_ratio({'[B]_RBC_h_Ex':r_c4_rbc_rbo[0],'[B]_RBO_h':r_c4_rbc_rbo[1]}, 'const_c4_rbc_rbo_b_exp4', c4_model_exp4)
const_c4_rbc_rbo_m_exp4 = set_fixed_flux_ratio({'[M]_RBC_h':r_c4_rbc_rbo[0],'[M]_RBO_h':r_c4_rbc_rbo[1]}, 'const_c4_rbc_rbo_m_exp4', c4_model_exp4)
#Optimize/Maximize sucrose output
B_Ex_Suc.objective_coefficient = 1.
result_exp4_fba = c4_model_exp4.optimize('maximize')
#Optimize/Minimize total flux
if result_exp4_fba.status == 'optimal':
result_exp4_pfba = cobra.flux_analysis.parsimonious.pfba(c4_model_exp4)
df_result_exp4[rbc_value] = result_exp4_pfba.fluxes
#Remove c4_rbc_rbo constraint
c4_model_exp4.remove_cons_vars(const_c4_rbc_rbo_b_exp4)
c4_model_exp4.remove_cons_vars(const_c4_rbc_rbo_m_exp4)
#Reset original c4_rbc_rbo constraints in c4 model
c4_model.add_cons_vars(const_c4_rbc_rbo_b)
c4_model.add_cons_vars(const_c4_rbc_rbo_m)
Code Cell 28: Experiment 4-- Effect of Photorespiratory Flux (Decarboxylation-Oxygenation Ratio)
0%| | 0/37 [00:00<?, ?it/s]
Initially, we optimised for the classical objective function of minimal total flux through the metabolic network at different levels of photorespiration. These different levels of photorespiration integrate changes to external CO2 concentration and stomatal opening status which is governed by plant water status and biotic interactions. From the complete flux distribution, we extracted fluxes of PEPC and PPDK, the decarboxylation enzymes, Rubisco and metabolite transporter between the two cells to ascertain the presence of a C4 cycle, see Figure 3, Figure 3, and Figure 3—figure supplement 1. At low photorespiratory levels, flux through PEPC is barely detectable (Figure 3(A)). If photorespiration increases to moderate levels, flux through PEPC can be predicted and increases to {{f_C4_CO2_M}} μmol/(m2s), that is all CO2 is funnelled through PEPC, for high photorespiratory fluxes. Concomitant with flux through PEPC, the activity of the decarboxylation enzymes changes (Figure 3(B)). At low to intermediate levels of photorespiratory flux, glycine decarboxylase complex activity is predicted to shuttle CO2 to the bundle sheath at up to {{round(df_result_exp4.loc['B_GlyDH_m'].max(),2)}} μmol/(m2s). Decarboxylation of C4 acids is initially mostly mediated by PEP-CK and is largely taken over by NADP-ME at high fluxes through photorespiration. Flux through NAD-ME is very low under all photorespiration levels. The decarboxylation enzymes dictate flux through the different Rubiscos in the model (Figure 3(C)). At low photorespiratory flux, both the Rubiscos in mesophyll and bundle sheath are active. Only very little flux occurs through the CCM-dependent Rubisco, which is a result of the glycine decarboxylase (Figure 3(B)). With increasing photorespiratory flux, this flux through glycine decarboxylase increases (Figure 3(B)) and therefore, total Rubisco activity exceeds the carbon intake flux (Figure 3(C)). Carbon fixation switches to the CCM-dependent Rubisco with increasing flux through PEPC (Figure 3(A)) and the classic C4 cycle decarboxylation enzymes (Figure 3(B)). Flux through PPDK mostly reflects flux through PEPC (Figure 3(D)). The transport fluxes between the cells change with changing photosynthetic mode (Figure 4).
########################################################
############## Figure 3. ##############
########################################################
#{
# "caption": "### Effect of oxygenation : carboxylation ratio on the major steps in C4 cycle, including (**A**) activity of phosphoenolpyruvate carboxylase (PEPC), (**B**) activity of Rubisco, (**C**) activity of the decarboxylation enzymes, (**D**) activity of pyruvate phosphate dikinase (PPDK)",
# "id": "fig3",
# "label": "Figure 3.",
# "trusted": true
#}
#Dictionary of c4 cycle reactions and their subplots
D_rxn ={
(1,1): {'rxn':['[M]_PEPC2_c', '[B]_PEPC2_c'], 'title':'PEPC'},
(1,2): {'rxn':['[B]_MalDH4_h', '[B]_MalDH2_m', '[B]_PEPC1_c', '[B]_GlyDH_m' ], 'title': 'Decarboxylation Enzymes'},
(2,1): {'rxn':['[M]_RBC_h', '[B]_RBC_h_Ex', '[B]_RBC_h'], 'title': 'Decarboxylation by Rubisco'},
(2,2): {'rxn':['[M]_PyrPiDK_h','[B]_PyrPiDK_h' ], 'title':'PPDK'}
}
#Dictionary of legend names for c4 cycle reactions
D_rxn_alias = {
'[M]_PEPC2_c': ('Mesophyll',0),
'[B]_PEPC2_c': ('Bundle Sheath',1),
'[B]_MalDH4_h': ('NADP-ME',6),
'[B]_MalDH2_m': ('NAD-ME',7),
'[B]_PEPC1_c': ('PEPCK',8),
'[B]_GlyDH_m': ('Gly DH',9),
'[M]_RBC_h': ('Mesophyll, constrained',0),
'[B]_RBC_h_Ex': ('Bundle sheath, constrained',1),
'[B]_RBC_h': ('Bundle sheath, unconstrained',2),
'[M]_PyrPiDK_h': ('Mesophyll',0),
'[B]_PyrPiDK_h':('Bundle Sheath',1)
}
#Create figure with subplots
fig_exp41 = make_subplots(rows=2,
cols=2,
subplot_titles=[f"<b>({list(string.ascii_uppercase)[i]}) {info['title']}</b>" for i, info in enumerate(D_rxn.values())],
y_title= 'Flux [µmol/(m\u00B2s)]',
x_title= 'Rubisco Carboxylation : Oxygenation Ratio',
#horizontal_spacing=0,
vertical_spacing=0.1,
specs=[[{},{}],[{},{}]]
)
#Add traces to the figure
for i, item in enumerate(D_rxn.items()):
pos_x_y = item[0]
info = item[1]
#Add empty trace to group legend by title
trace = go.Scatter(
x = [None],
y = [None],
name = f"<b>({list(string.ascii_uppercase)[i]}) {info['title']}</b>",
legendgroup = info['title'],
mode = 'markers',
marker = dict(color='black', symbol='triangle-right')
)
fig_exp41.add_trace(trace,pos_x_y[0],pos_x_y[1])
for r_id in info['rxn']:
#Add trace with an a reaction group
trace = go.Bar(
x = df_result_exp4.columns,
y = abs(df_result_exp4.loc[r_id]),
name = D_rxn_alias[r_id][0],
legendgroup=info['title'],
marker=dict(color = plotly.colors.DEFAULT_PLOTLY_COLORS[D_rxn_alias[r_id][1]])
)
fig_exp41.add_trace(trace,pos_x_y[0],pos_x_y[1])
#Update xaxes
fig_exp41.update_xaxes(
tickfont=dict(size=16),
ticksuffix=' : 1',
tickangle=25,
title=dict(font=dict(size=18))
)
#Update yaxes
fig_exp41.update_yaxes(
tickfont=dict(size=16),
title=dict(font=dict(size=18))
)
#Update annotations
fig_exp41.update_annotations(font=dict(size=18))
#Update layout
fig_exp41.update_layout(
barmode='stack',
width= 1000,
height=800,
legend=dict(
orientation = 'v',
tracegroupgap = 10,
traceorder='grouped',
font=dict(size=18),
)
)
#Show figure
fig_exp41.show()
Effect of oxygenation : carboxylation ratio on the major steps in C4 cycle, including (A) activity of phosphoenolpyruvate carboxylase (PEPC), (B) activity of Rubisco, (C) activity of the decarboxylation enzymes, (D) activity of pyruvate phosphate dikinase (PPDK)
########################################################
############## Figure 4. ##############
########################################################
#(E) and (F) have been integrated into one graph
#{
# "caption": "### Effect of oxygenation : carboxylation ratio on the metabolite exchange between mesophyll and bundle sheath cells in the in C4 cycle. Positive fluxes represent the transport of metabolites from the mesophyll to the bundle sheath, negative fluxes indicate the transport of metabolites from the bundle sheath to the meophyll.",
# "id": "fig4",
# "label": "Figure 4.",
# "trusted": true
#}
#Get exchange reaction between mesophyll and bundle sheath
rxn_mb_transport = c4_model.reactions.query(lambda rxn: (rxn.id.startswith('[MB]'))).list_attr('id')
#Extract fluxes for exchange reactions
df_result_exp4_transport = df_result_exp4.loc[rxn_mb_transport]
df_result_exp4_transport = df_result_exp4_transport[(abs(df_result_exp4_transport) > 0.01).sum(axis=1) > 0]
df_result_exp4_transport = df_result_exp4_transport.reindex(df_result_exp4_transport.mean(axis=1).sort_values().index)
#Create Figure
fig_exp42 = go.Figure()
#Add trace
trace = go.Heatmap(
x=df_result_exp4_transport.columns,
y=df_result_exp4_transport.index.str.split('_',expand=True).get_level_values(1),
z=df_result_exp4_transport,
colorscale = 'RdBu_R',
colorbar = dict(
tickfont=dict(size=16),
title = dict(
text='<b>Flux [µmol/(m\u00B2s)]</b>',
side='right',
font=dict(size=18)
)
)
)
fig_exp42.add_trace(trace)
#Update axes
fig_exp42.update_xaxes(
tickfont=dict(size=16),
ticksuffix=' : 1',
tickangle=25,
title= dict(text='Rubisco Carboxylation : Oxygenation Ratio',font=dict(size=18))
)
#Update yaxes
fig_exp42.update_yaxes(
tickfont=dict(size=16)
)
#Update layout
fig_exp42.update_layout(
barmode='stack',
width= 1000,
height=800,
title = dict(
text = '<b>(E) + (F) Transport between Mesophyll and Bundle Sheath</b>',
font = dict(size=18),
x = 0.5
)
)
#Show figure
fig_exp42.show()
Effect of oxygenation : carboxylation ratio on the metabolite exchange between mesophyll and bundle sheath cells in the in C4 cycle. Positive fluxes represent the transport of metabolites from the mesophyll to the bundle sheath, negative fluxes indicate the transport of metabolites from the bundle sheath to the meophyll.
null
##############################################################
############## FIGURE 3 - Supplement 1 ##############
##############################################################
#{
# "caption": "### Flux maps illustrating the effect of the oxygenation : carboxylation ratio of Rubisco on the C3-C4 trajectory. Flux maps illustrating the effect of the proportion of photorespiratory flux through Rubisco. (**A**) Low photorespiratory flux; (**B**) Moderate photorespiratory flux; and (**C**) High photorespiratory flux. (Arc width and colour are set relative to flux values in μmol/(m^2^s), grey arcs - no flux).",
# "id": "fig3s1",
# "label": "Figure 3—figure supplement 1",
# "trusted": true
#}
# Rubisco carboxylation proportion to display on metabolic map
show_co2_proportion = {1: 'High Photorespiratory Flux',3: 'Moderate Photorespiratory Flux', 10: 'Low Photorespiratory Flux'}
# Build metabolic map and add flux solution for each ratio
for i, co2_proportion in enumerate(sorted(show_co2_proportion.keys(), reverse=True)):
#Build map
b = Builder(
map_json = 'elife-49305.ipython.src/2018-06-29-mb-C4-RBO-RBC-Ratio.json',
reaction_styles = ['color','size', 'text'],
reaction_data = df_result_exp4[co2_proportion],
reaction_scale = [
{'type': 'value', 'value': 40, 'color': '#ff0000', 'size': 25},
{'type': 'value', 'value': -40, 'color': '#ff0000', 'size': 25},
{'type': 'value', 'value': 20, 'color': '#209123', 'size': 20},
{'type': 'value', 'value': -20, 'color': '#209123', 'size': 20},
{'type': 'value', 'value': 0.01, 'color': '#9696ff', 'size': 5},
{'type': 'value', 'value': -0.01, 'color': '#9696ff', 'size': 5},
{'type': 'value', 'value': 0, 'color': '#ccc', 'size': 3}],
menu = False,
height = 1000
)
#Add title
display(HTML(f"<h1>({list(string.ascii_uppercase)[i]}) {show_co2_proportion[co2_proportion]}</h1>"))
#Display
display(b)
(A) Low Photorespiratory Flux
(B) Moderate Photorespiratory Flux
(C) High Photorespiratory Flux
Flux maps illustrating the effect of the oxygenation : carboxylation ratio of Rubisco on the C3-C4 trajectory. Flux maps illustrating the effect of the proportion of photorespiratory flux through Rubisco. (A) Low photorespiratory flux; (B) Moderate photorespiratory flux; and (C) High photorespiratory flux. (Arc width and colour are set relative to flux values in μmol/(m2s), grey arcs - no flux).
null
At low rates of photorespiration when PEPC is barely active, the only flux towards the bundle sheath is CO2 diffusion with no fluxes towards the mesophyll (Figure 4). In the intermediate phase glycolate and glycerate are predicted to be transported and a low-level C4 cycle dependent on the transport of aspartate, malate, PEP and alanine operates (Figure 4). In case of high photorespiratory rates, the exchange between mesophyll and bundle sheath is mainly carried by malate and pyruvate (Figure 4). Flux through PPDK (Figure 3(D)) is lower than flux through PEPC (Figure 3(A)) at the intermediate stage. Evolution of C4 photosynthesis with NADP-ME as the major decarboxylation enzyme is predicted if the photorespiratory flux is high and model optimised for minimal total flux, in other words, resource limitation.
C4 modes with different decarboxylation enzymes result from different set of constraints
######################################################################################################################################
######################################################################################################################################
############## EXPERIMENT 5: Effect of Decarboxylation Enzymes on the c4 mode ##############
######################################################################################################################################
######################################################################################################################################
display(HTML(f"<h1>Code Cell 32: Experiment 5 -- Effect of Decarboxylation Enzymes on the c4 mode </h1>"))
#Create copy of c4 model
c4_model_exp5 = c4_model.copy()
#Reaction variables
B_Ex_Suc = c4_model_exp5.reactions.get_by_id("[B]_Ex_Suc")
B_RBO = c4_model_exp5.reactions.get_by_id("[B]_RBO_h")
M_RBO = c4_model_exp5.reactions.get_by_id("[M]_RBO_h")
#Decarboxylation reactions ids and names of enzymes
c4_mode_r_id_enzyme = {'[B]_MalDH4_h': 'NADP-ME', '[B]_PEPC1_c': 'PEP-CK', '[B]_MalDH2_m': 'NAD-ME',}
#Set flux through decarboxylation enzymes two zero
for c4_mode_r_id in c4_mode_r_id_enzyme.keys():
set_fixed_flux(c4_mode_r_id, 0, c4_model_exp5)
#Initialise data frames to hold results
df_result_exp5_pfba = pd.DataFrame(index=c4_model.reactions.list_attr('id'), columns=c4_mode_r_id_enzyme.keys(), dtype='float64')
df_result_exp5_pfva = pd.DataFrame(index=c4_model.reactions.list_attr('id'), columns=c4_mode_r_id_enzyme.keys(), dtype='float64')
#Get exchange reaction between mesophyll and bundle sheath
rxn_mb_transport = c4_model.reactions.query(lambda rxn: (rxn.id.startswith('[MB]'))).list_attr('id')
#Perform FBA experiment for each decarboxylation enzyme
for c4_mode_r_id in tqdm(c4_mode_r_id_enzyme.keys()):
#Allow non-zero flux for current decarboxylation enzyme
set_bounds(c4_mode_r_id, (0,inf), c4_model_exp5)
#Optimization - Maximize sucrose output & Minimize Oxygenation by Rubisco
B_Ex_Suc.objective_coefficient = 1.
B_RBO.objective_coefficient = -1.
M_RBO.objective_coefficient = -1.
result_exp5_fba = c4_model_exp5.optimize('maximize')
#Optimize/Minimize total flux
if result_exp5_fba.status == 'optimal':
result_exp5_pfba = cobra.flux_analysis.parsimonious.pfba(c4_model_exp5)
df_result_exp5_pfba[c4_mode_r_id] = result_exp5_pfba.fluxes
pfba_factor = 0.015
result_exp5_pfva = cobra.flux_analysis.flux_variability_analysis(c4_model_exp5,reaction_list=rxn_mb_transport, pfba_factor= 1 + pfba_factor)
df_result_exp5_pfva[c4_mode_r_id] = result_exp5_pfva.apply(lambda x: (x[0], x[1]), axis=1)
#Reset flux of current decarboxylation enzyme to zero
set_fixed_flux(c4_mode_r_id, 0, c4_model_exp5)
Code Cell 32: Experiment 5 -- Effect of Decarboxylation Enzymes on the c4 mode
0%| | 0/3 [00:00<?, ?it/s]
Among the known independent evolutionary events leading to C4 photosynthesis, 20 are towards NAD-ME while 21 occurred towards NADP-ME 62Sage2004. PEP-CK is dominant or at least co-dominant only in Panicum maximum012Bräutigam et al.2014, Alloteropsis semialata semialata018Christin et al.2012, and in the Chloridoideae062Sage2004. To analyse whether the predicted evolution of the C4 cycle is independent of a particular decarboxylation enzyme, we performed three separate experiments, where only one decarboxylation enzyme can be active at a time. The other decarboxylation enzymes were de-activated by constraining the reaction flux to zero resulting in three different predictions, one for each decarboxylation enzyme. The flux distributions obtained under the assumption of oxygenation : carboxylation ratio of 1 : 3 and minimisation of photorespiration as an additional objective predicts the emergence of a C4 cycle for each known decarboxylation enzyme. To visualise the possible C4 fluxes, the flux distribution for candidate C4 cycle enzymes was extracted from each of the three predictions and visualised as arc width and color (Figure 4). While the flux distribution in the mesophyll is identical for three predicted C4 cycles of the decarboxylation enzymes, it is diverse in the bundle sheath due to the different localisation of the decarboxylation and related transport processes, see Figure 4. The flux distribution does not completely mimic the variation in transfer acids known from laboratory experiments 30Hatch1987 since all of the decarboxylation enzymes use the malate/pyruvate shuttle. In the case of NAD-ME and PEP-CK, the two-cell model also predicts a supplementary flux through the aspartate/alanine shuttle. We tested whether transfer acids other than malate and pyruvate are feasible and explored the near-optimal space. To this end, the model predictions are repeated, allowing deviation from the optimal solution and the changes recorded. Deviations from the optimal solution are visualised as error bars (Figure 5). Performing a flux variability analysis (FVA) and allowing the minimal total flux to differ by {{pfba_factor * 100}}%, predicts that for most metabolites which are transferred between mesophyll and bundle sheath, the variability is similar for all three decarboxylation types. For the NAD-ME and PEP-CK types, changes in the near-optimal space were observed for the transfer acids malate, aspartate, pyruvate and alanine. Minor differences were present for triose phosphates and phosphoglycerates as well as for PEP. For the NADP-ME type, FVA identifies only minor variation (Figure 5). In the case of NAD-ME but not in the case of NADP-ME the activity of the malate/pyruvate shuttle can be taken over by the aspartate/alanine shuttle and partly taken over in case of PEP-CK, see Figure 5. The aspartate/alanine shuttle is thus only a near-optimal solution when the model and by proxy evolutionary constraints are resource efficiency and minimal photorespiration.
########################################################
############## Figure 5. ##############
########################################################
#{
# "caption": "### Flux maps illustrating the effect of the C4 mode. (**A**) NADP-ME, (**B**) PEP-CK, (**C**) NAD-ME. (Arc width and colour are set relative to flux values in μmol/(m^2^s), grey arcs - no flux).",
# "id": "fig5",
# "label": "Figure 5.",
# "trusted": true
#}
# Build metabolic map and add flux solution for decarboxylation enzyme
for i, c4_mode_r_id in enumerate(c4_mode_r_id_enzyme.keys()):
#Build map
b = Builder(
map_json = 'elife-49305.ipython.src/2018-06-29-mb-C4-Map-Decarb-Enzymes.json',
reaction_styles = ['color','size', 'text'],
reaction_data = df_result_exp5_pfba[c4_mode_r_id],
reaction_scale = [
{'type': 'value', 'value': 40, 'color': '#ff0000', 'size': 25},
{'type': 'value', 'value': -40, 'color': '#ff0000', 'size': 25},
{'type': 'value', 'value': 20, 'color': '#209123', 'size': 20},
{'type': 'value', 'value': -20, 'color': '#209123', 'size': 20},
{'type': 'value', 'value': 0.01, 'color': '#9696ff', 'size': 5},
{'type': 'value', 'value': -0.01, 'color': '#9696ff', 'size': 5},
{'type': 'value', 'value': 0, 'color': '#ccc', 'size': 3}],
menu = False,
height = 1000
)
#Add title
display(HTML(f"<h3>({list(string.ascii_uppercase)[i]}) {c4_mode_r_id_enzyme[c4_mode_r_id]}</h3>"))
#Display
display(b)
(A) NADP-ME
(B) PEP-CK
(C) NAD-ME
Flux maps illustrating the effect of the C4 mode. (A) NADP-ME, (B) PEP-CK, (C) NAD-ME. (Arc width and colour are set relative to flux values in μmol/(m2s), grey arcs - no flux).
null
########################################################
############## Figure 6. ##############
########################################################
#{
# "caption": "### Flux variability analysis of metabolite exchange with 1.5% deviation of the total flux minimum. The upper bar defines the maximum exchange flux, while the lower bar defines the minimum exchange flux, points indicate the value of the original flux solution under minimal metabolic effort constraint. Positive flux values correspond to the transport direction from mesophyll to bundle sheath, negative values to the transport direction from bundle sheath to mesophyll.",
# "id": "fig6",
# "label": "Figure 6.",
# "trusted": true
#}
#Create figure with subplots
fig_exp5 = make_subplots(
rows = 3,
cols = 1,
subplot_titles = [f"<b>FVA with {enzyme} (pFBA Factor = 1.5 %)</b>" for enzyme in c4_mode_r_id_enzyme.values()],
y_title = 'Flux [µmol/(m\u00B2s)]',
x_title = 'Exchange Metabolites',
vertical_spacing=0.1
)
#Get exchange reaction between mesophyll and bundle sheath
rxn_mb_transport = c4_model.reactions.query(lambda rxn: (rxn.id.startswith('[MB]'))).list_attr('id')
#Add subplot for each decarboxylation enzyme
for i, c4_mode_r_id in enumerate(c4_mode_r_id_enzyme.keys()):
#Create trace
trace = go.Scatter(
x = df_result_exp5_pfba.loc[rxn_mb_transport,:].index.str.split('_',expand=True).get_level_values(1),
y = df_result_exp5_pfba.loc[rxn_mb_transport,c4_mode_r_id],
mode = 'markers',
name = c4_mode_r_id,
error_y = dict(
type = 'data',
symmetric = False,
array = df_result_exp5_pfva.loc[rxn_mb_transport,c4_mode_r_id].apply(lambda x: x[1]) - df_result_exp5_pfba.loc[rxn_mb_transport,c4_mode_r_id],
arrayminus = df_result_exp5_pfba.loc[rxn_mb_transport,c4_mode_r_id] - df_result_exp5_pfva.loc[rxn_mb_transport,c4_mode_r_id].apply(lambda x: x[0]),
)
)
#Add trace
fig_exp5.add_trace(trace,i+1,1)
#Add annotation - postive flux: transport from mesophyll to bundle sheath
anno_ms2bs = dict(
x=-1,
y=25,
xref=f"x{i+1}",
yref=f"y{i+1}",
text= u"[M] \u2192 [BS]",
textangle= -90,
showarrow=False,
font=dict(
size=6,
color='#000000'
),
align='center',
)
fig_exp5.add_annotation(anno_ms2bs)
#Add annotation - negative flux: transport from bundle sheath to mesophyll
anno_ms2bs = dict(
x=-1,
y=-25,
xref=f"x{i+1}",
yref=f"y{i+1}",
text= '[BS] \u2192 [M]',
textangle= -90,
showarrow=False,
font=dict(
size=6,
color='#000000'
),
align='center',
)
fig_exp5.add_annotation(anno_ms2bs)
#Update xaxes
fig_exp5.update_xaxes(
tickangle = 35,
tickfont=dict(size=16)
)
#Update yaxes
fig_exp5.update_yaxes(
range = [-50, 50],
tickfont=dict(size=16)
)
#Update layout
fig_exp5.update_layout(
width = 1000,
height = 1000,
showlegend = False
)
#Update annotations
fig_exp5.update_annotations(font=dict(size=18))
#Show figure
fig_exp5.show()
Flux variability analysis of metabolite exchange with 1.5% deviation of the total flux minimum. The upper bar defines the maximum exchange flux, while the lower bar defines the minimum exchange flux, points indicate the value of the original flux solution under minimal metabolic effort constraint. Positive flux values correspond to the transport direction from mesophyll to bundle sheath, negative values to the transport direction from bundle sheath to mesophyll.
null
######################################################################################################################################
######################################################################################################################################
############## EXPERIMENT 6: Effect of PPFD and Photon Distribution betwenn Mesophyll and Bundle Sheath ##############
######################################################################################################################################
######################################################################################################################################
display(HTML(f"<h1>Code Cell 35: Experiment 6 -- Effect of PPFD and Photon Distribution betwenn Mesophyll and Bundle Sheath</h1>"))
#Remove original constraints on PPFD from c4 model
c4_model.remove_cons_vars(const_hnu_sum)
c4_model.remove_cons_vars(const_hnu_ratio)
#Create copy of c4 model
c4_model_exp6 = c4_model.copy()
#Reaction variables
B_Ex_Suc = c4_model_exp6.reactions.get_by_id("[B]_Ex_Suc")
M_Im_hnu = c4_model_exp6.reactions.get_by_id("[M]_Im_hnu")
B_Im_hnu = c4_model_exp6.reactions.get_by_id("[B]_Im_hnu")
B_RBO = c4_model_exp6.reactions.get_by_id("[B]_RBO_h")
M_RBO = c4_model_exp6.reactions.get_by_id("[M]_RBO_h")
#Decarboxylation reactions ids and names of enzymes
c4_mode_r_id_enzyme = {'[B]_MalDH4_h': 'NADP-ME', '[B]_PEPC1_c': 'PEP-CK', '[B]_MalDH2_m': 'NAD-ME', }
#Porportions of light hitting the mesophyll
#ds_prop_light_m = pd.Series(np.arange(0.5,10.1,0.1))
ds_prop_light_m = pd.Series(np.concatenate((np.arange(0.5,1.,0.1, dtype='float64'),np.arange(1,11,1)),axis = 0))
min_ppfd_bs_m = 1/ds_prop_light_m.max()
max_ppfd_bs_m = 1/ds_prop_light_m.min()
#Maximum light uptake
hnu_max = 1000
#Total PPFD
#ds_ppfd = pd.Series(np.arange(0,hnu_max+50,50))
ds_ppfd = pd.Series(sorted(np.concatenate((np.array([0,50,150]),np.arange(100,hnu_max+100,100)), axis=None)))
#Initialise dataframes to hold results
light_index = pd.MultiIndex.from_product([ds_ppfd.values,ds_prop_light_m.values, ], names=["PPFD", "prop_light_M"])
df_result_exp6 = pd.DataFrame(index=c4_model.reactions.list_attr('id'), columns=light_index, dtype='float64')
#Test all combinations of PPFD and photon distribution
for light in tqdm(light_index):
#Add constraint on PPFD
ppfd = light[0]
const_hnu1_exp6 = c4_model_exp6.problem.Constraint(
1.0 * M_Im_hnu.flux_expression + 1.0 * B_Im_hnu.flux_expression,
lb = float(ppfd),
ub = float(ppfd),
name = 'const_hnu1_exp6',
)
c4_model_exp6.add_cons_vars(const_hnu1_exp6)
#Add contraint on light distribution
prop = light[1]
const_hnu2_exp6 = set_fixed_flux_ratio({'[M]_Im_hnu':prop,'[B]_Im_hnu':1}, 'const_hnu2_exp6', c4_model_exp6)
#Optimization - Maximize sucrose output & Minimize Oxygenation by Rubisco
B_Ex_Suc.objective_coefficient = 1.
B_RBO.objective_coefficient = -1.
M_RBO.objective_coefficient = -1.
result_exp6_fba = c4_model_exp6.optimize('maximize')
#Optimize/Minimize total flux
if result_exp6_fba.status == 'optimal':
result_exp6_pfba = cobra.flux_analysis.parsimonious.pfba(c4_model_exp6)
df_result_exp6[light] = result_exp6_pfba.fluxes
#Remove light constraints
c4_model_exp6.remove_cons_vars(const_hnu1_exp6)
c4_model_exp6.remove_cons_vars(const_hnu2_exp6)
#Add original constraints on PPFD back to c4 model
c4_model.add_cons_vars(const_hnu_sum)
c4_model.add_cons_vars(const_hnu_ratio)
#Transpose results
df_result_exp6 = df_result_exp6.T
Code Cell 35: Experiment 6 -- Effect of PPFD and Photon Distribution betwenn Mesophyll and Bundle Sheath
0%| | 0/195 [00:00<?, ?it/s]
/Users/blaetke/opt/anaconda3/envs/elife-49305-era/lib/python3.9/site-packages/cobra/util/solver.py:508: UserWarning:
Solver status is 'infeasible'.
To analyse the effect of other conditions on the particular C4 state, we apply the minimisation of photorespiration as an additional objective to minimal total flux. Since NAD-ME and PEP-CK type plants use amino acids as transfer acids in nature, nitrogen availability has been tagged as a possible evolutionary constraint that selects for decarboxylation by NAD-ME or PEP-CK. When nitrate uptake was limiting, the optimal solution to the model predicted overall reduced flux towards the phloem output (Figure 8—figure supplement 1) but reactions were predicted to occur in the same proportions as predicted for unlimited nitrate uptake. Flux through NADP-ME and supplementary flux through PEP-CK dropped proportionally, since restricting nitrogen limits the export of all metabolites from the system and reduced CO2 uptake is observed (Figure 8—figure supplement 1). Similarly, limiting water or CO2 uptake into the model resulted in overall reduced flux towards the phloem output, see Figure 8—figure supplement 2 and Figure 8—figure supplement 3, but reactions were predicted to occur in the same proportions as predicted for unlimited uptake.
########################################################
############## FIGURE 7 ##############
########################################################
#{
# "caption": "### Effect of total PPFD on CO~2~ uptake rate in C4 mode.",
# "id": "fig7",
# "label": "Figure 7.",
# "trusted": true
#}
#Create figure
fig_exp61 = go.Figure()
#Create trace for co2 flux
trace = go.Scatter(
y = df_result_exp6.mean(axis=0, level='PPFD')['[M]_Im_CO2'],
x = df_result_exp6.index.get_level_values(0).unique(),
error_y=dict(
array = df_result_exp6.var(axis=0, level='PPFD')['[M]_Im_CO2']
)
)
#Add trace to figure
fig_exp61.add_trace(trace)
#Update yaxes
fig_exp61.update_yaxes(
title = dict(text='Flux [µmol/(m\u00B2s)]',font=dict(size=18)),
tickfont=dict(size=16)
)
#Update xaxes
fig_exp61.update_xaxes(
title = dict(text='PPFD [µE]',font=dict(size=18)),
tickangle = 35,
tickfont=dict(size=16),
#type='category'
)
#Update layout
fig_exp61.update_layout(
width = 1000,
height = 500,
title = dict(text='<b>CO\u2082 Uptake</b>', font=dict(size=20), x=0.5)
)
#Show figure
fig_exp61.show()
Effect of total PPFD on CO2 uptake rate in C4 mode.
########################################################
############## FIGURE 8 ##############
########################################################
#{
# "caption": "### Effect of light on the C4 mode. Heat-maps illustrating the activity of the decarboxylation enzymes PEP-CK, NADP-ME, and NAD-ME relative to the CO~2~ uptake rate in dependence of the total PPFD and the photon distribution among mesophyll and bundle sheath.",
# "id": "fig8",
# "label": "Figure 8.",
# "trusted": true
#}
#Create figure with subplot
fig_exp62 = make_subplots(
rows=3,
cols=1,
subplot_titles = [f"<b>{enzyme}</b>" for enzyme in c4_mode_r_id_enzyme.values()],
y_title= 'PPFD [BS] : PPFD [M]',
x_title = 'Total PPFD [µE]',
vertical_spacing = 0.1
)
#Get co2 flux for all combinations
df_im_co2 = df_result_exp6['[M]_Im_CO2'].reset_index().pivot_table(index='PPFD', columns='prop_light_M', aggfunc='mean')
#Get low light threshold
low_light_thres = df_im_co2[((df_im_co2.round(1) < df_im_co2.round(1).max().max()).sum(axis=1) != 0)].index.max()
#Save heatmap dataframes
dfs_r_id_co2 = {}
#Add subplot for each decarboxylation enzyme
for i, r_id in enumerate(c4_mode_r_id_enzyme.keys()):
#Get decarboxylation flux of current enzyme for all combinations
df_r_id = df_result_exp6[r_id].reset_index().pivot_table(index='PPFD', columns='prop_light_M', aggfunc='mean')
dfs_r_id_co2[r_id] = pd.DataFrame(df_r_id.values/df_im_co2.values, index = df_r_id.index, columns = df_r_id.columns)
#Create trace
trace = go.Heatmap(
z = (df_r_id.values/df_im_co2.values).T,
x = df_im_co2.index,
y = [round(value,1) for value in df_im_co2.columns.get_level_values(1)],
colorbar = dict(
title = dict(
text = 'Decarboxylation Rate : CO\u2082 Uptake Rate',
font = dict(size=18)
),
xpad = 20,
titleside = 'right'
),
zmin = 0,
zmax = 1,
name= c4_mode_r_id_enzyme[r_id],
showscale = True if i == 0 else False,
)
#Add trace to figure
fig_exp62.append_trace(trace,i+1,1)
#Max percentage of decarboylation by NADPME under low light
nadpme_max_low_light = dfs_r_id_co2['[B]_MalDH4_h'].loc[:low_light_thres].max().max().round(2) * 100
#Update yaxes
fig_exp62.update_yaxes(
tickprefix= '1:',
tickfont=dict(size=16),
type='category'
)
#Update xaxes
fig_exp62.update_xaxes(
tickangle = 35,
tickvals=df_im_co2.index,
tickfont=dict(size=16),
type='category'
)
#Update annotations
fig_exp62.update_annotations(
font=dict(size=20)
)
#Update layout
fig_exp62.update_layout(
width = 600,
height = 1000
)
#Show figure
fig_exp62.show()
Effect of light on the C4 mode. Heat-maps illustrating the activity of the decarboxylation enzymes PEP-CK, NADP-ME, and NAD-ME relative to the CO2 uptake rate in dependence of the total PPFD and the photon distribution among mesophyll and bundle sheath.
Given that C4 plants sometimes optimise light availability to the bundle sheath 8Bellasio and Lundgren2016 we next explored light availability and light distribution. The model prediction is re-run with changes in the constraints, and the resulting tables of fluxes are queried for CO2 uptake and fluxes through the decarboxylation enzymes. In the experiment, we varied the total PPFD between {{dsppfd.min()}} μmol/(m2s) to {{ds_ppfd.max()}} μmol/(m2s) and photon distribution $PPF{D}$ / in the range between {{minppfd_bs_m}} to {{max_ppfd_bs_m}}, see Figure 7 and Figure 8. Under light limitation, if the total PPFD is lower than {{low_light_thres}} μmol/(m2s) , the CO2 uptake rate is reduced, leading to a decreased activity of the decarboxylation enzymes (Figure 7). PEP-CK is used in the optimal solutions active under light-limiting conditions (Figure 8). Under limiting light conditions, photon distribution with a higher proportion in the bundle sheath shifts decarboxylation towards NADP-ME but only to up to {{nadpme_max_low_light}}%. Under non-limiting conditions, the distribution of light availability determines the optimal decarboxylation enzyme. NADP-ME is the preferred decarboxylation enzyme with supplemental contributions by PEP-CK if light availability is near the threshold of {{low_light_thres}} μmol/(m2s) or if at least twice as many photons are absorbed by the mesophyll. Excess light availability and a higher proportion of photons reaching the bundle sheath leads to optimal solutions which favour PEP-CK as the decarboxylation enzyme. In the case of very high light availability and an abrupt shift towards the bundle sheath, NAD-ME becomes the optimal solution (Figure 8). NAD-ME is the least favourable enzyme overall, only low activity is predicted under extreme light conditions, where the bundle sheath absorbs equal or more photons than the mesophyll (Figure 8). PEP-CK complements the activity of NADP-ME and NAD-ME to 100% in many conditions, meaning the _two-cell model also predicts the co-existence of PEP-CK/NADP-ME and PEP-CK/NAD-ME mode, while the flux distribution indicates no parallel use of NAD-ME and NADP-ME, compare Figure 8.
null
######################################################################################################################################
######################################################################################################################################
############## EXPERIMENT 7: Effect of N-Limtation on C4 Mode ##############
######################################################################################################################################
######################################################################################################################################
display(HTML(f"<h1>Code Cell 38: Experiment 7 -- Effect of N-Limtation on C4 Mode</h1>"))
#Create copy of c4 model
c4_model_exp7 = c4_model.copy()
#Reaction variables
B_Ex_Suc = c4_model_exp7.reactions.get_by_id("[B]_Ex_Suc")
M_Im_NO3 = c4_model_exp7.reactions.get_by_id("[M]_Im_NO3")
B_Im_NO3 = c4_model_exp7.reactions.get_by_id("[B]_Im_NO3")
B_RBO = c4_model_exp7.reactions.get_by_id("[B]_RBO_h")
M_RBO = c4_model_exp7.reactions.get_by_id("[M]_RBO_h")
#Decarboxylation reactions ids and names of enzymes
c4_mode_r_id_enzyme = {'[B]_MalDH2_m': 'NAD-ME', '[B]_PEPC1_c': 'PEP-CK', '[B]_MalDH4_h': 'NADP-ME'}
#NO3 flux
no3_flux = np.arange(0,2.1,0.2)
#Initialise dataframes to hold results
df_result_exp7 = pd.DataFrame(index=c4_model.reactions.list_attr('id'), columns=no3_flux, dtype='float64')
#Test all no3 values
for no3_value in tqdm(df_result_exp7.columns):
#Add N-limitation constraint
const_NO3_exp7 = c4_model_exp7.problem.Constraint( M_Im_NO3.flux_expression + B_Im_NO3.flux_expression,
lb = no3_value, ub = no3_value, name='const_NO3_exp7')
c4_model_exp7.add_cons_vars(const_NO3_exp7)
#Optimization - Maximize sucrose output & Minimize Oxygenation by Rubisco
B_Ex_Suc.objective_coefficient = 1.
B_RBO.objective_coefficient = -1.
M_RBO.objective_coefficient = -1.
result_exp7_fba = c4_model_exp7.optimize('maximize')
#Optimize/Minimize total flux
if result_exp7_fba.status == 'optimal':
result_exp7_pfba = cobra.flux_analysis.parsimonious.pfba(c4_model_exp7)
df_result_exp7[no3_value] = result_exp7_pfba.fluxes
#Remove N-limitation constraint
c4_model_exp7.remove_cons_vars(const_NO3_exp7)
Code Cell 38: Experiment 7 -- Effect of N-Limtation on C4 Mode
0%| | 0/11 [00:00<?, ?it/s]
/Users/blaetke/opt/anaconda3/envs/elife-49305-era/lib/python3.9/site-packages/cobra/util/solver.py:508: UserWarning:
Solver status is 'infeasible'.
##################################################################################
############## Figure 8—figure supplement 1 ##############
##################################################################################
#{
# "caption": "### Effect of NO~3~^-^ limitation on the flux through the different decarboxylation enzymes.",
# "id": "fig8s1,
# "label": "Figure 8—figure supplement 1",
# "trusted": true
#}
#Create figure
fig_exp7 = go.Figure()
#Add trace for each decarboxylation enzyme
for i, r_id in enumerate(c4_mode_r_id_enzyme.keys()):
#Create bar plot trace
trace = go.Bar(
y = df_result_exp7.loc[r_id],
x = df_result_exp7.columns,
name = c4_mode_r_id_enzyme[r_id]
)
#Add trace to figure
fig_exp7.add_trace(trace)
#Update xaxes
fig_exp7.update_xaxes(
title = dict(
text = 'NO\u2083 Uptake Rate [µmol/(m\u00B2s)]',
font = dict(size=18)
),
tickfont = dict(size=16)
)
#Update yaxes
fig_exp7.update_yaxes(
range = [0, 40],
title = dict(
text = 'Flux [µmol/(m\u00B2s)]',
font = dict(size=18)
),
tickfont = dict(size=16)
)
#Update layout
fig_exp7.update_layout(
barmode='stack',
width= 1000,
height=500,
legend=dict(
font=dict(size=18),
)
)
#Show figure
fig_exp7.show()
Effect of NO3- limitation on the flux through the different decarboxylation enzymes.
######################################################################################################################################
######################################################################################################################################
############## EXPERIMENT 8: Effect of H2O-Limtation on C4 Mode ##############
######################################################################################################################################
######################################################################################################################################
display(HTML(f"<h1>Code Cell 40: Experiment 8 -- Effect of H2O-Limtation on C4 Mode</h1>"))
#Create copy of c4 model
c4_model_exp8 = c4_model.copy()
#Reaction variables
B_Ex_Suc = c4_model_exp8.reactions.get_by_id("[B]_Ex_Suc")
M_Im_H2O = c4_model_exp8.reactions.get_by_id("[M]_Im_H2O")
B_Im_H2O = c4_model_exp8.reactions.get_by_id("[B]_Im_H2O")
B_RBO = c4_model_exp8.reactions.get_by_id("[B]_RBO_h")
M_RBO = c4_model_exp8.reactions.get_by_id("[M]_RBO_h")
#Decarboxylation reactions ids and names of enzymes
c4_mode_r_id_enzyme = {'[B]_MalDH2_m': 'NAD-ME', '[B]_PEPC1_c': 'PEP-CK', '[B]_MalDH4_h': 'NADP-ME'}
#H2O flux
h2O_flux = np.arange(0,45,5)
#Initialise dataframes to hold results
df_result_exp8 = pd.DataFrame(index=c4_model.reactions.list_attr('id'), columns=h2O_flux, dtype='float64')
#Test all H2O values
for h2o_value in tqdm(df_result_exp8.columns):
#Add max H2O-uptake constraint
const_h2o_exp8 = c4_model_exp8.problem.Constraint( M_Im_H2O.flux_expression + B_Im_H2O.flux_expression,
lb = 0, ub = h2o_value, name='const_h2o_exp8')
c4_model_exp8.add_cons_vars(const_h2o_exp8)
#Optimize/Maximize sucrose output
B_Ex_Suc.objective_coefficient = 1.
B_RBO.objective_coefficient = -1.
M_RBO.objective_coefficient = -1.
result_exp8_fba = c4_model_exp8.optimize('maximize')
#Optimize/Minimize total flux
if result_exp8_fba.status == 'optimal':
result_exp8_pfba = cobra.flux_analysis.parsimonious.pfba(c4_model_exp8)
df_result_exp8[h2o_value] = result_exp8_pfba.fluxes
#Remove max H2O-uptake constraint
c4_model_exp8.remove_cons_vars(const_h2o_exp8)
Code Cell 40: Experiment 8 -- Effect of H2O-Limtation on C4 Mode
0%| | 0/9 [00:00<?, ?it/s]
##################################################################################
############## Figure 8—figure supplement 2 ##############
##################################################################################
#{
# "caption": "### Effect of H~2~O imitation on the flux through the different decarboxylation enzymes.",
# "id": "fig8s2",
# "label": "Figure 8—figure supplement 2",
# "trusted": true
#}
#Create figure
fig_exp8 = go.Figure()
#Add trace for each decarboxylation enzyme
for i, r_id in enumerate(c4_mode_r_id_enzyme.keys()):
#Create bar plot trace
trace = go.Bar(
y = df_result_exp8.loc[r_id],
x = df_result_exp8.columns,
name = c4_mode_r_id_enzyme[r_id]
)
#Add trace to figure
fig_exp8.add_trace(trace)
#Update xaxes
fig_exp8.update_xaxes(
title = dict(
text = 'Max H\u2082O Uptake Rate [µmol/(m\u00B2s)]',
font = dict(size=18)
),
tickfont = dict(size=16)
)
#Update yaxes
fig_exp8.update_yaxes(
range = [0, 40],
title = dict(
text = 'Flux [µmol/(m\u00B2s)]',
font = dict(size=18)
),
tickfont = dict(size=16)
)
#Update layout
fig_exp8.update_layout(
barmode='stack',
width= 1000,
height=500,
legend=dict(
font=dict(size=18),
)
)
#Show figure
fig_exp8.show()
Effect of H2O imitation on the flux through the different decarboxylation enzymes.
######################################################################################################################################
######################################################################################################################################
############## EXPERIMENT 9: Effect of CO2-Limtation on C4 Mode ##############
######################################################################################################################################
######################################################################################################################################
display(HTML(f"<h1>Code Cell 42: Experiment 9 -- Effect of CO2-Limtation on C4 Mode</h1>"))
#Create copy of c4 model
c4_model_exp9 = c4_model.copy()
#Reaction variables
B_Ex_Suc = c4_model_exp9.reactions.get_by_id("[B]_Ex_Suc")
M_Im_CO2 = c4_model_exp9.reactions.get_by_id("[M]_Im_CO2")
B_Im_CO2 = c4_model_exp9.reactions.get_by_id("[B]_Im_CO2")
B_RBO = c4_model_exp9.reactions.get_by_id("[B]_RBO_h")
M_RBO = c4_model_exp9.reactions.get_by_id("[M]_RBO_h")
#Decarboxylation reactions ids and names of enzymes
c4_mode_r_id_enzyme = {'[B]_MalDH2_m': 'NAD-ME', '[B]_PEPC1_c': 'PEP-CK', '[B]_MalDH4_h': 'NADP-ME'}
#H2O flux
co2_flux = np.arange(0,45,5)
#Initialise dataframes to hold results
df_result_exp9 = pd.DataFrame(index=c4_model.reactions.list_attr('id'), columns=co2_flux, dtype='float64')
#Test all CO2 values
for co2_value in tqdm(df_result_exp9.columns):
#Add max CO2-uptake constraint
const_co2_exp9 = c4_model_exp9.problem.Constraint( M_Im_CO2.flux_expression + B_Im_CO2.flux_expression,
lb = 0, ub = co2_value, name='const_co2_exp9')
c4_model_exp9.add_cons_vars(const_co2_exp9)
#Optimize/Maximize sucrose output
B_Ex_Suc.objective_coefficient = 1.
B_RBO.objective_coefficient = -1.
M_RBO.objective_coefficient = -1.
result_exp9_fba = c4_model_exp9.optimize('maximize')
#Optimize/Minimize total flux
if result_exp9_fba.status == 'optimal':
result_exp9_pfba = cobra.flux_analysis.parsimonious.pfba(c4_model_exp9)
df_result_exp9[co2_value] = result_exp9_pfba.fluxes
#Remove max CO2-uptake constraint
c4_model_exp9.remove_cons_vars(const_co2_exp9)
Code Cell 42: Experiment 9 -- Effect of CO2-Limtation on C4 Mode
0%| | 0/9 [00:00<?, ?it/s]
##################################################################################
############## Figure 8—figure supplement 3 ##############
##################################################################################
#{
# "caption": "### Effect of CO~2~ limitation on the flux through the different decarboxylation enzymes.",
# "id": "fig8s3",
# "label": "Figure 8—figure supplement 3",
# "trusted": true
#}
#Create figure
fig_exp9 = go.Figure()
#Add trace for each decarboxylation enzyme
for i, r_id in enumerate(c4_mode_r_id_enzyme.keys()):
#Create bar plot trace
trace = go.Bar(
y = df_result_exp9.loc[r_id],
x = df_result_exp9.columns,
name = c4_mode_r_id_enzyme[r_id]
)
#Add trace to figure
fig_exp9.add_trace(trace)
#Update xaxes
fig_exp9.update_xaxes(
title = dict(
text = 'Max CO\u2082 Uptake Rate [µmol/(m\u00B2s)]',
font = dict(size=18)
),
tickfont = dict(size=16)
)
#Update yaxes
fig_exp9.update_yaxes(
range = [0, 40],
title = dict(
text = 'Flux [µmol/(m\u00B2s)]',
font = dict(size=18)
),
tickfont = dict(size=16)
)
#Update layout
fig_exp9.update_layout(
barmode='stack',
width= 1000,
height=500,
legend=dict(
font=dict(size=18),
)
)
#Show figure
fig_exp9.show()
Effect of CO2 limitation on the flux through the different decarboxylation enzymes.
######################################################################################################################################
######################################################################################################################################
############## EXPERIMENT 10: Effect of Malate/Aspartate-Exchange Ratio on C4 Mode ##############
######################################################################################################################################
######################################################################################################################################
display(HTML(f"<h1>Code Cell 46: Experiment 10 -- Effect of Malate/Aspartate-Exchange Ratio on C4 Mode</h1>"))
#Create copy of c4 model
c4_model_exp10 = c4_model.copy()
#Reaction variables
B_Ex_Suc = c4_model_exp10.reactions.get_by_id("[B]_Ex_Suc")
B_RBO = c4_model_exp10.reactions.get_by_id("[B]_RBO_h")
M_RBO = c4_model_exp10.reactions.get_by_id("[M]_RBO_h")
#Decarboxylation reactions ids and names of enzymes
c4_mode_r_id_enzyme = {'[B]_MalDH2_m': 'NAD-ME', '[B]_PEPC1_c': 'PEP-CK', '[B]_MalDH4_h': 'NADP-ME'}
#Max exchange flux
ex_flux = 40
#Malate flux
mal_flux = np.arange(0,45,5)
#Initialise dataframes to hold results
df_result_exp10 = pd.DataFrame(index=c4_model.reactions.list_attr('id'), columns=mal_flux, dtype='float64')
#Test all mal/asp exchange values
for mal_value in tqdm(df_result_exp10.columns):
#Add Mal/Asp transport constraint
set_fixed_flux('[MB]_Mal_c',mal_value, c4_model_exp10)
set_fixed_flux('[MB]_Asp_c',ex_flux - mal_value, c4_model_exp10)
#Optimize/Maximize sucrose output
B_Ex_Suc.objective_coefficient = 1.
B_RBO.objective_coefficient = -1.
M_RBO.objective_coefficient = -1.
result_exp10_fba = c4_model_exp10.optimize('maximize')
#Optimize/Minimize total flux
if result_exp10_fba.status == 'optimal':
result_exp10_pfba = cobra.flux_analysis.parsimonious.pfba(c4_model_exp10)
df_result_exp10[mal_value] = result_exp10_pfba.fluxes
Code Cell 46: Experiment 10 -- Effect of Malate/Aspartate-Exchange Ratio on C4 Mode
0%| | 0/9 [00:00<?, ?it/s]
##################################################################################
############## Figure 8—figure supplement 4 ##############
##################################################################################
#{
# "caption": "### Effect of malate:aspartate transport ratio on the flux through the different decarboxylation enzymes.",
# "id": "fig8s4",
# "label": "Figure 8—figure supplement 4",
# "trusted": true
#}
#Create Figure
fig_exp10 = go.Figure()
#Add Trace for each decarboxylation enzyme
for i, r_id in enumerate(c4_mode_r_id_enzyme.keys()):
#Create bar plot trace
trace = go.Bar(
y = df_result_exp10.loc[r_id],
x = df_result_exp10.columns,
name = c4_mode_r_id_enzyme[r_id]
)
#Add trace to figure
fig_exp10.add_trace(trace)
#Update xaxes
fig_exp10.update_xaxes(
tickvals = df_result_exp10.columns,
ticktext = [f'{mal_value} : {ex_flux - mal_value}' for mal_value in df_result_exp10.columns],
title = dict(
text = 'Mal : Asp Exchange',
font = dict(size=18)
),
tickfont = dict(size=16)
)
#Update yaxes
fig_exp10.update_yaxes(
range = [0, 40],
title = dict(
text = 'Flux [µmol/(m\u00B2s)]',
font = dict(size=18)
),
tickfont = dict(size=16)
)
#Update layout
fig_exp10.update_layout(
barmode='stack',
width= 1000,
height=500,
legend=dict(
font=dict(size=18),
)
)
#Show figure
fig_exp10.show()
Effect of malate:aspartate transport ratio on the flux through the different decarboxylation enzymes.
null
Finally, we assumed that intercellular transport capacity for charged metabolites might be different between species. Assuming a fixed transport ratio between aspartate and malate (Figure 8—figure supplement 4) introduces a shift in the C4 state. Higher proportions of malate exchange foster the use of NADP-ME (Figure 8—figure supplement 4). In contrast, higher portions of aspartate exchange foster the use of PEP-CK (Figure 8—figure supplement 4).
Discussion
Evolutionary CBM can suggest the molecular outcomes of past evolutionary events if models are parametrised with objective functions representing possible selective pressures. In the case of C4 photosynthesis, more than sixty independent evolutionary origins represent metabolic types characterised by their decarboxylation enzyme. The selective pressure which drives evolution towards one or the other flux are unknown and were tested using CBM.
One-cell model reflects C3 plant physiology
To analyse evolution towards C4 photosynthesis based on C3 metabolism, a CBM of C3 metabolism is required (Figure 1). Design, simulation, validation cycles used current knowledge about plant biochemistry 32Heldt2015 to identify possible errors in the metabolic map required for modelling. Even after error correction (Table 1), a significant problem remained, namely excessive fluxes to balance protons in all compartments. This observation leads to the realisation that the biochemical knowledge about transport reactions does not extend to the protonation state of the substrates, which affects all eukaryotic CBM efforts. In plants, predominantly export and vacuolar transport reactions are directly or indirectly coupled with proton gradients to energise transport 16Bush199350Neuhaus2007. For chloroplasts and mitochondria, proton-coupled transport reactions have been described but may couple different metabolite transporters together rather than energising them 26Furumoto et al.2011. Introducing proton sinks in all compartments solves the immediate modelling problem. However, intracellular transport reactions and their energetic costs are no longer correctly assessed by the model. Despite this band-aid fix which will be required for all eukaryotic constraint-based models which include proton-coupled transport reactions, the curated one-cell model correctly predicts energy usage and its distribution (Figure 1—figure supplement 3, Figure 1—figure supplement 4 and 40Li et al.2017). This indicates that in models which exclude vacuolar transport and energised export reactions, energy calculations remain likely within the correct order of magnitude. Overall, our one-cell model operates within parameters expected for a C3 plant: The predicted PPFD lies within the range of light intensities used for normal growth condition of Arabidopsis thaliana, which varies between 100 μmol/(m2s)–200 μmol/(m2s), see Table 2. The gross rate of O2 evolution for a PPFD of 200 μmol/(m2s) is estimated to be 16.5 μmol/(m2s) in the literature 75Sun et al.1999, which is in close proximity to the predicted flux of the one-cell model, see Table 2. For the amount of respiratory ATP that is used for maintenance, 40Li et al.2017 predicted an even lower proportion of energy 16%, see Figure 1—figure supplement 4(B). The model’s flux map is in accordance with known C3 plant physiology 32Heldt2015, and its input and output parameters match expected values (Figure 2(B)). The current model excludes specialised metabolism since the output function focuses solely on substances exported through the phloem in a mature leaf. If the model were to be used to study biotic interactions in the future, the addition of specialised metabolism in the metabolic map and a new output function would be required.
The two-cell model predicts a C4 cycle if photorespiration is present
Most evolutionary concepts about C4 photosynthesis assume that selective pressure drives pathway evolution due to photorespiration and carbon limitation 31Heckmann et al.2013. Most extant C4 species occupy dry and arid niches 23Edwards et al.2010, even more, the period of C4 plant evolution was accompanied with an increased oxygen concentration in the atmosphere 62Sage2004. Therefore, it is frequently assumed that carbon limitation by excessive photorespiration drives the evolution of C4 photosynthesis. Yet, in most habitats plants are limited by nutrients other than carbon 1Agren et al.201236Körner2015. Ecophysiological analyses also show that C4 can evolve in non-arid habitats 43Liu and Osborne201544Lundgren and Christin201753Osborne and Freckleton2009. To resolve this apparent contradiction, we tested whether resource limitation may also lead to the evolution of a C4 cycle. We optimised the model approximating resource limitation via an objective function for total minimal flux at different photorespiratory levels. Indeed, with increasing photorespiration, the optimisation for resource efficiency leads to the emergence of the C4 cycle as the optimal solution. Balancing the resource cost of photorespiration against the resource cost of the C4 cycle, the model predicts that N limitation may have facilitated C4 evolution given high levels of photorespiration. Other possible selective pressures such as biotic interactions can currently not be tested using the model since specialised metabolism is not included in the metabolic map or the output function. Extant C4 species have higher C : N ratios reflecting the N-savings the operational C4 cycle enables 61Sage et al.1987. The photorespiratory pump using glycine decarboxylase based CO2 enrichment also emerges from the model, showing that C2 photosynthesis is also predicted under simple resource limitation. Indeed N-savings have been reported from C2 plants compared with their C3 sister lineages 66Schlüter et al.2016. Simply minimising photorespiration as the objective function also yields C4 photosynthesis as the optimal solution. Hence, two alternatively or parallelly acting selective pressures towards C4 photosynthesis, limitation in C and/or N, are identified by the model. In both cases, the model correctly predicts the C4 cycle of carboxylation and decarboxylation and the C2 photorespiratory pump as observed in extant plants. The evolution of C4 photosynthesis in response to multiple selective pressures underscores its adaptive value and potential for agriculture. Intermediacy also evolves indicating that it, too, is likely an added value trait which could be pursued by breeding and engineering efforts.
The optimal solutions for the metabolic flux patterns predict an intermediate stage in which CO2 transport via photorespiratory intermediates glycolate and glycerate (Figure 4) and decarboxylation by glycine decarboxylase complex (Figure 3(B)) is essential. All of the models of C4 evolution 48Monson19996Bauwe201063Sage et al.201231Heckmann et al.201386Williams et al.2013 predict that the establishment of a photorespiratory CO2 pump is an essential intermediate step towards the C4 cycle. The photorespiratory CO2 pump, also known as C2 photosynthesis, relocates the photorespiratory CO2 release to the bundle sheath cells. Plants using the photorespiratory CO2 pump are often termed C3-C4 intermediates owing to their physiological properties 63Sage et al.2012. Displaying the flux solution in Figure 3 (A) -(D) and Figure 4 on a metabolic map in Figure 3—figure supplement 1 clearly illustrates that increasing photorespiratory flux through Rubisco drives the two-cell metabolic model from C3 to C4 metabolism by passing the C3-C4 intermediate state. On the C3-C4 trajectory, the activity of Rubisco is shifted from the mesophyll to the bundle sheath, as well as from the constrained to the CCM-dependent Rubisco population as a consequence of the increased costs of photorespiration under increased ratio, see Equation 5. The increase of the oxygenation rate in the photorespiration constraint drives the reprogramming of the metabolism to avoid oxygenation by establishing the C4 cycle. Therefore, our analysis recovers the evolutionary C3-C4 trajectory and confirms the emergence of a photorespiratory CO2 pump as an essential step during the C4 evolution also under optimisation for resources 31Heckmann et al.2013. The model may also provide a reason for why some plant species have halted their evolution in this intermediary phase 65Scheben et al.2017. Under the conditions of resource limitations and intermediate photorespiration, the model predicts intermediacy as the optimal solution. In a very narrow corridor of conditions, no further changes are required to reach optimality and the model thus predicts that a small number of species may remain intermediate.
Two-cell model realises different C4 states
Since the model predicts C4 metabolism without specific constraints, different input and reaction constraints can be tested for their influence on the molecular nature of the C4 cycle. This approach may identify the selective pressure and boundaries limiting evolution. Initial optimisation without additional constraints or input limitations predict a C4 cycle based on decarboxylation by NADP-ME (Figure 3 and Figure 3—figure supplement 1(A)). This prediction recapitulates intuition; the NADP-ME based C4 cycle is considered the 'most straight forward' incarnation of C4 photosynthesis, it is always explained first in textbooks and is a major focus of research. The NADP-ME based cycle thus represents the stoichiometrically optimal solution when resource limitation or photorespiration are considered. Once NADP-ME is no longer available via constraint, PEP-CK and NAD-ME become optimal solutions albeit with a prediction of malate and pyruvate as the transfer acids (Figure 8). The FVA identified aspartate and alanine as slightly less optimal solutions (Figure 6). Since in vivo this slightly less optimal solution has evolved in all NAD-ME origins tested to date, kinetic rather than stoichiometric reasons suggest themselves for the use of aspartate and alanine 13Bräutigam et al.2018.
Light is a potential evolutionary driver for the different C4 states
Since all extant C3 species and therefore also the ancestors of all C4 species contain all decarboxylation enzymes 4Aubry et al.2011, it is unlikely that unavailability of an enzyme is the reason for the evolution of different decarboxylation enzymes in different origins 62Sage2004. Stochastic processes during evolution, that is up-regulation of particular enzyme concentrations via changes in expression and therefore elements cis to the gene 14Bräutigam and Gowik2016, may have played a role in determining which C4 cycle evolved. Alternatively, environmental determinants may have contributed to the evolution of different C4 cycles. Physiological experiments have pointed to a connection between nitrogen use efficiency and type of decarboxylation enzyme 58Pinto et al.2016. Hence the variation in nitrogen input to the model was tested for their influence on optimal solutions with regard to decarboxylation enzymes. Input limitation of nitrogen, water as a metabolite, and CO2 limited the output of the system but did not change the optimal solution concerning decarboxylation, see Figure 8—figure supplement 1, Figure 8—figure supplement 2, and Figure 8—figure supplement 3, making it an unlikely candidate as the cause. Differences in nitrogen use is possibly a consequence of decarboxylation type.
In some grasses, light penetrable cells overlay the vascular bundle leading to different light availability (summarised in 8Bellasio and Lundgren2016 and 34Karabourniotis et al.2000) and hence light availability and distribution were tested (Figure 8). Changes in light input and distribution of light input between mesophyll and bundle sheath indeed altered the optimal solutions (Figure 8). The changes in the solution can be traced to the energy status of the plant cells. For very high light intensities, the alternative oxidases in the mitochondria are used to dissipate the energy and hence a path towards NAD-ME is paved. Under light limitation, the C4 cycle requires high efficiency and hence PEP-CK which, at least in part allows energy conservation by using PEP rather than pyruvate as the returning C4 acid, is favoured. Interestingly, the sensitivity of different species towards environmental changes in light is influenced by the decarboxylation enzyme present 72Sonawane et al.2018. NADP-ME species are less compromised compared to NAD-ME species by shade possibly reflecting an evolutionary remnant as NAD-ME is predicted to emerge only in high light conditions. PEP-CK is more energy efficient compared to malic enzyme based decarboxylation which requires PEP recycling by PPDK at the cost of two molecules of ATP (Figure 3(D)). Notably, two C4 plants known to rely on PEP-CK P. maximum and A. semialata (African accessions) are shade plants which grow in the understory 44Lundgren and Christin2017. PEP-CK can be co-active with NADP-ME and NAD-ME (Figure 8). This co-use of PEP-CK with a malic enzyme has been shown in C4 plants 57Pick et al.201187Wingler et al.1999 and explained as an adaptation to different energy availability and changes in light conditions 57Pick et al.20117Bellasio and Griffiths2014. Dominant use of PEP-CK in the absence of malic enzyme activity as suggested (Figure 3(B), Figure 3—figure supplement 1 and Figure 5) is rare in vivo080Ueno and Sentoku2006 but observed in P. maximum and in A. semialata. While the model predictions are in line with ecological observations, we cannot exclude that kinetic constraints (i.e. 13Bräutigam et al.2018) may also explain why a stoichiometrically optimal solution such as the NADP-ME cycle is not favoured in nature where NADP-ME and NAD-ME species evolve in nearly equal proportions 62Sage2004.
Conclusion
CBM of photosynthetically active plant cells revealed a major knowledge gap impeding CBM, namely the unknown protonation state of most transport substrates during intracellular transport processes. When photoautotrophic metabolism was optimised in a single cell for minimal metabolic flux and therefore, optimal resource use, C3 photosynthetic metabolism was predicted as the optimal solution. Under low photorespiratory conditions, a two-celled model which contains a CCM-dependent Rubisco optimised for resource use, still predicts C3 photosynthesis. However, under medium to high photorespiratory conditions, a molecularly correct C4 cycle emerged as the optimal solution under resource limitation and photorespiration reduction as objective functions which points to resource limitation as an additional driver of C4 evolution. Light and light distribution was the environmental variable governing the choice of decarboxylation enzymes. Modelling compartmented eukaryotic cells correctly predicts the evolutionary trajectories leading to extant C4 photosynthetic plant species.
References
-
- GIAgren
- JÅWetterstedt
- MFBillberger
- doi10.1111/j.1469-8137.2012.04116.x
- pmid22458659
-
- AArnold
- ZNikoloski
- doi10.1104/pp.114.235358
- pmid24808102
-
- MAshburner
- CABall
- JABlake
- DBotstein
- HButler
- JMCherry
- APDavis
- KDolinski
- SSDwight
- JTEppig
- MAHarris
- DPHill
- LIssel-Tarver
- AKasarskis
- SLewis
- JCMatese
- JERichardson
- MRingwald
- GMRubin
- GSherlock
- doi10.1038/75556
-
- SAubry
- NJBrown
- JMHibberd
- doi10.1093/jxb/err012
- pmid21321052
-
- SBailey
- RGWalters
- SJansson
- PHorton
- doi10.1007/s004250100556
- pmid11678285
-
- HBauwe
-
- CBellasio
- HGriffiths
- doi10.1104/pp.113.228221
- pmid24254314
-
- CBellasio
- MRLundgren
- doi10.1111/nph.14063
- pmid27375085
-
- MBerkemeyer
- RScheibe
- OOcheretina
- doi10.1074/jbc.273.43.27927
- pmid9774405
-
- MABlätke
-
- NRBoyle
- JAMorgan
- doi10.1186/1752-0509-3-4
- pmid19128495
-
- ABräutigam
- SSchliesky
- CKülahoglu
- CPOsborne
- APWeber
- doi10.1093/jxb/eru100
- pmid24642845
-
- ABräutigam
- USchlüter
- MRLundgren
- SFlachbart
- OEbenhöh
- GSchönknecht
- P-AChristin
- SBleuler
- J-MDroz
- COsborne
- AWeber
- UGowik
- doi10.1101/387431
-
- ABräutigam
- UGowik
- doi10.1093/jxb/erw056
- pmid26912798
-
- ABräutigam
- APMWeber
-
- DRBush
- doi10.1146/annurev.pp.44.060193.002501
-
- CYCheung
- MGPoolman
- DAFell
- RGRatcliffe
- LJSweetlove
- doi10.1104/pp.113.234468
- pmid24596328
-
- PAChristin
- EJEdwards
- GBesnard
- SFBoxall
- RGregory
- EAKellogg
- JHartwell
- CPOsborne
- doi10.1016/j.cub.2012.01.054
- pmid22342748
-
- CGdeOliveiraDal'Molin
- LEQuek
- RWPalfreyman
- SMBrumbley
- LKNielsen
- doi10.1104/pp.109.148817
- pmid20044452
-
- FDöring
- MStreubel
- ABräutigam
- UGowik
- doi10.1093/jxb/erw041
- pmid26976818
-
- NCDuarte
- doi10.1101/gr.2250904
-
- SMDuff
- TJRydel
- ALMcClerren
- WZhang
- JYLi
- EJSturman
- CHalls
- SChen
- JZeng
- JPeng
- CNKretzler
- AEvdokimov
- doi10.1016/j.abb.2012.06.006
- pmid22750542
-
- EJEdwards
- CPOsborne
- CAStrömberg
- SASmith
- WJBond
- PAChristin
- ABCousins
- MRDuvall
- DLFox
- RPFreckleton
- OGhannoum
- JHartwell
- YHuang
- CMJanis
- JEKeeley
- EAKellogg
- AKKnapp
- ADLeakey
- DMNelson
- JMSaarela
- RFSage
- OESala
- NSalamin
- CJStill
- BTipple
- C4 Grasses Consortium
- doi10.1126/science.1177216
- pmid20431008
-
- RJEllis
- doi10.1016/0968-0004(79)90212-3
-
- TAFeodorova
- EVVoznesenskaya
- GEEdwards
- EHRoalson
- doi10.1600/036364410X539880
-
- TFurumoto
- TYamaguchi
- YOhshima-Ichie
- MNakamura
- YTsuchida-Iwata
- MShimamura
- JOhnishi
- SHata
- UGowik
- PWesthoff
- ABräutigam
- APWeber
- KIzui
- doi10.1038/nature10250
- pmid21866161
-
- CGietl
- doi10.1016/0167-4838(92)90476-T
-
- CGomesdeOliveiraDal’Molin
- L-EQuek
- RWPalfreyman
- LKNielsen
- doi10.1186/1471-2164-12-S4-S5
-
- GFJHaberlandt
- V.erlagvonWEngelmann
-
- MDHatch
- doi10.1016/S0304-4173(87)80009-5
-
- DHeckmann
- SSchulze
- ADenton
- UGowik
- PWesthoff
- APWeber
- MJLercher
- doi10.1016/j.cell.2013.04.058
- pmid23791184
-
- HWHeldt
-
- EMJosse
- AJSimkin
- JGaffé
- AMLabouré
- MKuntz
- PCarol
- doi10.1104/pp.123.4.1427
- pmid10938359
-
- GKarabourniotis
- JFBornman
- DNikolopoulos
- doi10.1046/j.1365-3040.2000.00558.x
-
- TKiba
- ABFeria-Bourrellier
- FLafouge
- LLezhneva
- SBoutet-Mercey
- MOrsel
- VBréhaut
- AMiller
- FDaniel-Vedele
- HSakakibara
- AKrapp
- doi10.1105/tpc.111.092221
- pmid22227893
-
- CKörner
- doi10.1016/j.pbi.2015.05.003
- pmid26037389
-
- WLacher
-
- ADLeakey
- MUribelarrea
- EAAinsworth
- SLNaidu
- ARogers
- DROrt
- SPLong
- doi10.1104/pp.105.073957
- pmid16407441
-
- NELewis
- KKHixson
- TMConrad
- JALerman
- PCharusanti
- ADPolpitiya
- JNAdkins
- GSchramm
- SOPurvine
- DLopez-Ferrer
- KKWeitz
- REils
- RKönig
- RDSmith
- BØPalsson
- doi10.1038/msb.2010.47
- pmid20664636
-
- LLi
- CJNelson
- JTrösch
- ICastleden
- SHuang
- AHMillar
- doi10.1105/tpc.16.00768
- pmid28138016
-
- AHLiepman
- LJOlsen
- doi10.1104/pp.011460
- pmid12529529
-
- NLinka
- APWeber
- doi10.1093/mp/ssp108
- pmid20038549
-
- HLiu
- CPOsborne
- doi10.1093/jxb/eru430
- pmid25504656
-
- MRLundgren
- PAChristin
- doi10.1093/jxb/erw451
- pmid28025316
-
- JHMacduff
- AKBakken
- doi10.1093/jxb/erg058
- pmid12493871
-
- RMahadevan
- CHSchilling
- doi10.1016/j.ymben.2003.09.002
- pmid14642354
-
- JMallmann
- DHeckmann
- ABräutigam
- MJLercher
- APWeber
- PWesthoff
- UGowik
- doi10.7554/eLife.02478
- pmid24935935
-
- RKMonson
-
- LAMueller
- PZhang
- SYRhee
- doi10.1104/pp.102.017236
- pmid12805578
-
- HENeuhaus
- doi10.1016/j.febslet.2007.02.003
- pmid17307167
-
- WLOgren
- GBowes
- doi10.1038/newbio230159a0
- pmid5279476
-
- JDOrth
- IThiele
- BØPalsson
- doi10.1038/nbt.1614
- pmid20212490
-
- CPOsborne
- RPFreckleton
- doi10.1098/rspb.2008.1762
-
- CPál
- BPapp
- MJLercher
- PCsermely
- SGOliver
- LDHurst
- doi10.1038/nature04568
- pmid16572170
-
- BPapp
- BSzappanos
- RANotebaart
-
- JPetersen
- KFörster
- PTurina
- PGräber
- doi10.1073/pnas.1202799109
- pmid22733773
-
- TRPick
- ABräutigam
- USchlüter
- AKDenton
- CColmsee
- UScholz
- HFahnenstich
- RPieruschka
- URascher
- USonnewald
- APWeber
- doi10.1105/tpc.111.090324
- pmid22186372
-
- HPinto
- JRPowell
- RESharwood
- DTTissue
- OGhannoum
- doi10.1111/pce.12636
- pmid26381794
-
- JReiser
- NLinka
- LLemke
- WJeblick
- HENeuhaus
- doi10.1104/pp.104.049502
- pmid15516503
-
- JRozema
- doi10.2134/asaspecpub61.c1
-
- RFSage
- RWPearcy
- JRSeemann
- doi10.1104/pp.85.2.355
- pmid16665701
-
- RFSage
- doi10.1111/j.1469-8137.2004.00974.x
-
- RFSage
- TLSage
- FKocacinar
- doi10.1146/annurev-arplant-042811-105511
- pmid22404472
-
- RSaha
- PFSuthers
- CDMaranas
- doi10.1371/journal.pone.0021784
- pmid21755001
-
- AScheben
- FWolter
- JBatley
- HPuchta
- DEdwards
- doi10.1111/nph.14702
- pmid28762506
-
- USchlüter
- ABräutigam
- UGowik
- MMelzer
- PAChristin
- SKurz
- TMettler-Altmann
- APWeber
- doi10.1093/jxb/erw391
- pmid28110276
-
- USchlüter
- AKDenton
- ABräutigam
- doi10.1016/j.pbi.2016.03.007
- pmid27082280
-
- ISchomburg
- AChang
- OHofmann
- CEbeling
- FEhrentreich
- DSchomburg
- doi10.1016/S0968-0004(01)02027-8
- pmid11796225
-
- CJSchultz
- GMCoruzzi
- doi10.1046/j.1365-313X.1995.07010061.x
- pmid7894512
-
- TShikanai
- doi10.1007/s11120-016-0227-0
- pmid26858094
-
- MSimons
- AMisra
- GSriram
-
- BVSonawane
- RESharwood
- SWhitney
- OGhannoum
- doi10.1093/jxb/ery129
- pmid29659931
-
- RJSpreitzer
- MESalvucci
- doi10.1146/annurev.arplant.53.100301.135233
- pmid12221984
-
- MStitt
- SCZeeman
- doi10.1016/j.pbi.2012.03.016
- pmid22541711
-
- JSun
- TWOkita
- GEEdwards
- doi10.1104/pp.119.1.267
-
- DSwarbreck
- CWilks
- PLamesch
- TZBerardini
- MGarcia-Hernandez
- HFoerster
- DLi
- TMeyer
- RMuller
- LPloetz
- ARadenbaugh
- SSingh
- VSwing
- CTissier
- PZhang
- EHuala
- doi10.1093/nar/gkm965
- pmid17986450
-
- KThomas
- R-KBenjamin
- PFernando
- GBrian
- BMatthias
- FJonathan
- KKyle
- HJessica
- GJason
- CSylvain
-
- RTurgeon
- doi10.1146/annurev.pp.40.060189.001003
-
- PTurina
- JPetersen
- PGräber
- doi10.1016/j.bbabio.2016.02.019
-
- OUeno
- NSentoku
- doi10.1111/j.1365-3040.2005.01418.x
-
- AVishwakarma
- SDTetali
- JSelinski
- RScheibe
- KPadmasree
- doi10.1093/aob/mcv122
- pmid26292995
-
- EVVoznesenskaya
- NKKoteyeva
- SDXChuong
- ANIvanova
- JBarroca
- LACraven
- GEEdwards
- doi10.1071/FP06287
-
- YWang
- ABräutigam
- APWeber
- XGZhu
- doi10.1093/jxb/eru058
- pmid24609651
-
- SWeissmann
- FMa
- KFuruyama
- JGierse
- HBerg
- YShao
- MTaniguchi
- DKAllen
- TPBrutnell
- doi10.1105/tpc.15.00497
- pmid26813621
-
- TLWilkinson
- AEDouglas
- doi10.1046/j.1570-7458.2003.00014.x
-
- BPWilliams
- IGJohnston
- SCovshoff
- JMHibberd
- doi10.7554/eLife.00961
- pmid24082995
-
- AWingler
- RPWalker
- ZHChen
- RCLeegood
- doi10.1104/pp.120.2.539
- pmid10364405
-
- HYamamoto
- LPeng
- YFukao
- TShikanai
- doi10.1105/tpc.110.080291
- pmid21505067